Exploring Predictive Maintenance Across Industries
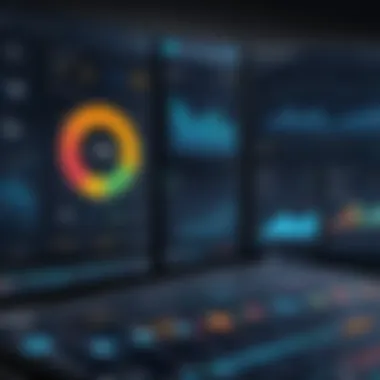
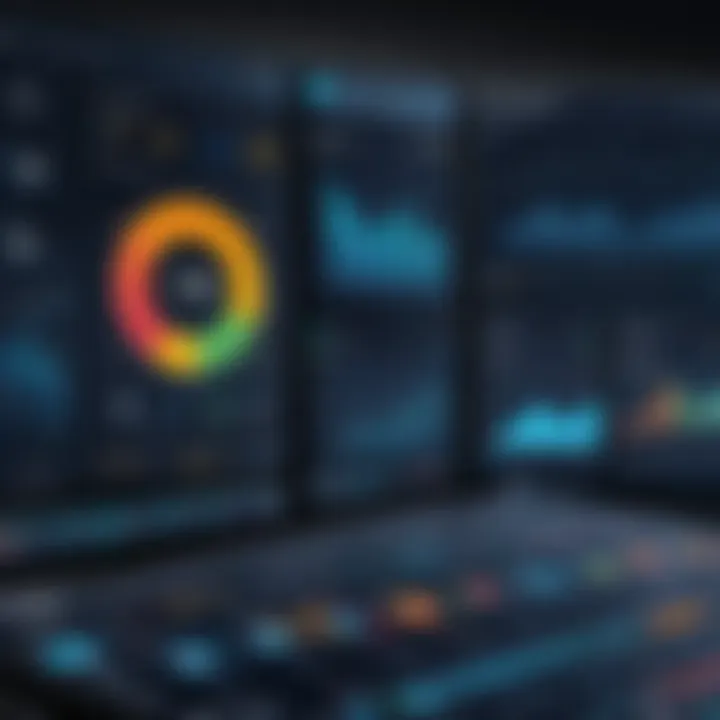
Intro
Predictive maintenance has emerged as a crucial strategy for enhancing operational efficiencies across various industries. With the rapid advancement of technology, businesses now have access to sophisticated tools and methodologies that help anticipate equipment failures before they occur. This proactive approach leads to minimized downtime and significant cost savings.
The ability to predict when equipment is likely to fail relies heavily on data analysis and sensor technologies. These tools gather real-time data to assess the condition of equipment, presenting a clear picture of the systemβs health. By analyzing this data, organizations can make informed decisions, optimizing their maintenance schedules and resource allocation.
Given its applicability across diverse sectors, from manufacturing to healthcare, understanding predictive maintenance is paramount. This article will explore key features, the integration of predictive maintenance into existing processes, associated tools, and the tangible benefits it offers across different fields.
Understanding Predictive Maintenance
Predictive maintenance is a strategic approach that has gained significant traction in various industries. Its importance lies in the ability to foresee potential equipment failures before they occur. This proactive method starkly contrasts with traditional maintenance practices, which often rely on scheduled checks or reactive responses to equipment breakdowns. By understanding predictive maintenance, organizations can optimize their operations through improved reliability and reduced costs associated with unexpected downtimes.
The benefits of predictive maintenance are far-reaching. Primarily, it enhances operational efficiency. With the right tools and technologies, businesses can monitor the health of their machinery in real time. This insight leads to better decision-making regarding when maintenance should occur, aligning it seamlessly with production schedules. Organizations can also realize significant cost efficiencies. By addressing issues before they escalate, firms can avoid expensive repairs and the costs related to idle time.
