Machine Learning Market Growth Insights by Gartner
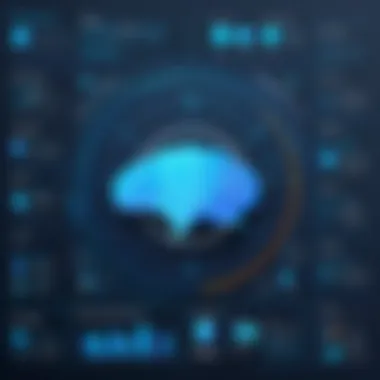
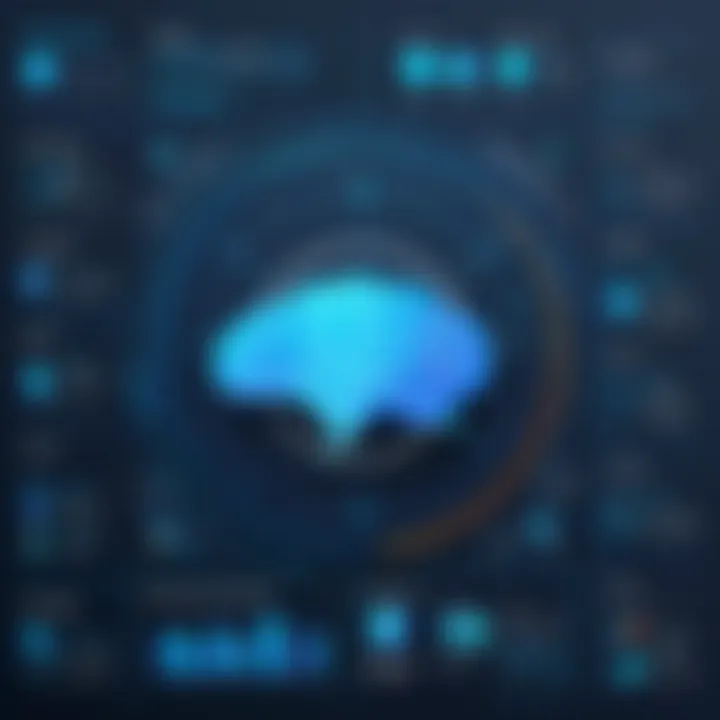
Intro
The machine learning market has seen significant expansion in recent years. Gartner's insights offer valuable perspectives on this evolution. Understanding the factors influencing market size is crucial for businesses and decision-makers eager to harness machine learning's potential. Various elements shape market dynamics, such as technological innovations, investment strategies, and the increasing prevalence of machine learning in diverse industries.
Gartner's analysis outlines the growth trajectory of this market, indicating key growth drivers and regional disparities. As organizations seek to implement machine learning solutions, comprehending these elements becomes essential. Failure to do so may impede the realization of potential benefits and competitive advantages.
In this article, expect a thorough examination of market size, important trends, and future projections. Through comprehensive insights, businesses can effectively navigate the rapidly changing landscape of B2B software solutions. Let's delve deeper into the features and implications of the machine learning market as analyzed by Gartner.
Understanding Machine Learning Fundamentals
Understanding the fundamentals of machine learning is crucial, as it lays the groundwork for analyzing the market size and trends. This section offers insights into machine learning's definition, types, and key components, which are all essential for grasping how machine learning fits within business models and technology landscapes. By comprehending these basics, stakeholders, including business leaders and IT professionals, are better positioned to evaluate opportunities and risks associated with integrating machine learning into their operations.
Definition of Machine Learning
Machine learning is a subfield of artificial intelligence focused on enabling systems to learn from data and improve their performance over time without explicit programming. This process involves algorithms that identify patterns in data, facilitating accurate predictions and decision-making. While often conflated with AI, machine learning specifically deals with the data-driven learning aspect. The significance of this definition extends beyond academics; it directly influences how organizations approach data analytics and predictive modeling.
