Qubole vs Databricks: Key Features and Insights
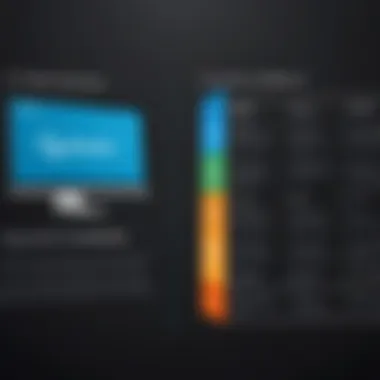
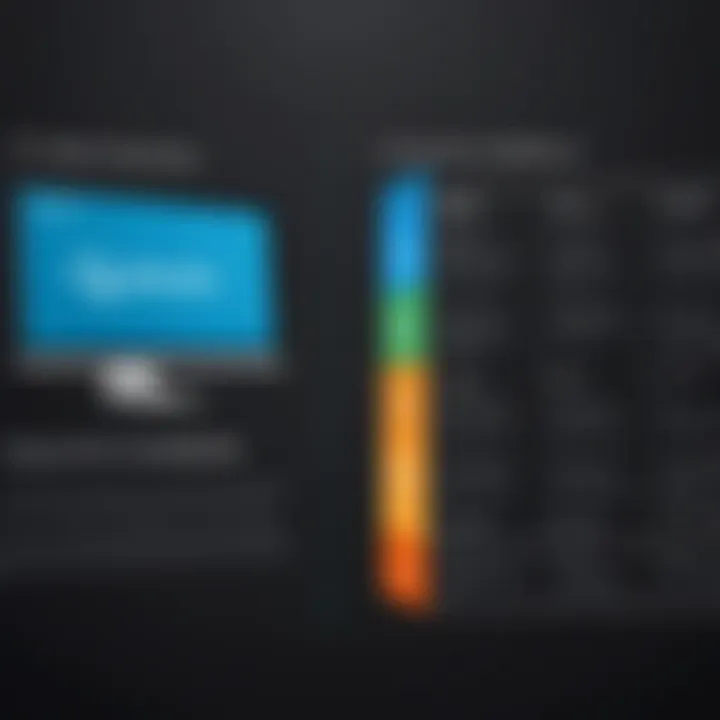
Intro
In the world of big data, two platforms stand out: Qubole and Databricks. Both offer capabilities designed to simplify data processing and improve performance. However, their approaches and features can differ significantly. Understanding these differences is key for organizations looking to optimize their data strategies. This analysis offers a thorough examination of both platforms, focusing on their essential features, pricing models, and unique advantages.
By comprehensively analyzing these platforms, businesses can better inform their purchasing decisions and align their data solutions with operational needs. The goal is to provide a clear overview that highlights the strengths and weaknesses of each platform, ultimately aiding decision-makers in the B2B landscape.
Key Features
Overview of Core Features
Qubole provides a robust platform that supports multiple engines such as Apache Spark, Presto, and Hive. This versatility allows users to choose the best tool for their specific workload. Databricks, built around Apache Spark, offers streamlined functionalities that enhance Spark's capabilities. Key features of both platforms include:
- Scalability: Both Qubole and Databricks offer scalable solutions suitable for varying data workloads.
- Data Processing Speed: Databricks emphasizes its optimization for fast data processing and speed.
- Support for Multiple Data Sources: Qubole allows connections to various data lakes, while Databricks integrates smoothly with data warehouses.
Each platform also provides unique capabilities unique to their architecture, impacting the overall user experience.
User Interface and Experience
User experience is crucial for any data platform. Qubole’s interface is characterized by its simplicity and intuitive navigation, making it accessible for users with varying technical expertise. Conversely, Databricks employs a more complex interface designed with data science teams in mind. This can lead to a steeper learning curve but offers powerful tools for experienced users. Factors to consider include:
- Learning Curve: Qubole tends to be more user-friendly, making it a good choice for teams with varying levels of expertise.
- Collaboration: Databricks promotes collaboration through shared notebooks and real-time editing features.
- Customization Options: Qubole allows for significant customization of the dashboard.
"Understanding how each platform facilitates user engagement is crucial in selecting the right one for your business needs."
Pricing and Plans
Overview of Pricing Models
Evaluating the cost structure of each platform is essential for organizations managing tight budgets. Qubole implements a consumption-based pricing model. This pricing is based on the resources utilized, such as compute power and storage.