However, understanding predictive maintenance is not solely about the advantages. Considerations such as initial investment, training, and integration with existing systems must also be explored. These factors can influence how effectively an organization can implement predictive maintenance strategies. Recognizing these dimensions is essential for achieving a comprehensive understanding of this field.
Definition of Predictive Maintenance
Predictive maintenance can be defined as a proactive maintenance strategy that utilizes data-driven insights to forecast when equipment might fail. This methodology relies heavily on various data collection methods. These include sensors, historical performance data, and analytics tools. By analyzing this data, organizations can identify patterns and anomalies that indicate potential failures. Thus, predictive maintenance allows for timely interventions, ensuring that machines operate at optimal efficiency.
Historical Context
The roots of predictive maintenance can be traced back to the evolution of industrial operations. Initially, maintenance practices were rudimentary, often relying on technicians to conduct regular checks based on a fixed schedule. As technology developed, the concept began to shift.
The introduction of computerized maintenance management systems in the 1980s marked a significant turning point. These systems provided a structured way to track and manage maintenance activities. The advent of the Internet of Things in the 2000s further propelled predictive maintenance into mainstream industry applications. Now, equipment can be monitored in real time, and data can be shared seamlessly across various platforms, significantly enhancing the effectiveness of predictive strategies.
The evolution of predictive maintenance reflects a broader shift towards data-driven decision-making in modern industries.
Thousands of companies have begun to integrate predictive maintenance into their operations. The focus has shifted from merely keeping machines running to ensuring they run optimally, a paradigm that fundamentally changes the way maintenance is perceived in industrial environments.
Key Principles of Predictive Maintenance
Predictive maintenance (PdM) is an essential approach in maintaining operational efficiency and reducing costs across multiple industries. Understanding the key principles of predictive maintenance is crucial. It informs the integration of various methods that enhance decision-making processes, influence equipment lifecycle management, and ultimately improve a company's bottom line. These principles revolve around data collection and analysis, which are fundamental in predicting potential failures before they happen.
Data Collection Techniques
Data collection is the first step in a successful predictive maintenance strategy. It involves gathering relevant data from various sources, which is crucial for accurate assessment and analysis. A few effective techniques include:
- Sensors: Deploying condition monitoring sensors on equipment helps capture variable like temperature, pressure, and vibration in real time. This data provides insights into the operational state of machinery.
- Automated Data Logs: Many modern machines come equipped with built-in data logging capabilities. These logs automatically store operational data, making it accessible for future analysis.
- Human Input: Operators often have valuable insights that can be collected through surveys or interviews. Their firsthand experience can complement raw data input.
Applying these varied techniques allows companies to compile comprehensive datasets that inform predictive models. A rich repository of data is key to effective analysis.
Analysis Methodologies
Once data is collected, the next step is employing appropriate analysis methodologies that can extract actionable insights. Predictive maintenance relies heavily on statistical analysis, machine learning, and data mining techniques. Some commonly utilized methods include:
- Statistical Process Control (SPC): This method uses statistical tools to monitor and control processes. It helps identify variability in a process that may indicate a need for maintenance.
- Regression Analysis: This technique evaluates relationships among variables. Predictive maintenance can utilize regression models to predict equipment failure based on historical data.
- Machine Learning Algorithms: These algorithms learn patterns from historical data. Advanced methodologies can include supervised learning, unsupervised learning, and reinforcement learning, which enhance the accuracy of predictions.
Implementing these methodologies significantly enhances the ability to foresee failures. By focusing on data points that signal potential issues, organizations can mitigate downtime and effectively allocate maintenance resources.
"Investing in predictive maintenance leads to substantial long-term savings and enhanced operational efficiency."
Adopting principles of predictive maintenance enables organizations to shift their maintenance strategy from reactive to proactive. The benefits manifest through reduced operational costs, enhanced asset reliability, and improved service quality. By recognizing the significance of data collection and analysis, businesses position themselves to thrive in an increasingly competitive landscape.
