Q Data Analysis: Comprehensive Insights for B2B Software
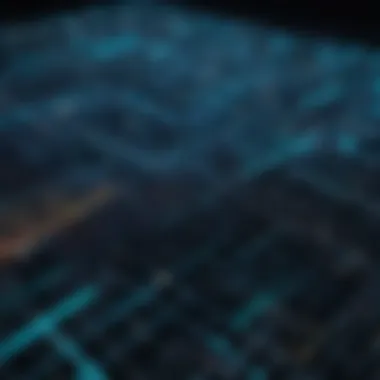
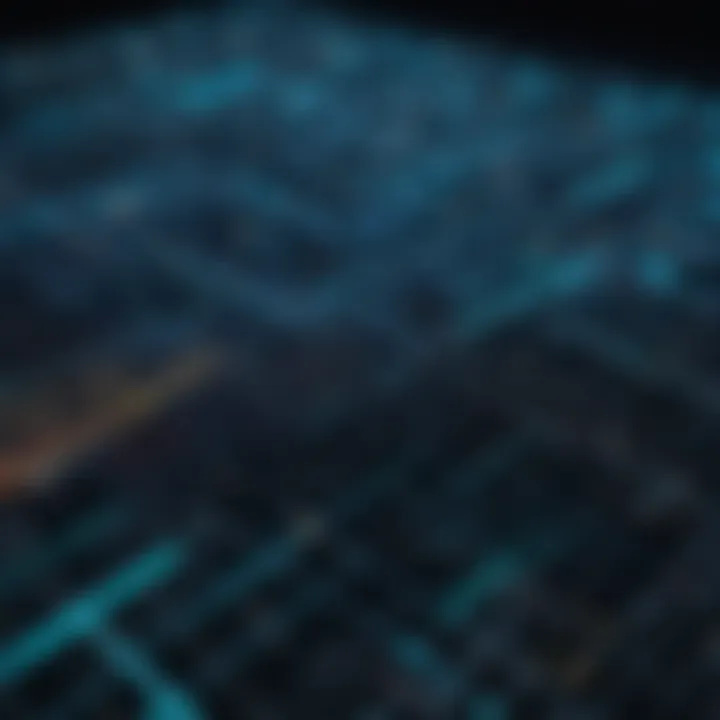
Intro
In the contemporary business landscape, data has become a currency of its own. Particularly within the sphere of B2B software solutions, Q data analysis has emerged as a pivotal tool for organizations aiming to utilize data strategically. This methodology provides a framework for transforming raw data into insightful information that can steer decision-making processes. Understanding the relevance and approach to Q data analysis is essential for those involved in the procurement and implementation of technology solutions.
The essence of Q data analysis revolves around its methodologies and applications. It encompasses various techniques that facilitate a thorough examination of data sets, yielding actionable insights. B2B organizations that harness these insights can often gain a competitive edge, improving operational efficiency and fostering better customer relationships.
This article aims to unravel the complexities surrounding Q data analysis. We will explore its core features, pricing models, and practical applications that cater to decision-makers, procurement officers, and IT professionals. In doing so, we will equip our audience with the necessary knowledge to navigate the intricacies of data analysis within enterprise technology.
Data-driven decision-making is no longer just a trend; it is a vital practice for successful businesses. In the parts to come, we will outline the key aspects that define Q data analysis and examine how integrating advanced analytical tools can fundamentally transform organizational strategies.
Understanding Q Data Analysis
Q Data Analysis serves a pivotal role in modern business practices, especially within the context of B2B software solutions. Understanding this form of analysis is vital for organizations aiming to leverage their data effectively. It allows decision-makers to extract valuable insights that inform strategy and drive enterprise growth.
Definition and Scope
Q Data Analysis can be defined as a systematic approach to examining data to draw meaningful conclusions and make data-driven decisions. The scope of Q Data Analysis goes beyond simple data collection; it encapsulates the methods used in cleaning, processing, and interpreting data to uncover trends and patterns that can influence business outcomes. This process is essential for organizations that manage large volumes of data and need clarity in interpreting those data sets.