In contrast, Databricks offers tiered pricing plans. These plans vary depending on the features and support levels needed. Users should consider:
- Consumption-Based Costs: Ideal for inconsistent workloads that do not require constant resources.
- Tiered Pricing Plans: Suitable for organizations looking for specific capabilities and predictable monthly billing.
Comparison of Different Plans
When comparing the plans offered by both platforms, organizations should take into account:
- Qubole's Flexible Pricing: Its multiple engines can lead to diverse pricing depending on the use case.
- Databricks's Premium Plans: These include enhanced support options and additional features, which can contribute to higher overall costs.
Understanding the pricing models will aid buyers in selecting a platform that not only meets their operational needs but also aligns with their budget constraints.
Foreword to Qubole and Databricks
Understanding the landscape of big data platforms is crucial for organizations looking to leverage their data. Qubole and Databricks are two prominent players in this field, each bringing unique strengths and capabilities to the table. This section primarily highlights the importance of these platforms and sets the foundation for a deeper exploration of their features and functionalities.
Organizations today require agile data solutions that can scale efficiently, provide advanced analytics capabilities, and integrate with various data sources. This need emphasizes the significance of platforms like Qubole and Databricks, as they offer tailored tools for data processing and analysis. Their importance in business operations cannot be overstated, as choosing the right platform can significantly impact operational efficiency and decision-making.
In this section, we will provide an overview of both Qubole and Databricks. This will give readers an understanding of where each platform excels, what innovative features they offer, and the implications these have for businesses aiming to utilize big data effectively.
Overview of Qubole
Qubole is a cloud-based data platform designed to help users manage and analyze vast datasets effortlessly. It is engineered to work seamlessly with multiple cloud services, including Amazon Web Services, Google Cloud Platform, and Microsoft Azure. One of the standout features of Qubole is its user-friendly interface, which allows data scientists and analysts to interact with data without requiring extensive technical knowledge.
This platform supports various data processing engines, such as Apache Spark, Apache Hive, and Presto. By offering multi-engine support, Qubole provides flexibility in choosing the right tool for specific tasks. This helps users optimize their workflows based on the operational requirements of their projects. Furthermore, Qubole’s auto-scaling capability allows businesses to scale their resources dynamically based on demand, which can lead to cost savings and performance improvements.
Qubole is particularly noted for its robust data governance and security features. Organizations can manage user permissions and maintain data integrity while ensuring compliance with regulations. Its ability to integrate with popular BI tools further enhances its appeal, making it an ideal choice for data visualization and reporting tasks.
Overview of Databricks
Databricks, co-founded by the creators of Apache Spark, stands out for its unified analytics platform that integrates data engineering and data science. It offers an environment that streamlines the processes of data preparation, machine learning, and analytics. One of Databricks’ specific strengths is its collaborative workspace, which fosters teamwork among data engineers and scientists, enabling them to work on shared notebooks in real time.
The platform is designed to simplify data workflows, enabling organizations to harness data lakes and turn them into valuable insights. Databricks supports a range of programming languages, including Python, SQL, and R, allowing users to choose their preferred languages for data manipulation and analysis.
Moreover, Databricks provides robust machine learning capabilities, making it easier for teams to deploy models into production efficiently. With features such as automated cluster management, flexible scaling, and end-to-end workflows, it stands out as a powerful tool for advanced analytics. Security in Databricks is also fortified, with fine-grained access controls and compliance features tailored for enterprise needs. This aspect makes it suitable for organizations that handle sensitive data and require stringent security protocols.
"The choice between Qubole and Databricks hinges on organizational requirements for data processing and analytics capabilities. Both platforms deliver unique benefits suited for different use cases."
With this understanding of Qubole and Databricks, we now shift towards a more focused comparison of key features that define their operational efficiencies.