Technologies Enabling Predictive Maintenance
The effectiveness of predictive maintenance hinges significantly on the technologies facilitating it. These technologies not only ensure smooth integration into existing work processes but also enhance the overall efficacy of maintenance strategies. They empower organizations to gather real-time data, perform sophisticated analyses, and ultimately, make informed operational decisions.
IoT Integration
The Internet of Things (IoT) plays a pivotal role in predictive maintenance. By connecting various equipment and devices to a network, IoT enables the collection of vast amounts of data automatically. This real-time data is critical for identifying potential issues before they escalate, providing organizations with the opportunity to address problems proactively.
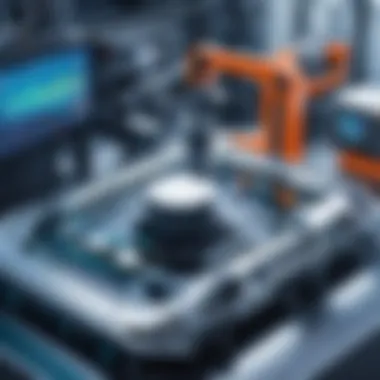
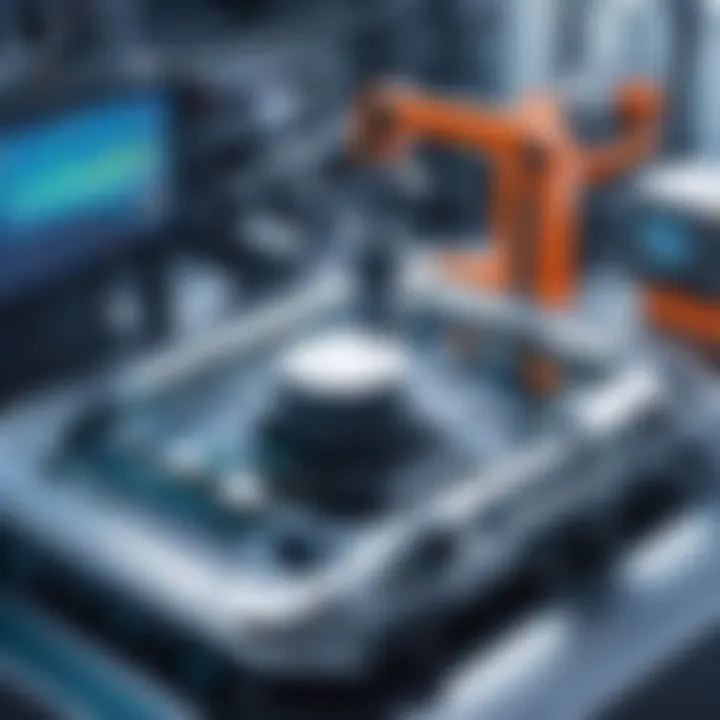
Key benefits of IoT integration include:
- Enhanced Monitoring: Devices equipped with IoT capabilities can transmit performance metrics continually, allowing for better oversight of equipment condition.
- Data Utilization: Collected data aids in uncovering patterns and trends that inform maintenance schedules and resource allocation.
- Increased Connectivity: Integration across different devices fosters interoperability, ensuring that systems communicate effectively to streamline operations.
Despite its advantages, IoT integration also comes with considerations. Security and privacy concerns are paramount; organizations must safeguard sensitive data against cyber threats. Additionally, the infrastructure to support IoT technologies needs to be robust, which can entail a significant investment.
Artificial Intelligence and Machine Learning
Artificial Intelligence (AI) and Machine Learning (ML) are transforming predictive maintenance by offering superior analytical capabilities. These technologies analyze the massive datasets generated by IoT devices, identifying nuances that human analysts might miss. AI algorithms, particularly machine learning models, can predict failures with remarkable accuracy based on historical data and current trends.
Benefits include:
- Predictive Analytics: AI systems can assess when equipment is likely to fail, allowing maintenance teams to preempt issues and reduce unexpected downtime.
- Improved Decision-Making: The insights generated from AI analyses lead to more strategic planning and resource allocation.