Its importance is underscored by the increasing reliance on data in all sectors; therefore, understanding Q Data Analysis allows businesses to harness insights from their data effectively. Benefits of a solid grasp of this area include improved decision-making, optimized operations, and enhanced customer experiences.
Historical Context
Historically, data analysis has evolved significantly, primarily driven by advancements in technology. In the early days, data analysis was often conducted manually, with analysts relying on basic statistical techniques to draw insights. As businesses began to recognize the value of data, more sophisticated methods emerged. The advent of computers in the 1960s accelerated this evolution, enabling more complex data handling and analysis.
In recent decades, the rise of big data and increased computational power has transformed Q Data Analysis. The introduction of machine learning algorithms and data visualization tools has further refined the analysis process. Now, businesses can analyze vast datasets in real-time, making it easier to derive actionable insights quickly. Understanding this historical progression not only highlights the importance of Q Data Analysis today but also sheds light on future trends in the industry.
"In the evolving landscape of data, the ability to analyze and interpret insights is no longer a luxury, but a necessity for survival."
Through understanding its definition, scope, and historical context, professionals can better appreciate the complexities of Q Data Analysis and its crucial role in strategic business decisions.
Core Principles of Data Analysis
Core principles of data analysis serve as the foundation for understanding and interpreting data. These principles dictate how data is collected, processed, and analyzed, ensuring that the outcomes are reliable and applicable. In the context of Q data analysis, adhering to these principles is crucial for extracting meaningful insights, which in turn drives strategic decision-making. The benefits of these principles include enhanced accuracy, increased efficiency, and better clarity in results. It is critical for organizations to recognize these elements to harness the full potential of their data analysis practices.
Data Collection Techniques
Data collection is a key area within data analysis that influences the quality of insights extracted. The two main types of data collection techniques are qualitative and quantitative data collection.
Qualitative vs Quantitative Data
When discussing Qualitative vs Quantitative data, the most significant aspect that comes into play is the nature of the information being gathered. Qualitative data is primarily descriptive, encompassing insights gathered from interviews or open-ended survey responses. This approach helps in understanding emotions, motivations, and reasons behind certain behaviors. Conversely, quantitative data focuses on numerical values and statistics, making it easier to analyze with mathematical models.
Key characteristic: The primary advantage of qualitative data is its depth in exploring complexities. This makes it a vital choice for contexts where exploring human behavior is important. On the other hand, quantitative data benefits from being easily measurable and comparably analyzed, which is crucial for predictive analytics and tracking performance over time.
The unique feature of qualitative data is its subjective nature, which can provide nuanced understandings but can also lead to biases. Quantitative data, on the contrary, can deliver objective facts but may overlook deeper insights. Thus, employing a mix of both has become a beneficial strategy in many analytical frameworks, including Q data analysis.
Primary and Secondary Data
The discussion of Primary and Secondary data is also essential to understand the varying sources and their impact on analysis outcomes. Primary data refers to original data collected by the researcher for specific purposes at hand. This is often gathered through surveys, experiments, or direct measurements. In contrast, secondary data involves the analysis of existing data that was collected by others, such as reports, databases, or literature.
Key characteristic: Primary data allows for tailored collection that directly targets the questions needing answers, which, therefore, makes it a popular choice for researchers looking for specific insights. However, it can be time- and resource-consuming to gather. Meanwhile, secondary data is abundant and accessible, making it an attractive option for analysts, but it might not align perfectly with current requirements or contexts of study.
The uniqueness of primary data lies in its specificity and context relevance, while secondary data is characterized by its ease and breadth. Both types of data have distinct advantages and disadvantages that can impact the course of the analysis performed in Q data analysis.
Data Processing and Cleaning
Data processing and cleaning are crucial steps in ensuring that data is accurate and usable. In this phase, raw data collected undergoes transformation, which includes checking for errors, removing duplicates, and filling in missing values. The importance of this step cannot be overstressed. If the data is flawed at this level, the subsequent analysis will likely lead to misleading conclusions. Efficient data cleaning not only enhances the quality of insights but also optimizes the efficacy of analytical models used down the line. Decisions based on poor data can cost organizations significantly. Thus, investing time in proper data processing and cleaning establishes a reliable foundation for meaningful analytics in Q data analysis.