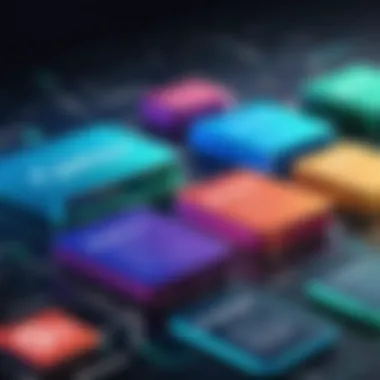
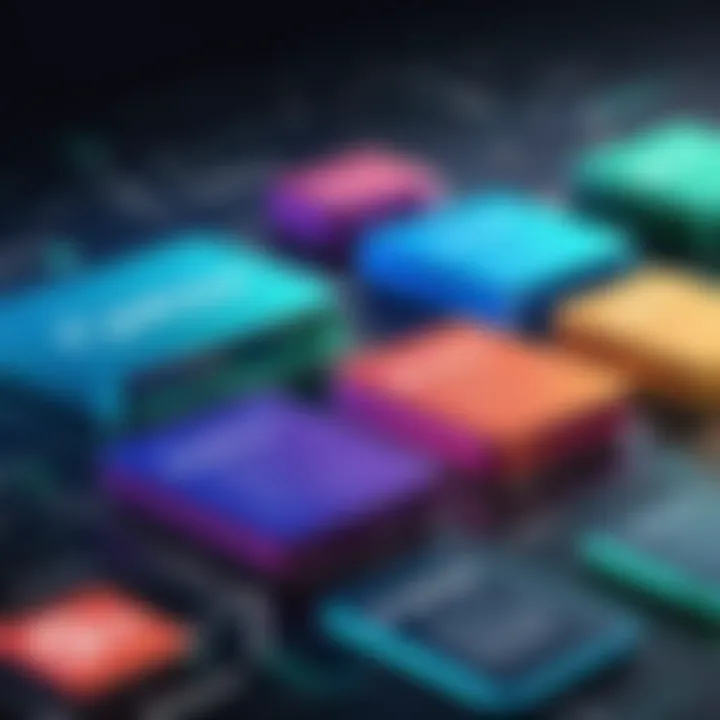
Key Features Comparison
A detailed comparison of key features between Qubole and Databricks is essential for understanding which platform may best serve a business's needs. Each platform has unique offerings that cater to different challenges in big data processing and analytics. Evaluating these features helps stakeholders to identify specific capabilities that align with their operational requirements and strategic goals.
Data Processing Capabilities
Data processing is the core function of any big data platform. Qubole excels in providing a range of data processing options. It supports multiple processing engines like Apache Spark, Hive, Presto, and others, creating a flexible environment for users. Users can select the best engine depending on their data workload, which optimizes efficiency.
In contrast, Databricks is tailored for Apache Spark, providing unique optimizations and improved performance. These smart features reduce the complexity of setting up Spark and eliminate many of the headaches that come with its management. Databricks also incorporates its Delta Lake, which allows for better data management practices, ensuring ACID transactions and improved consistency across data lakes.
Choosing between these two platforms often comes down to specific data processing needs. If a company requires versatility, Qubole’s multi-engine approach could be preferable. For those heavily invested in Spark, Databricks' targeted optimizations present a strong case.
Integration with Other Tools
Integration capabilities are pivotal in the decision-making process regarding big data platforms. Qubole provides robust integration with various tools like AWS, Google Cloud, and Microsoft Azure. This flexibility allows businesses to leverage existing systems and data sources without significant reconfiguration.
On the other hand, Databricks offers seamless integration with tools catering to data science and machine learning, such as MLflow and TensorFlow. This aspect can be particularly appealing for organizations focusing on advanced analytics and machine learning projects. The collaborative features of Databricks, including shared workspaces, also enhance productivity among data teams.
Ultimately, the choice of platform could depend on the existing toolchains within an organization. A company utilizing a diverse ecosystem of tools might find Qubole's integration capabilities more beneficial. Conversely, a data-driven organization invested in machine learning may lean towards Databricks due to its specialized integrations.
User Interface and Experience
User interface plays a critical role in the effectiveness of any data platform. Qubole emphasizes simplicity with its intuitive dashboard, which aids users in navigating their data tasks efficiently. The user experience is designed to accommodate both technical users and business analysts, which could be a strong selling point for organizations with diverse team capabilities.
Databricks, while offering a slightly steeper learning curve, provides advanced features that power users might prefer. Its notebooks enable users to combine code and documentation seamlessly, fostering collaboration among data teams. This direct connection between code, its results, and corresponding narratives can significantly enhance the data interpretation process.
In this regard, organizations may need to consider the skill set of their teams. If an organization has less technical personnel, Qubole's user interface may be more suitable. If the focus is on fostering advanced analytics through collaboratively-written code, Databricks presents a compelling option.
Performance Metrics
Performance metrics are crucial when evaluating data processing platforms such as Qubole and Databricks. They provide insight into how well each platform handles large datasets, scales under various loads, and maintains efficiency in data management. Understanding these metrics not only helps organizations choose the right solution but also ensures that the selected platform aligns well with their operational requirements. In this section, we will focus on two main areas: speed and scalability, as well as data management efficiency.