- Continuous Learning: ML systems adapt over time, improving their predictive capabilities as they learn from new data and outcomes.
Organizations implementing AI and ML must address challenges such as the need for skilled personnel who can interpret the results and a reliable data input process to avoid inaccurate predictions.
Sensors and Monitoring Devices
Sensors are the backbone of predictive maintenance. These devices collect critical information about equipment conditions, such as temperature, vibration, and pressure. With accurate data in hand, organizations can make precise maintenance decisions.
The importance of sensors and monitoring devices lies in:
- Real-Time Data Acquisition: Sensors enable instant data collection, which is vital for monitoring the health of machinery continuously.
- Condition-Based Maintenance: This approach assesses equipment's actual condition rather than relying solely on time-based maintenance schedules.
- Wide Applicability: Sensors can be utilized across different industries, from manufacturing to healthcare, to support predictive maintenance initiatives.
While sensors are beneficial, challenges in their implementation must be considered. Calibration and maintenance of these devices are essential to ensure accurate readings. Additionally, organizations must integrate sensor data into their analysis frameworks effectively.
"Implementing the right technologies for predictive maintenance is not just about efficiency; it's about transforming how organizations approach maintenance strategies for longevity and sustainability."
Applications Across Various Industries
Predictive maintenance has emerged as an essential approach across multiple industries. Its relevance lies in the ability to enhance operational efficiencies and reduce unnecessary costs. By proactively addressing equipment issues before they escalate, organizations can secure significant savings and improve service reliability. The applications of predictive maintenance vary widely, but the core benefits remain consistent: minimized downtime, improved asset management, and better resource allocation.
Manufacturing Sector
In the manufacturing sector, predictive maintenance plays a pivotal role in keeping production lines running smoothly. Continuous monitoring of machine performance helps identify potential failures early. This is especially crucial for complex manufacturing systems where a breakdown can halt production entirely.
The use of sensors to gather real-time data about equipment health is a key technique. With this data, companies can analyze trends and behaviors of their machinery. For instance, a manufacturing plant utilizing predictive maintenance might track vibration and temperature readings from their machines. If these readings deviate from normal range, maintenance can be scheduled before a failure occurs, thus avoiding costly downtime.
Transportation and Logistics
In transportation and logistics, predictive maintenance enhances vehicle and equipment reliability. Fleet operators can monitor the condition of their vehicles through on-board diagnostics systems. This allows for data-driven decisions regarding vehicle maintenance and repairs.
For example, a logistics company might analyze data on fuel efficiency, tire wear, and engine performance. By addressing issues early, companies can reduce the risk of breakdowns that could disrupt supply chains and delivery schedules. Effectively managing fleet maintenance leads to better service levels and customer satisfaction.
Energy Sector
The energy sector also benefits significantly from predictive maintenance applications. Power plants and renewable energy facilities use advanced monitoring systems to oversee the health of turbines and generators. The ability to preemptively address mechanical failures is vital for maintaining energy supply and operational stability.
Through data analysis, energy companies can predict when equipment is likely to fail. This involves examining historical data, operating environments, and performance metrics. When potential issues are identified, maintenance can be scheduled during non-peak hours, ensuring minimal impact on energy production.
Healthcare Applications
In healthcare, predictive maintenance is increasingly recognized for its impact on medical equipment management. Hospitals rely on various devices and machines that require constant operational efficiency. Predictive maintenance allows healthcare providers to ensure that equipment like MRI machines or ventilators are functioning optimally.
By monitoring the performance of medical devices and using data analytics, healthcare facilities can preemptively address potential device failures. This ensures that critical equipment is available when needed, ultimately safeguarding patient care. Predictive maintenance in healthcare can lead to significant cost savings and enhance the overall quality of care.
Predictive maintenance in diverse sectors illustrates its versatility and importance in modern operations.