Analytical Techniques in Q Data Analysis
Analytical techniques play a critical role in Q data analysis. They facilitate a structured approach to understanding and interpreting data. Efficient use of these techniques leads to better insights, informed decision-making, and improved outcomes across various business operations. Knowledge of these analytical techniques enables professionals to extract value from raw data, deriving actionable strategies tailored to specific business needs.
Descriptive Analytics
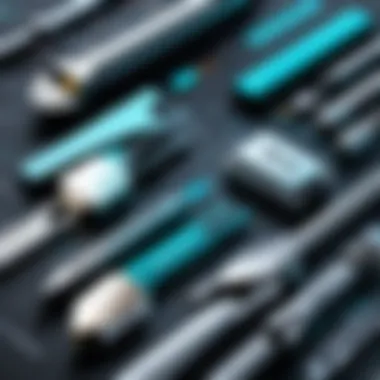
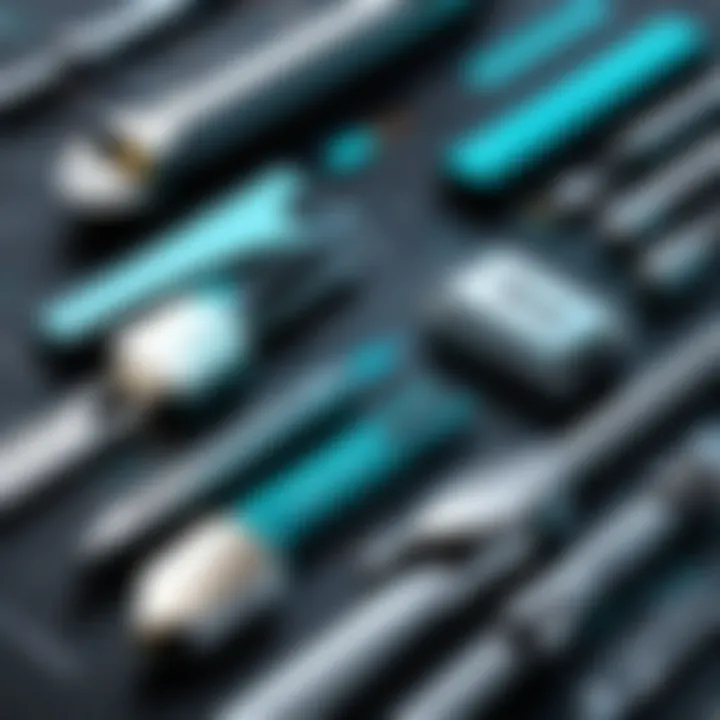
Descriptive analytics serves as the foundation for understanding historical data. This technique focuses on summarizing data from the past to identify trends and patterns. Through methods like statistical analysis and data visualization, descriptive analytics provides a clear picture of what has already happened.
Key aspects include:
- Data Summarization: Descriptive analytics helps condense vast amounts of data into understandable formats, such as dashboards and charts. This in turn enables quick assessments of performance.
- Identification of Trends: By analyzing past behaviors, businesses can recognize patterns that inform future strategies.
- Performance Measurement: Organizations can measure their performance against benchmarks to evaluate success over time.
Overall, descriptive analytics is essential for generating insights that are immediately actionable.
Predictive Analytics
Predictive analytics takes a forward-looking approach. It uses past data and statistical algorithms to predict future outcomes. This technique leverages data mining, machine learning, and historical data to forecast likely events.
Consider the following elements:
- Risk Assessment: By understanding potential future risks, businesses can create strategies to mitigate these challenges.
- Market Trends Forecasting: Predictive models can assist organizations in identifying upcoming trends, allowing them to stay ahead in the competitive landscape.