Speed and Scalability
Speed is often a determining factor for organizations that rely on big data analytics. Both Qubole and Databricks utilize powerful underlying technologies to process data quickly. Qubole offers a variety of processing engines, including Apache Spark and Presto, allowing users to select the best engine depending on their specific use case.
On the other hand, Databricks emphasizes its optimized version of Spark, which enhances performance through various features. These optimizations can lead to noticeably faster query times and job execution. The scalability of both platforms is also impressive, enabling them to handle increasing data volumes and user loads effectively.
When considering speed and scalability, it's essential to assess the following:
- Query performance: How quickly does the platform return results for large-scale queries?
- Resource allocation: Does the platform efficiently utilize resources to optimize performance?
- Concurrent users: How well does the platform support multiple users working simultaneously?
Each of these aspects affects the overall performance of data operations, contributing to faster insights and more agile decision-making for businesses.
Data Management Efficiency
Data management efficiency is another vital performance metric. It encapsulates how well a platform manages data storage, retrieval, and processing tasks. Here, both Qubole and Databricks provide unique features that cater to different data management needs.
In Qubole, automatic scaling and workload management are notable features. This allows users to scale resources based on real-time demands, ensuring data operations are efficient without excessive costs. Additionally, Qubole’s support for various data sources and formats simplifies the integration and migration processes.
Databricks, meanwhile, integrates seamlessly with its Delta Lake, which enhances the ability to manage data efficiently at scale. Delta Lake’s time travel capabilities allow users to query historical data versions, facilitating easy data audit and recovery processes. This also means that data can be managed in a more organized manner, reducing redundancy and ensuring integrity.
Important factors to consider include:
- Data integration: How easily can data from different sources be integrated?
- Data governance: Are there strong data management practices in place to ensure data quality and compliance?
- Cost efficiency: Does the platform optimize costs while managing data effectively?
Pricing Models
Understanding the pricing models of Qubole and Databricks is crucial for any business looking to invest in big data solutions. The selected pricing structure can have a profound impact on the long-term costs, operational efficiency, and overall return on investment. Choosing the right model goes beyond the base cost; it also encompasses reward mechanisms tied to usage, scalability, and custom flexibility.
Pricing models typically include various pricing factors such as compute resources, storage options, and the features included at each tier. Assessing these elements helps businesses evaluate which platform aligns best with their budget and operational needs.
In addition to upfront costs, it’s essential to consider the following:
- Usage-Based Pricing: Pay only for what you use, which can be more economical for businesses with variable workloads.
- Subscription Models: Fixed monthly or yearly fees providing predictable expenses.
- Discount Structures: Discounts for long-term commitments or high-volume usage, which can shift the cost dynamics favorably.
"Understanding the pricing model is like fitting the last piece into a puzzle. It completes the overall picture of the solution you are considering."
Thus, businesses must evaluate these models to establish a comprehensive view of potential investment returns while ensuring that they do not compromise future scalability or operational flexibility.
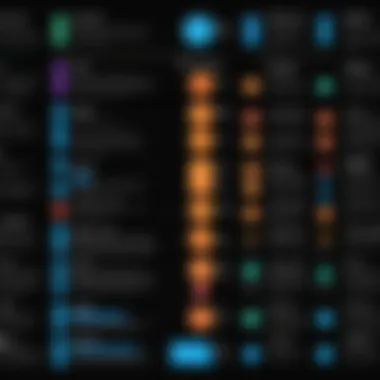
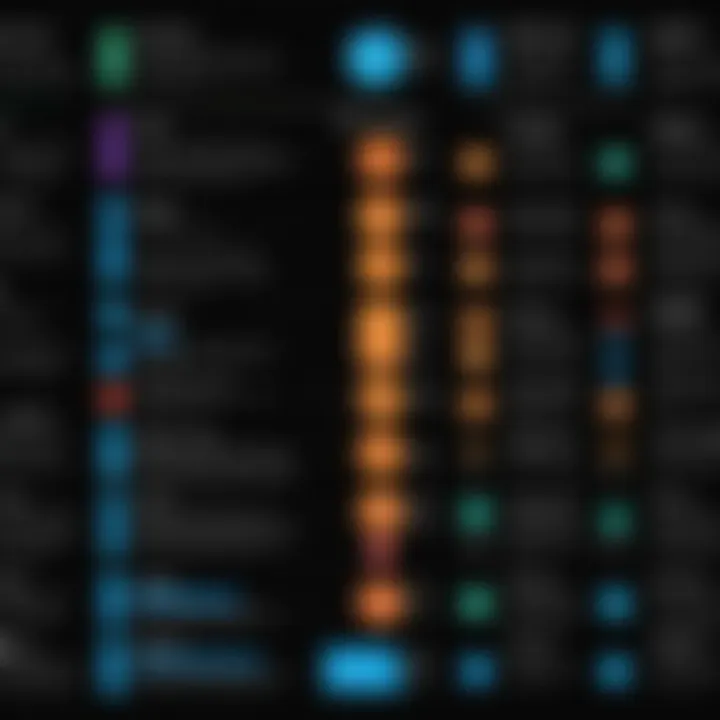
Qubole Pricing Structure
Qubole employs a flexible pricing structure that allows customers to tailor their spending to their unique data processing needs. Essentially, it combines usage-based and reserved pricing. Users can reduce costs by selecting a monthly reserved instance, which guarantees lower pricing for a committed period.