Benefits of Predictive Maintenance
The importance of predictive maintenance cannot be overstated. It transforms how industries maintain and manage their operations. This section explores the specific benefits that predictive maintenance offers, which includes cost efficiency, minimized downtime, and enhanced equipment lifespan. By understanding these benefits, organizations can formulate better strategies that contribute to overall efficiency and productivity.
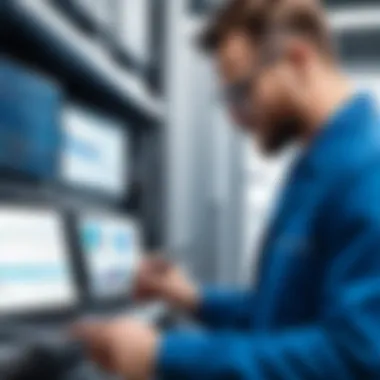
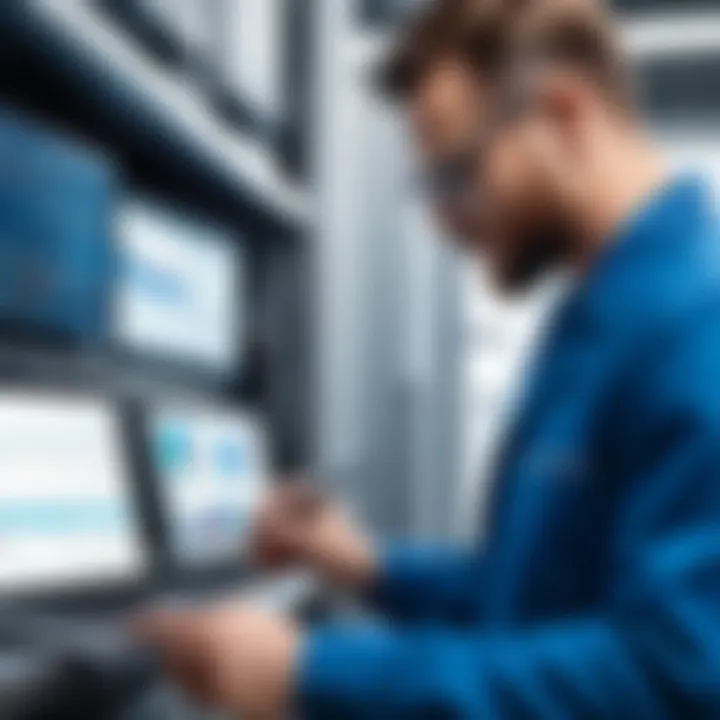
Cost Efficiency
One of the primary benefits of predictive maintenance is cost efficiency. This approach shifts maintenance practices from being reactive to proactive. Companies can significantly reduce the costs associated with unexpected failures and repairs.
By predicting failures before they occur, businesses can schedule maintenance during non-peak hours. This optimization reduces the labor costs and minimizes the financial impact associated with emergency repairs. Moreover, predictive maintenance techniques allow for better inventory management of spare parts.
Using tools like sensors and data analytics, organizations can monitor equipment health in real-time. This leads to smarter purchasing decisions when ordering parts, reducing excess stock, and saving money. In turn, these savings on maintenance and repair can be reinvested into other vital areas of the business, boosting overall performance.
Minimized Downtime
Minimized downtime is another essential benefit that predictive maintenance brings to the table. Unplanned equipment failures can halt production lines, leading to significant financial losses. Predictive maintenance mitigates this risk by monitoring the condition of equipment continuously.
When organizations implement predictive maintenance, they get real-time alerts about potential issues. These alerts enable maintenance teams to address problems before equipment failures happen, effectively reducing the frequency and duration of downtime.
This proactive approach helps maintain steady productivity levels. With fewer disruptions to operations, businesses can better meet customer demand and optimize performance metrics across the board. Preventing downtime also fosters a more reliable service reputation, which is critical in a competitive landscape.