- Customer Behavior Prediction: Businesses can tailor their marketing efforts based on anticipated customer behavior, which in turn increases conversion rates.
This analytical technique is valuable for strategic planning, helping organizations make informed decisions based on likely future scenarios.
Prescriptive Analytics
Prescriptive analytics goes beyond prediction. It provides recommendations for how to handle future situations. This technique uses optimization and simulation algorithms to suggest courses of action based on a set of potential scenarios.
Important factors include:
- Decision Support: By presenting multiple options and their potential outcomes, prescriptive analytics empowers decision-makers to choose strategies with the best possible results.
- Resource Optimization: Organizations can efficiently allocate resources based on recommendations, enhancing overall operational efficiency.
- Scenario Testing: This technique allows businesses to simulate various scenarios and analyze possible outcomes before making commitments.
In summary, prescriptive analytics is vital for developing strategic plans and executing them effectively.
Together, these analytical techniques help organizations harness the power of their data, transforming raw inputs into informed business strategies.
Tools and Technologies for Q Data Analysis
Tools and technologies play a crucial role in Q data analysis. They streamline the processes of data collection, processing, and analysis. Understanding these tools allows stakeholders to make informed decisions based on data-driven insights. Each tool offers unique features that cater to specific analytical needs. Consequently, choosing the right tool can enhance productivity and accuracy in analyses. This section examines the overview of analytical platforms, the ongoing debate between open source and proprietary software, along with their respective advantages and limitations.
Overview of Analytical Platforms
Analytical platforms are software environments that support data analysis. These platforms help in organizing data, running complex queries, and visualizing results. They vary widely in functionality and complexity. Platforms like Tableau and Microsoft Power BI focus on visual analytics and dashboarding. Other, more heavy-duty options include Apache Hadoop, which is designed for big data processing. Each platform offers specific tools to support various stages of data analysis, from ingestion to reporting.
"The right analytical platform can reduce the time taken for data analysis considerably."
It is vital to select a platform based on specific business needs, team skills, and expected outcomes. A thorough evaluation of features, scalability and integration capabilities is important in making this decision.
Open Source vs Proprietary Software
The debate between open source and proprietary software remains significant in the landscape of Q data analysis tools. Each option has its advocates, and understanding their merits and drawbacks is essential for making an informed choice.
Advantages of Open Source Tools
Open source tools provide several advantages. One significant aspect is the cost efficiency they offer. Since these tools are available without purchase restrictions, businesses can save significant funds. Moreover, the collaborative nature of open source software often leads to rapid innovation. Developers from around the world contribute to improving the tools, making them more robust over time.
A key characteristic of open source tools is their flexibility. Users can modify the software to suit their unique analytical needs. Popular open source tools, such as R and Python, have extensive libraries to support various data analysis tasks. Their wide adoption in the community means plenty of resources and support are available.
- Cost-Effective: Reduces initial investment
- Flexible Customization: Tailored functionalities
- Vibrant Community Support: Abundant resources available
Limitations of Proprietary Solutions
Proprietary solutions present both advantages and disadvantages. They often come with dedicated support and easier user interfaces. This can be appealing for organizations seeking a quick solution. However, they also come with limitations. One major aspect is the cost. Users must often pay licensing fees, which can become quite substantial over time.
A notable drawback of proprietary software is the lack of customization. Unlike open source options, users cannot modify the software to their liking. This restriction may limit organizations in achieving specific analytical goals. Examples of prominent proprietary software include SAP and Oracle solutions, both of which offer powerful features but come at a high price.
- Dedicated Support: Accessible technical assistance
- User-Friendly Interfaces: Easier for non-technical users
- High Costs: Requires budget consideration
When selecting between open source and proprietary tools, organizations must consider their budget, needs, and expertise. This decision will significantly shape their data analysis capabilities and long-term strategy.