Specific elements of Qubole's pricing structure include:
- Compute Charges: Based on the resources used, charged per hour or minute, which enables companies to scale their usage as needed.
- Storage Costs: Monthly charges for data storage, which apply to both managed and external storage.
- Add-On Features: Certain features, such as advanced security and data governance tools, may incur additional costs, further customizing the user experience.
This structure makes it easier for organizations to forecast expenses accurately while maintaining the flexibility to adjust based on changing data needs.
Databricks Pricing Structure
Databricks also utilizes a consumption-based model with options for monthly subscription pricing. Its pricing is segmented into tiers based on the number of users and functionalities required. This tiered structure allows businesses to select a package that suits their specific requirements.
Key aspects of Databricks' pricing model include:
- Databricks Units (DBUs): A critical unit of measure for pricing, they are billed based on the processing power and time utilized for workloads, providing transparency in billing.
- Workspace Charges: Monthly fees for using Databricks workspaces that contain notebooks and collaborative tools.
- Volume Discounts: Businesses that commit to higher usage levels may benefit from discounts, further enhancing cost-effectiveness.
Overall, the pricing structure of Databricks is designed to align closely with usage patterns, allowing organizations to only pay for the resources that fit their needs.
Use Cases
Understanding the use cases for Qubole and Databricks plays a vital role in determining which platform aligns best with specific organizational needs. Each platform offers unique features that cater to distinct industries and scenarios. By examining these use cases, businesses can uncover the practical benefits and considerations when integrating either Qubole or Databricks into their operations. This insight not only enhances strategic planning but also ensures that decision-makers can deploy the right solution to maximize efficiency and effectiveness.
Industries Leveraging Qubole
Qubole is favored by organizations looking to harness the power of big data for various applications. Particularly, industries that prioritize data analytics, artificial intelligence, and machine learning benefit greatly from Qubole’s flexibility and cloud-native architecture. For example, companies in the e-commerce sector use Qubole for real-time analytics to track customer behavior and optimize inventory management.
In addition, the telecommunications industry employs Qubole for processing large volumes of data generated by mobile devices. The ability to scale resources dynamically helps these organizations adapt quickly to changing demands while maintaining performance. Furthermore, the finance and banking sector values Qubole’s robust data processing capabilities for risk analysis and fraud detection.
Industries Leveraging Databricks
Databricks shines in environments focused on collaborative data science and data engineering. Its seamless integration with Apache Spark makes it a go-to platform for companies in the tech and software development sectors that require efficient data processing and machine learning capabilities. Organizations in the healthcare industry also leverage Databricks to analyze patient data, streamline operations, and enhance care delivery.
Moreover, businesses in the media and entertainment fields utilize Databricks for handling massive datasets, enabling them to gain insights into content preferences and viewer engagement. The comprehensive tools available within Databricks foster innovation, thus proving attractive to industries that prioritize data-driven decision-making.
By examining the industries leveraging both Qubole and Databricks, it becomes clear that the choice between the two can significantly impact datahandling capabilities and overall business outcomes.
Strengths and Weaknesses Assessment
Assessing the strengths and weaknesses of Qubole and Databricks is vital for any organization evaluating these platforms. Understanding what each solution excels at can help businesses leverage their technological capabilities effectively. Conversely, recognizing weaknesses allows decision-makers to weigh potential risks and limitations against their operational objectives. This assessment guides users in identifying which platform best meets their data processing and analysis needs, ultimately leading to informed choices and optimized resource allocation.
Strengths of Qubole
Qubole presents several strengths that make it a compelling choice for businesses. Here are some notable advantages:
- Multi-Cloud Flexibility: Qubole operates seamlessly across different cloud platforms. This allows businesses to choose their preferred infrastructure without being locked into a single provider.