Enhanced Equipment Lifespan
Enhanced equipment lifespan is a significant advantage of predictive maintenance. The methodology emphasizes maintaining equipment at the right time rather than relying on scheduled maintenance regardless of the actual condition. By applying this strategy, organizations can extend the usable life of their machinery.
Regular monitoring enables businesses to identify and rectify issues before they escalate. This leads to less wear and tear on the equipment and better overall operational efficiency. Moreover, maintaining equipment at optimal performance levels ensures that it operates effectively and safely, reducing the risk of accidents linked to malfunctioning machinery.
In summary, predictive maintenance fosters a sustainable approach to equipment management.
Predictive maintenance significantly enhances operational efficiency and sustainability through proper asset management.
By focusing on cost efficiency, minimizing downtime, and enhancing equipment lifespan, organizations can not only optimize their maintenance practices but also position themselves strategically in their respective markets. The comprehension of these benefits is critical for decision-makers who seek to leverage predictive maintenance for continuous improvement.
Challenges in Implementing Predictive Maintenance
Predictive maintenance offers numerous advantages but also faces several challenges that can hinder its implementation. A clear understanding of these challenges sets a base for organizations aiming to adopt predictive maintenance effectively. These challenges range from complex data management to the intricacies of integrating predictive maintenance systems with existing legacy infrastructure. Addressing these challenges is crucial for leveraging the full potential of predictive maintenance and optimizing operational efficiencies.
Data Management Issues
Data management represents one of the most prominent challenges in implementing predictive maintenance systems. Organizations generate vast amounts of data from a variety of sources, including IoT sensors, machinery reports, and operation logs. Managing this data effectively involves several considerations:
- Volume and Variety: The sheer volume of data can be overwhelming. Traditional data management systems may struggle to handle this influx. Additionally, data comes in various formats, requiring specific strategies for integration and analysis.
- Quality of Data: Accurate predictions rely significantly on the quality and integrity of the data. Inaccurate or incomplete data can lead to unreliable maintenance predictions. Hence, continuous data validation and cleansing are necessary.
- Real-time Data Processing: Predictive maintenance efforts rely on timely data insights. Organizations must have systems capable of real-time analysis for effective decision-making.
A thorough approach to data management can facilitate significant improvements in predictive maintenance efficacy.
Integration with Legacy Systems
Integration with legacy systems presents another major hurdle. Many organizations operate with established systems that are not designed to interact with new predictive maintenance technologies. This challenge manifests in several key areas:
- Compatibility Issues: New predictive maintenance technologies must be compatible with existing infrastructure. This often requires customization or even replacement of legacy systems, which can be costly and time-consuming.
- Resistance to Change: Employees accustomed to legacy systems may resist transitioning to new technologies. Overcoming this human factor involves training and change management strategies to ensure a smooth transition.
- Cost of Transition: Implementing predictive maintenance can require significant investments in both technology and training to ensure successful integration. Organizations need to weigh these costs against expected benefits to make informed decisions.
Future Trends in Predictive Maintenance
Predictive maintenance is an evolving domain, shaped significantly by technological advancements and shifting industry needs. As companies strive for reduced operational costs and enhanced efficiency, understanding future trends becomes crucial. This section examines pertinent trends, providing insights into the trajectory that predictive maintenance may take. Gaining clarity on these trends allows businesses to better prepare and adapt their strategies effectively.
Evolution of Machine Learning Algorithms
Machine learning is transforming predictive maintenance by refining data analysis capabilities. Traditional predictive maintenance often relied on simple statistical methods. However, current enhancements focus on the application of sophisticated algorithms. These algorithms can learn from historical data patterns and continuously improve their accuracy over time.
Machine learning enables the identification of subtle anomalies that may precede equipment failure, which might not be detectable through standard methods. Predictive models can classify and forecast potential failures with a higher degree of confidence, resulting in more timely interventions.
Some key points include:
- Adaptive Learning: Algorithms evolve as they gather more data, adapting to new patterns and improving predictive accuracy.
- Real-Time Processing: Advanced models can analyze data in real-time, providing immediate insights.