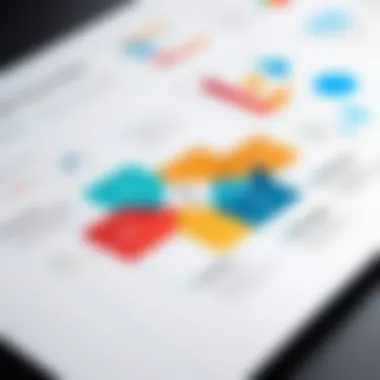
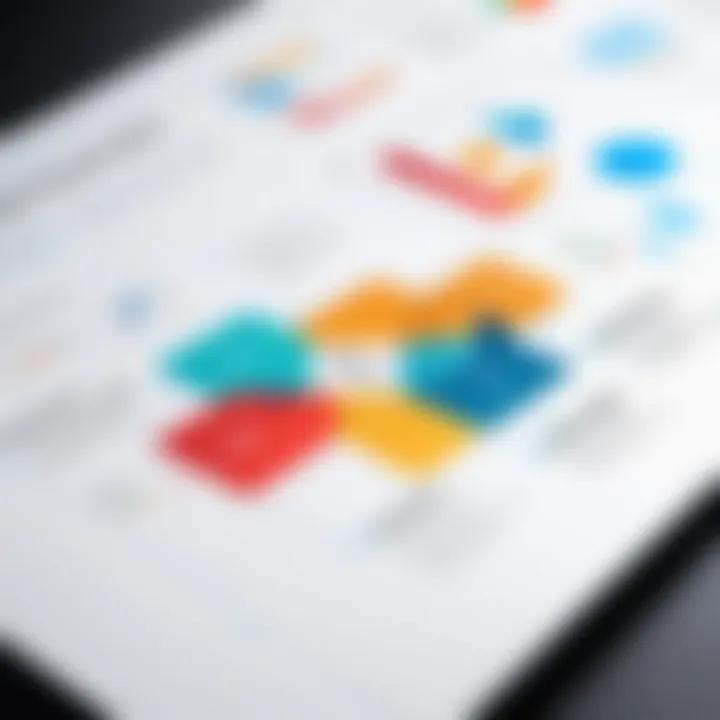
Application of Q Data Analysis in Business
The application of Q data analysis in business represents a crucial dimension in todayβs data-driven landscape. It enables organizations to harness vast amounts of information for practical decision-making and strategic planning. This section explores how Q data analysis can lead to enhanced business intelligence and contribute to operational efficiencies, thereby reinforcing the position of companies in competitive environments.
Driving Business Intelligence
Business intelligence is fundamentally about leveraging data to inform decision-making at all levels of an organization. With Q data analysis, businesses can access relevant insights that lead to improved strategies across various sectors.
- Enhanced Decision-Making: Q data analysis empowers decision-makers by providing them with actionable insights derived from complex datasets. This information can range from customer behavior patterns to market trends, enabling organizations to make informed choices.
- Predictive Analytics: Through predictive analytics, businesses can forecast future trends based on historical data, allowing for proactive planning. This capability is vital for mitigating risks and capitalizing on emerging opportunities.
- Data Visualization and Reporting: Effective visualization tools associated with Q data analysis simplify the interpretation of data. This means that insights can be communicated more clearly to stakeholders, aiding in collaborative decision-making processes.
"Effective data-driven decisions can drastically improve an organizationβs adaptability in a rapidly changing market."
Data analytics contributes significantly to understanding customer preferences, thus enhancing customer experience. By tailoring products or services according to consumer needs, businesses can improve loyalty and satisfaction levels.
Optimizing Operational Efficiency
Operational efficiency is a key goal for any organization looking to maximize resource utilization and minimize waste. Q data analysis contributes to this through several mechanisms:
- Process Improvement: By analyzing workflows and operations, companies can identify bottlenecks and inefficiencies. This analysis leads to re-engineering processes that streamline operations.
- Resource Allocation: Analyzing data allows for more intelligent resource allocation. Understanding where resources are under or over-utilized helps in planning better, ultimately reducing costs.
- Performance Monitoring: Q data analysis provides the metrics needed to monitor various operational parameters. Keeping tabs on these metrics ensures organizations can quickly respond to issues before they escalate.