- Automated Scaling: With its robust auto-scaling capabilities, Qubole manages compute resources efficiently. This leads to optimal performance during workload spikes without manual adjustments.
- Unified Data Management: The platform provides integrated data management tools that enable users to access, process, and analyze data from various sources in one place. This reduces silos and promotes better data governance.
- Support for Multiple Languages: Qubole allows data processing using different programming languages like Python, SQL, R, and more. This flexibility caters to diverse team skill sets.
- Advanced Analytics: The built-in machine learning tools facilitate complex analytics tasks. Businesses can gain valuable insights without needing additional third-party tools.
Weaknesses of Qubole
While Qubole offers impressive features, it also has certain weaknesses. These should be considered:
- Steeper Learning Curve: Users may experience challenges in fully utilizing the platform’s features due to its complexity. Training may be required for effective use.
- Cost Considerations: Depending on the workloads and cloud usage, costs can escalate. Organizations should carefully assess pricing models to avoid unexpected expenses.
- Limited Community Support: Compared to some competitors, the Qubole user community is smaller. This could hinder quickly finding solutions to specific issues or challenges.
- Performance Fluctuations: During peak times, users may notice latency issues with data loading and processing. This can impact time-sensitive decision-making.
Strengths of Databricks
Databricks also offers unique strengths that cater to different needs:
- Collaborative Workspace: The platform provides interactive notebooks that promote collaboration among data scientists and analysts. This drives innovation and streamlined workflows.
- Apache Spark Integration: Databricks leverages Apache Spark, resulting in high-performance data processing and analysis capabilities. It is particularly suited for big data workloads.
- Machine Learning and AI Support: Users have access to advanced ML tools and libraries. This enables the development of sophisticated algorithms and predictive models.
- Real-Time Analytics: Databricks supports real-time analytics, allowing organizations to derive insights from data as it is generated. This is vital for industries requiring quick decision-making.
- Comprehensive Documentation: The platform has extensive documentation and resources available, which can ease the onboarding process for new users.
Weaknesses of Databricks
Despite its advantages, Databricks presents certain challenges:
- Costly for Small Businesses: The pricing structure can be prohibitive for smaller organizations. Cost considerations are essential in evaluating the overall value proposition.
- Complexity with Setup: Initial setup and configuration can require significant technical expertise. This might necessitate hiring specialized personnel for proper implementation.
- Dependence on Cloud Environment: Being primarily cloud-based, organizations with strict on-premises data requirements may find Databricks less suitable.
- Steep Learning Curve: New users may find it challenging to adapt to the platform's full range of functionalities due to its sophisticated features.
Security and Compliance
In the realm of big data, the significance of security and compliance cannot be overstated. Organizations increasingly rely on data-driven decisions, which necessitates stringent measures to protect sensitive information. When comparing Qubole and Databricks, it is crucial to evaluate how each platform addresses security and compliance. A robust security framework not only safeguards data from breaches but also ensures compliance with regulatory standards. This is vital in maintaining customer trust and reputation in the marketplace. The focus on security should encompass data encryption, user access management, and audit trails. These elements together help in mitigating risks associated with data handling and processing. Moreover, understanding the compliance posture of each platform aids organizations in selecting solutions that align with industry regulations.