- Integration with Other Technologies: As machine learning matures, it is increasingly integrated with IoT and edge computing, leading to more decentralized data processing and decision-making.
By leveraging these advancements, businesses can achieve smart predictive maintenance that not only mitigates risk but also optimizes resource allocation and operational efficiency.
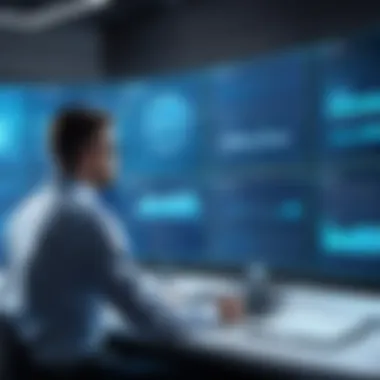
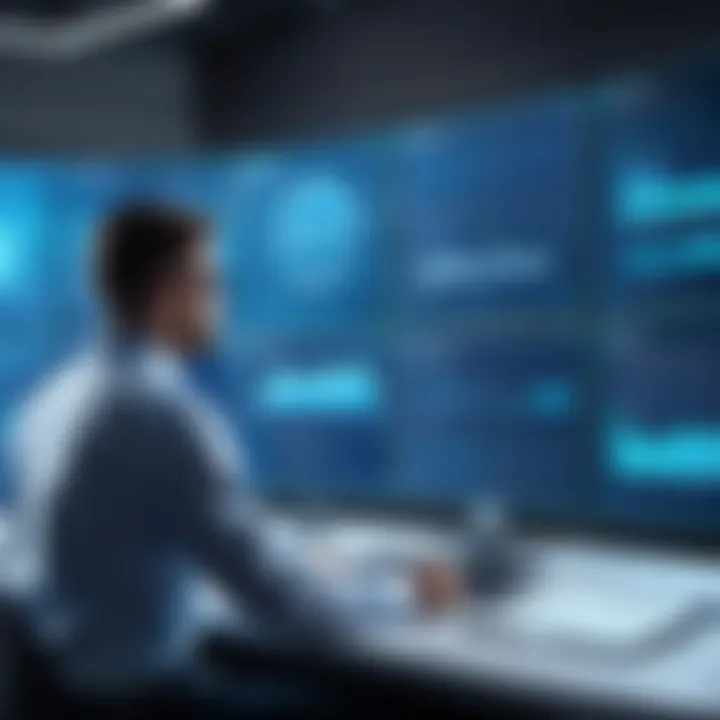
Role of Big Data Analytics
Big data analytics is fundamentally changing how predictive maintenance strategies are formulated. The sheer volume of data generated by IoT devices and sensors requires robust analytic processes to extract actionable insights. Organizations that harness big data are able to uncover patterns and trends that drive their maintenance strategies.
The relevant aspects of big data analytics in predictive maintenance include:
- Data Integration: It facilitates the amalgamation of diverse data sources for holistic analysis. This leads to a comprehensive understanding of equipment performance and health.
- Enhanced Predictive Capability: With superior analytical tools, businesses can predict when failures are likely to occur with a solid degree of reliability.
- Proactive Decision Making: The insights from data analytics support preventive measures, allowing companies to address potential issues before they escalate.
An effectively implemented big data strategy not only aids in predicting failures but also enhances operational decisions across the board, ensuring that maintenance processes are proactive rather than reactive.
"Predictive maintenance is not just about keeping machines running; it's about enabling businesses to function efficiently and effectively with optimal resource management."
Case Studies and Real-World Examples
Case studies serve as a vital piece of the puzzle when discussing predictive maintenance. They provide tangible evidence of how predictive maintenance is applied in real-world scenarios. By examining these examples, readers can gain valuable insights into the practical applications, benefits, and challenges associated with implementing predictive maintenance strategies. These case studies showcase the effectiveness of predictive maintenance across various industries and highlight how organizations can achieve significant improvements in efficiency and productivity.