Challenges in Q Data Analysis
Understanding the challenges in Q data analysis is essential for anyone involved in data-driven decision-making. This section examines the obstacles that come with data quality issues and scalability concerns. Recognizing these challenges allows organizations to mitigate risks and improve their analytical practices, ultimately leading to better business outcomes.
Data Quality Issues
Data quality is a critical component in Q data analysis. High-quality data ensures more accurate insights and reliable decision-making. However, poor data quality can lead to misleading conclusions, wastage of resources, and implementation of ineffective strategies. Some common problems associated with data quality include:
- Inaccuracy: Data that is not correct can stem from human error, faulty data entry, or outdated information sources.
- Inconsistency: Variation in data formats and standards across departments can create confusion and lead to discrepancies in reporting.
- Completeness: Missing data points can restrict the capacity for thorough analysis, thereby ignoring vital information that could impact decision-making.
Organizations need to implement strict data governance standards. This practice involves establishing protocols for data collection, storage, and usage. By doing so, they can enhance data quality, leading to improved analytical accuracy and reliability.
"Maintaining high data quality is not just a technical necessity but a strategic imperative for organizations."
To address these issues, businesses can adopt several strategies:
- Conduct regular data audits to identify and rectify inaccuracies.
- Implement standardized data entry protocols across all departments to achieve consistency.
- Use data cleaning tools to fill in the gaps and ensure comprehensive datasets are available.
Scalability Concerns
Scalability is another significant challenge in Q data analysis. As organizations grow, the volume of data they need to analyze typically increases. The existing systems might not be capable of handling this increased demand, which can lead to delays and inefficiencies.
Some key aspects to consider regarding scalability include:
- Infrastructure limitations: Current data processing systems might require extensive upgrades or replacements to handle larger datasets effectively.
- Increased complexity: As more data sources are integrated, the architecture can become complicated, making it difficult to manage and analyze data efficiently.
- Performance issues: If systems to not scale appropriately, they can suffer from lag or crashes during peak usage.
To mitigate these concerns, organizations should consider adopting scalable solutions from the beginning. Utilizing cloud-based data platforms can offer flexibility and agility in managing large datasets. Furthermore, leveraging distributed computing frameworks like Apache Spark can enhance processing capabilities, making it easier to analyze vast amounts of information without significant performance drawbacks.
Adapting to changing needs without compromising performance is vital for organizations to remain competitive. Recognizing scalability not merely as a hurdle but as an opportunity for growth allows businesses to prepare adequately.
By addressing both data quality and scalability issues, businesses can reinforce their analytical capabilities, leading to more insightful decision-making.
The Role of Data Governance
Data governance plays a crucial role in Q Data Analysis. It establishes the framework for managing data assets throughout an organization. Effective data governance ensures that data is accurate, consistent, and secure. Understanding its importance can drive better decision-making, improve compliance and enhance operational efficiency. Companies increasingly use their data as a strategic asset. Poor data governance can result in inconsistencies, compliance issues, and misuse of data which can have severe repercussions.
Effective data governance leads to several benefits:
- Enhanced Data Quality: It delineates clear standards for data management. This ensures that data is reliable and relevant, both critical for accurate analysis.
- Increased Trust in Data: Governance frameworks instill confidence among stakeholders. When data is governed properly, users are more likely to trust the insights derived from it.
- Regulatory Compliance: Organizations must navigate a complex landscape of regulations. Governance ensures that data policies meet compliance requirements, thus avoiding legal issues and fines.
- Improved Decision Making: With quality data at hand, decision-makers can base their strategies on reliable evidence, thus achieving better business outcomes.
Organizations must consider various elements in establishing data governance frameworks. This includes defining roles and responsibilities, creating data stewardship programs, and developing policies for data lifecycle management. Lack of clarity in these areas can lead to disputes and inefficiencies.