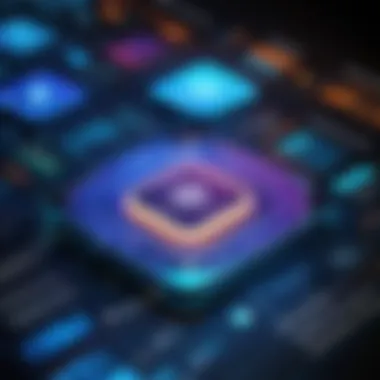
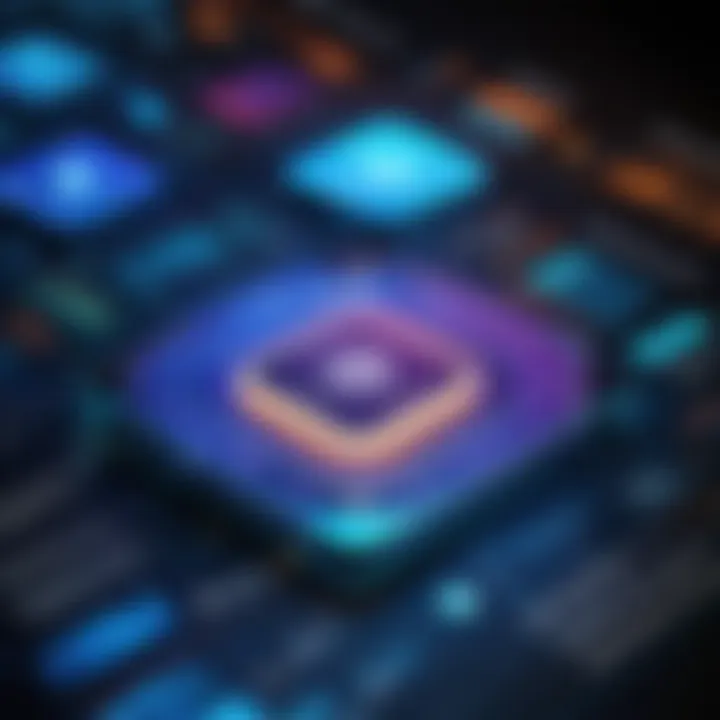
Data Security in Qubole
Qubole places a strong emphasis on data security through a multifaceted approach. It supports end-to-end encryption, ensuring that data is protected both in transit and at rest. This means sensitive information remains secure whether it is being sent over the internet or when it is stored on disk. Additionally, Qubole utilizes role-based access controls, allowing organizations to define who can access specific datasets. This feature helps to minimize the risk of unauthorized access.
Another security facet is the integration of third-party identity providers. Qubole supports services like Okta and Azure Active Directory, which bolster user authentication measures. Audit logs are also a key component in Qubole, providing organizations with a trail of user activity. These logs are essential for compliance audits and can help organizations identify and respond to potential security incidents efficiently.
Data Security in Databricks
Databricks similarly prioritizes data security through comprehensive measures. It employs encryption for data in transit and at rest, reflecting best practices in data protection. This ensures that any data flowing between the user's desktop and the Databricks environment is safeguarded from potential interception.
User access control in Databricks is also meticulous. It includes support for single sign-on (SSO) through various providers, ensuring that user identities are managed securely. The platform provides fine-grained access controls that allow data teams to set permissions at various levels, from the workspace down to the table or row level.
Furthermore, Databricks offers detailed logging capabilities, which are instrumental for monitoring data access and modifications. This not only facilitates security auditing but also assists in compliance with frameworks such as GDPR and HIPAA. Having these logs helps organizations maintain accountability and transparency regarding their data practices.
Customer Support and Community Engagement
Customer support and community engagement play critical roles in the overall experience of users on data platforms like Qubole and Databricks. This section examines how both companies approach these elements, which are vital for ensuring user satisfaction and successful implementation of their services. A responsive support team can make a significant difference, especially in a domain where complex data tasks may arise. Furthermore, community engagement fosters a collaborative environment where users can share knowledge and solutions.
Qubole Support Services
Qubole offers multiple support options to cater to their users' diverse needs. The support services include a combination of live chat, email, and a detailed knowledge base. Each of these methods serves a particular purpose. For immediate assistance, the live chat feature is particularly beneficial. It allows users to get real-time help for urgent issues. Email support, on the other hand, can be used for more comprehensive inquiries that require detailed feedback.
In addition to direct support, Qubole's customer support includes proactive monitoring services. This means that Qubole keeps an eye on the system's performance and may provide alerts regarding potential issues before they escalate. The aim is to maintain seamless performance and to reduce downtime. It is also essential to mention that Qubole's support team is made up of professionals who are well-versed in data engineering and analytics. This expertise allows for high-quality responses and effective troubleshooting.
Furthermore, users can utilize Qubole's community forums. These forums are a goldmine for users to exchange ideas, ask questions, and even find unofficial solutions from fellow users. User input often leads to product improvements, making community engagement another pillar of Qubole's user support strategy.
Databricks Support Services
Databricks also prioritizes customer support, offering several avenues for users to access help. They provide a centralized support portal, which includes a ticketing system for tracking support requests. This portal is designed for efficiency, allowing users to see the status of their inquiries and manage them seamlessly. Additionally, Databricks offers phone support during business hours, which can be crucial for organizations that operate on tight schedules.
Moreover, the knowledge base provided by Databricks is extensive. It includes documentation, tutorials, and best practices designed for both new and experienced users. This resource is particularly beneficial for teams that prefer self-service solutions.