Types of Machine Learning
Machine learning can be broadly categorized into three primary types: supervised learning, unsupervised learning, and reinforcement learning. Each type serves varied purposes and contexts, impacting how businesses leverage this technology.
Supervised Learning
Supervised learning is characterized by its dependence on labeled training data, where the algorithm learns to map inputs to known outputs. This method is often utilized for tasks like classification and regression, making it immensely popular in business applications. One reason for its favor is the clarity it brings, as results can often be predicted with high accuracy. Moreover, supervised learning systems can be easily evaluated against their pre-known outcomes, allowing for quick debugging and refinement. However, the need for extensive labeled datasets can be a limitation, especially when acquiring such data is time-consuming or costly.
Unsupervised Learning
Unsupervised learning, in contrast, works with unlabelled data. Its primary goal is to discover hidden patterns or intrinsic structures within the data. This method is beneficial for clustering and association tasks, such as customer segmentation or market basket analysis. This learning type is appealing due to its ability to handle large datasets without the need for extensive pre-processing. Its unique feature enables organizations to uncover insights that may not be apparent, leading to innovative business strategies. Nonetheless, the results can be less interpretable, making it difficult to derive actionable conclusions without additional analysis.
Reinforcement Learning
Reinforcement learning is distinctive in that it focuses on agents that learn through trial and error, receiving rewards or penalties based on their actions. This approach has gained traction in fields requiring optimization in decision-making processes, like robotics and gaming. It is a beneficial choice, mainly because it can adapt to complex environments and optimize for long-term rewards. However, it can also be computationally intensive and may require significant time for an agent to learn effectively and find optimal strategies, which might deter its immediate application in certain business contexts.
Key Components of Machine Learning Systems
Machine learning systems are composed of several key components that are essential for their functionality:
- Data: The foundation of any machine learning project is data. Quality and quantity of data directly influence the performance and accuracy of models.
- Algorithms: These are the mathematical representations guiding how data is interpreted, enabling systems to learn from patterns.
- Model Training: This involves using algorithms on training data to create predictive models, with various techniques employed to enhance accuracy.
- Evaluation: After training, models must be evaluated to ensure they perform effectively; this might involve metrics like precision and recall.
- Deployment: Once validated, models are deployed to operational environments, where they can provide real-time predictions or analytics.
By understanding these components, organizations can better strategize their approach to adopting machine learning solutions effectively.
Gartner's Perspective on Machine Learning
Understanding Gartner's viewpoint is crucial in contextualizing the current state and future potential of the machine learning market. Gartner, a renowned research and advisory firm, offers insights backed by data and analysis. Their perspective helps illuminate key trends and influences shaping the market.
By assessing Gartner's findings, businesses can better understand the competitive landscape. Their reports provide valuable market intelligence, guiding companies in strategic decision-making. This understanding aids executives in evaluating investment opportunities and technology adoption.
Overview of Gartner
Gartner, founded in 1979, provides research and advice on information technology. This organization's reputation for thorough analysis has made it a go-to source for businesses seeking to understand market dynamics. Their expertise spans various sectors, including software, hardware, and consulting services.
Using their extensive research capabilities, Gartner analyzes numerous industries. They employ a rigorous methodology that blends both quantitative and qualitative data. This ensures the reliability of their insights. Additionally, their reports often highlight emerging technologies, guiding enterprises in recognizing growth avenues.
Gartner's Research Methods
Gartner uses a multi-faceted approach in conducting research. This includes surveys, interviews with industry experts, and real-world case studies.
Some key methods include:
- Surveys: Collecting data from IT leaders and industry professionals.
- Interviews: Gaining insights from subject matter experts in machine learning.
- Market analysis: Evaluating trends and shifts through quantitative metrics.
Their research often culminates in comprehensive reports. These reports provide a detailed analysis of different market segments, highlighting growth prospects and challenges like data security and talent shortages. The extensive data collection provides stakeholders with confidence in their strategic planning.