Key elements of case studies include:
- Real-world data and outcomes: Understanding what actually happened in various situations.
- Application of technologies: Observing how tools were implemented in each context.
- Lessons learned: Gaining insights from both successes and failures.
Through these examples, business professionals can better appreciate the nuances of predictive maintenance and consider its potential for their operations.
Case Study: Manufacturing Efficiency
In the manufacturing sector, predictive maintenance has demonstrated its value through substantial efficiency gains. One notable example involves a leading automotive manufacturer, which faced frequent equipment failures that led to unexpected downtime.
To address this, the company implemented a predictive maintenance solution that integrated IoT sensors on critical machinery. These sensors collected data on machine performance in real-time, which was then analyzed using machine learning algorithms. The goal was to identify patterns indicative of potential failures before they occurred.
As a result of this initiative:
- The manufacturer achieved a 30% reduction in unplanned downtime.
- Maintenance costs decreased by 15%, as repairs were scheduled more strategically.
- Equipment lifespan extended significantly, leading to higher return on investment.
This case illustrates that predictive maintenance can transform traditional practices into proactive strategies, which is not just beneficial but essential in today's competitive market.
Case Study: Healthcare Improvements
The healthcare industry has also reaped rewards from predictive maintenance, particularly regarding medical imaging equipment. A prominent healthcare provider implemented predictive maintenance to improve the uptime of MRI and CT machines.
Through the installation of advanced monitoring tools, the facility began collecting data on machine usage and performance metrics. Analysis revealed trends that signaled when machines were likely to need maintenance. Accordingly, technicians could proactively address issues during scheduled downtimes rather than responding to unexpected breakdowns.
The results were remarkable:
- Patient wait times decreased by 40%, as machines became more readily available.
- Annual servicing costs were reduced by 20%.
- Overall patient satisfaction improved, fostering a better community reputation for the healthcare provider.
This scenario underscores how predictive maintenance enhances operational efficiency and elevates the quality of services in critical sectors like healthcare.
The future of predictive maintenance lies in its ability to transform data into actionable insights, helping businesses across various sectors deliver better performance and service.
Closure
The conclusion of this article serves as a vital synthesis of the key points discussed throughout the various sections. It encapsulates the significance of predictive maintenance across multiple industries, emphasizing its role in enhancing operational efficiency and reducing costs. It is crucial to reflect on how these methodologies integrate into the existing systems of organizations and the benefits they afford.
Summary of Key Insights
In reviewing predictive maintenance, several central insights have emerged:
- Proactive Approach: Rather than relying on reactive maintenance, organizations can minimize unexpected failures by predicting when maintenance is needed.
- Cost Savings: Implementing predictive maintenance leads to significant cost reductions by avoiding emergency repairs and extending equipment lifespan.
- Enhanced Data Utilization: Leveraging data from various sources can lead to informed decision-making that directly influences operational workflows.
- Cross-Industry Applicability: The versatility of predictive maintenance applications spans manufacturing, transportation, energy, and healthcare, showcasing its universal relevance.
These insights underline the importance of adopting predictive maintenance practices as a strategic decision.
Implications for Future Practices
Looking ahead, the implications of adopting predictive maintenance practices in organizations are profound:
- Integration with Advanced Technologies: As technologies like IoT and machine learning evolve, predictive maintenance will further enhance its accuracy and effectiveness.
- Focus on Data-Driven Decisions: Organizations will increasingly rely on data analytics to support maintenance strategies, making data stewardship a key consideration.
- Training and Development: Future workforces will require training to adapt to new tools and methodologies associated with predictive maintenance.
- Sustainability Goals: As companies seek more sustainable operations, predictive maintenance aligns with those goals by reducing waste and resource usage.
The shift to predictive maintenance is not just an operational change; it is a cultural transformation that compels organizations to embrace innovation and data-driven insights.
In summary, the path forward necessitates a strategic embrace of predictive maintenance, influencing not just efficiency but broader organizational practices.