Importance of Data Standards

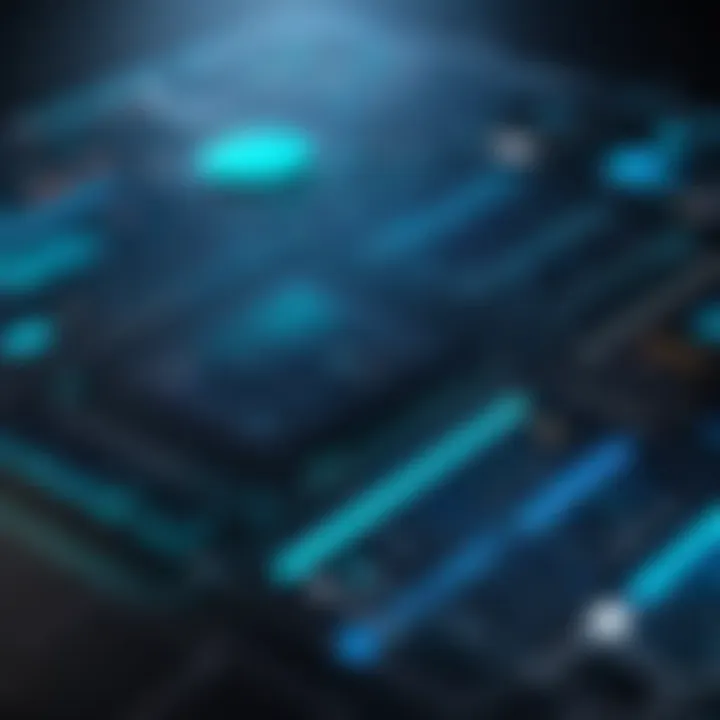
Data standards are integral to data governance. They provide a uniform way to define, store, and manage data. Adopting standards minimizes confusion and discrepancies, facilitating smooth data sharing across departments and systems. Implementing strong data standards can optimize data storage, retrieval, and processing, thus improving overall analysis effectiveness.
Key points regarding the importance of data standards include:
- Consistency: Standardized data ensures that everyone in the organization interprets data in the same way. This avoids discrepancies that can arise from differing definitions.
- Interoperability: With clear standards, data can easily be shared and utilized across various applications. This widens the potential for collaborative data analysis.
- Efficiency: When data formats and structures are standardized, the processes for data management and analysis become more streamlined.
Compliance with Regulations
In today's data-driven environment, compliance with regulations is a significant aspect of data governance. Organizations must adhere to various data protection laws such as GDPR, HIPAA, and CCPA. Non-compliance can lead to costly penalties and reputational damage.
Key considerations for compliance with regulations include:
- Familiarity with Laws: Organizations must stay informed about relevant laws. Regular training sessions and workshops can keep teams updated on compliance requirements.
- Robust Data Policies: Establishing clear policies on data handling, storage, and sharing helps mitigate risks associated with data breaches and regulatory issues.
- Regular Audits: Conducting audits can identify weaknesses in data management and compliance practices. This aids in promptly rectifying any shortcomings.
"Data governance not only safeguards an organization's data but also empowers it to leverage data effectively and responsibly."
Emerging Trends in Q Data Analysis
In today's data-driven landscape, staying ahead of the curve requires awareness of emerging trends in Q data analysis. Understanding these trends is crucial for businesses aiming to leverage their data for strategic decision-making. This section sheds light on two pivotal trends: artificial intelligence and machine learning, along with real-time data processing. Each trend underscores the evolving nature of data analysis and its implications for future practices in various industries.
Artificial Intelligence and Machine Learning
Artificial intelligence (AI) and machine learning (ML) have become significant components of Q data analysis. These technologies enable advanced data processing and analysis capabilities, moving beyond traditional methods. AI algorithms can analyze large datasets quickly, uncovering patterns that humans might miss. Machine learning techniques, on the other hand, allow systems to learn from data iteratively and improve over time without extensive reprogramming.
The integration of AI and ML into Q data analysis offers several benefits:
- Enhanced Prediction Accuracy: AI models refine predictive analyses by continuously learning from new data inputs.
- Automated Insights: Routine data analysis tasks can be automated, freeing up human resources for strategic initiatives.