Community engagement is another area where Databricks excels. The company encourages users to participate in forums and discussion groups. This not only creates a space for knowledge-sharing but also helps users connect with industry peers. The active community provides a platform for exchanging innovative use cases, which can serve as inspiration for users looking to maximize their use of Databricks.
"A responsive support system is crucial for the successful adoption of data platforms. It directly affects the productivity and satisfaction of users."
Future of Qubole and Databricks
As the big data landscape continues to evolve, understanding the future trajectories of Qubole and Databricks becomes crucial for organizations aiming to make informed technological investments. This section will explore anticipated trends and developments for both platforms, shedding light on how they might adapt to changing market demands, emerging technologies, and user needs.
The evolution of Qubole and Databricks is not just about new features; it involves how they position themselves in a competitive environment. Key elements include enhancing data processing capabilities, improving integration with other tools, and reflecting on user experiences. Companies rely on these platforms to remain efficient and competitive in the dynamic data-driven world.
Predicted Trends for Qubole
Qubole's future appears to be geared towards expanding its pool of integrations. As companies increasingly leverage a mixed-tech ecosystem, Broadening seamless connections with tools like Snowflake, AWS, and Google Cloud could enhance usability. Furthermore, Qubole may amplify its focus on automated workflows, aimed at reducing complexity for users.
Another important trend for Qubole is the emphasis on advanced analytics. Machine learning and artificial intelligence are becoming essential for many businesses. Qubole might develop more tools supporting these technologies, allowing users to derive deeper insights from their data.
As organizations continue to prioritize security, Qubole is likely to enhance its security frameworks to comply with stringent regulations. This adaptability will allow it to cater to industries that face complex data compliance challenges. In summary, Qubole's future trends will likely include:
- Enhanced integrations with popular platforms
- Focus on automated analytics workflows
- Advanced AI and machine learning capabilities
- Improved security measures for compliance
Predicted Trends for Databricks
Databricks is expected to continue its momentum in the market by consolidating its leadership in collaborative data science. The company has a strong foothold in the Apache Spark community, and it's predicted that they will further enhance collaborative tools for data scientists and engineers. This could include better interfaces or features for sharing and visualizing data, making the entire process more interactive.
The rise of lakehouse architecture is another area where Databricks will likely focus. As businesses seek to unify data lakes and data warehouses, Databricks may evolve its platform to provide more streamlined lakehouse solutions, catering to scalability and performance.
Security and compliance will remain a priority for Databricks, especially as organizations grapple with data privacy laws worldwide. Investing in these areas would serve as a crucial element to retain trust and build client relationships. In summary, important trends for Databricks might include:
- Advanced collaboration tools for data science teams
- Enhancements for lakehouse architecture that improve performance
- Increased investment in security and compliance frameworks
As companies continue to innovate and adapt to the changing data environment, the capacity of Qubole and Databricks to remain relevant will largely depend on their agility in adopting new technologies and meeting evolving client expectations.
The End and Recommendation
The conclusion and recommendation section serves as a crucial component of this article as it synthesizes the information presented in earlier sections while guiding decision-makers in their choice between Qubole and Databricks. This is particularly important due to the significant implications that such decisions have on business operations, data management strategies, and overall efficiency.
Final Thoughts on Qubole versus Databricks
Both Qubole and Databricks offer impressive capabilities for managing big data, but they cater to slightly different needs and approaches. Qubole shines in its flexibility and integration capabilities, allowing users to process large volumes of data efficiently. It is particularly effective for businesses that require a strong emphasis on self-service analytics and various tool integrations. On the other hand, Databricks excels in its ability to provide a collaborative environment for data scientists and analysts. Its integration with Apache Spark facilitates advanced analytical processes, making it ideal for organizations focused on real-time data processing and machine learning workflows.
Ultimately, the choice depends on specific organizational requirements. Companies needing robust data processing may favor Qubole, while those interested in machine learning and real-time analytics may find Databricks more appealing.
Choosing the Right Solution for Your Business
When selecting between Qubole and Databricks, several considerations should guide decision-making:
- Business Goals: Evaluate how each platform aligns with your organization's goals. Assess whether your primary focus is on data processing, analytics, or collaboration.
- Budget Constraints: Understand the pricing models discussed. Consider long-term costs against the backdrop of expected return on investment.
- Technical Expertise: Review the skillset of your team. If your team is more familiar with Spark, Databricks might be easier to adopt. Conversely, if they prefer a variety of tools, Qubole could be the better fit.
- Scalability Needs: Consider how each solution can scale with your business growth. You need a platform that will evolve alongside your data requirements.