- Personalized Experiences: Leveraging machine learning allows businesses to personalize services based on customer data, improving satisfaction and loyalty.
While the advantages are substantial, integrating AI and ML into existing frameworks also presents challenges such as data biases, the need for algorithm transparency, and ethical considerations. Businesses must navigate these complexities to fully exploit these technologies.
Real-Time Data Processing
Real-time data processing is another trend reshaping Q data analysis. The ability to process data instantaneously as it arrives transforms how organizations operate. Businesses can make decisions based on live data, enhancing their responsiveness and agility in a competitive environment.
Key aspects of real-time data processing include:
- Immediate Insights: Organizations gain immediate visibility into operational metrics, enabling timely adjustments to strategies.
- Improved Customer Engagement: Real-time capabilities allow businesses to cater to customer needs instantly, enhancing user experience.
- Risk Management: Instantaneous processing enables better risk detection and mitigation strategies through timely analysis of relevant data.
Implementing real-time data processing can be resource-intensive. It requires robust infrastructure and technology investments. However, the potential increase in efficiency and customer satisfaction can outweigh these costs in many scenarios.
The future of Q data analysis is inexorably linked to advancements in artificial intelligence, machine learning, and real-time processing capabilities.
In summary, as businesses continue to leverage data, understanding and adapting to these emerging trends is vital. By embracing AI, machine learning, and real-time data processing, organizations can enhance their analytical capabilities and drive value in their operations.
Future Perspectives
The future of Q data analysis stands as a promising frontier for businesses navigating the complexities of data-driven environments. As organizations increasingly rely on advanced analytical capabilities, understanding the upcoming shifts in this domain becomes essential. This section aims to highlight the significance of future perspectives in Q data analysis, exploring specific elements, benefits, and considerations that should be taken into account by stakeholders in diverse business sectors.
The Evolution of Data Analysis
Data analysis has undergone a profound transformation in recent years. Initially focused on basic statistical analysis, it has evolved into a sophisticated process that leverages advanced technologies, including artificial intelligence and machine learning. The current landscape sees analysts utilizing complex algorithms and vast datasets to derive insights that were previously unattainable.
This evolution is characterized by several key trends:
- Integration of AI and ML: Businesses are now incorporating AI and machine learning frameworks into their data analysis processes, enhancing predictive capabilities and providing deeper insights.
- Focus on Real-Time Analytics: The demand for instantaneous data insights is rising. Organizations seek tools that allow them to process data and make decisions in real time.
- User-Friendly Interfaces: As data analysis becomes more critical, software platforms are evolving to offer user-friendly interfaces, allowing even non-technical users to extract valuable insights without extensive training.
For decision-makers, understanding these changes is crucial for staying competitive. As technologies evolve, businesses must prioritize adapting to these innovations, ensuring they make the most of available data.
Anticipated Developments in Technologies
Looking forward, several anticipated technological advances will undoubtedly shape Q data analysis in the coming years. These developments promise to enhance the capability and efficiency of data analysis, offering organizations new ways to leverage their data assets.
Some notable upcoming trends include:
- Enhanced Analytical Tools: Expect the continuation of improvements in analytical tools, where platforms become more integrated and intelligent. This could lead to smoother workflows and more robust data management.
- Automated Insights: As automation evolves, more tools will provide automated insights without the need for extensive human intervention, streamlining decision-making processes.
- Greater Data Accessibility: Technologies focusing on democratizing data access will emerge, allowing individuals with different skill levels to engage with data more easily.
- Cloud Computing Growth: Cloud solutions will continue to expand, offering scalable infrastructure for data storage and analysis, making it easier for businesses to manage their data needs.
- Data Privacy Enhancements: With growing concerns around data security, future developments will likely focus on ensuring data privacy while maintaining the ability to analyze data effectively.
As organizations embrace these advancements, their ability to make informed decisions will improve significantly. The rise of innovative technologies will create new opportunities for businesses to harness the full potential of their data.
By recognizing the significance of these trends and preparing for them, stakeholders can position themselves advantageously in an increasingly competitive landscape.