Key Reports on Machine Learning
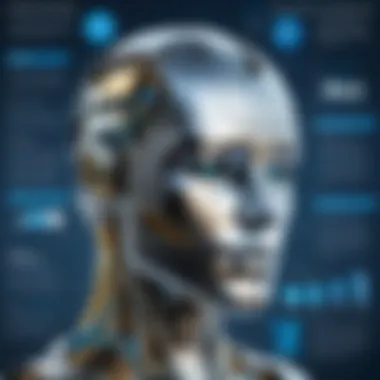
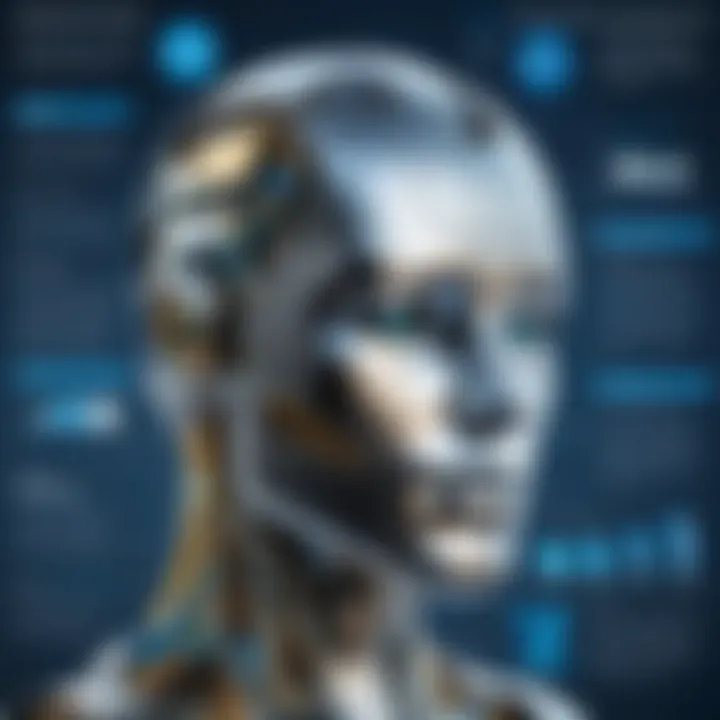
Gartner publishes several pivotal reports assessing machine learning's evolution. Some of the significant reports include:
- Hype Cycle for Artificial Intelligence: This report maps the maturity and adoption levels of diverse AI technologies, including machine learning.
- Magic Quadrant for Data Science and Machine Learning Platforms: It evaluates vendors based on their ability to execute and completeness of vision. This helps businesses determine suitable solutions for their needs.
- Predicts Report: A forward-looking analysis that highlights expectations for technological developments, including machine learning advancements over the next five years.
These publications serve as a vital resource for industry players. They discern critical insights that shape their strategies and operational approaches. By leveraging Gartner's findings, organizations can maintain competitive edges and ensure readiness for future developments in machine learning.
Current Market Size and Growth Rate
Understanding the current market size and growth rate of the machine learning sector is crucial for businesses and stakeholders in technology. From a strategic perspective, comprehending these metrics helps companies evaluate opportunities, formulate competitive strategies, and allocate resources efficiently. Furthermore, the significance of these statistics extends beyond mere numerical values; they reflect the broader trends in artificial intelligence and digital transformation across industries. As such, a deeper insight into market size and growth rate assists organizations in navigating investment and operational decisions.
Market Size Estimates
The machine learning market has experienced significant growth, with various estimates highlighting its expansive nature. According to Gartner's analysis, the current market size is poised at approximately $XX billion. This figure considers a range of factors, including software solutions, services, and infrastructure utilized for machine learning applications.
When examining specific segments within the broader machine learning ecosystem, several domains contribute to this expanding market. These include:
- Predictive Analytics: Tools for forecasting are increasingly integrated into business operations.
- Natural Language Processing (NLP): NLP technologies are used in customer support and interaction.
- Computer Vision: Utilized in industries such as healthcare and security.
The adoption rates across sectors reflect a burgeoning recognition of the benefits machine learning offers, including improved efficiency, enhanced analytical capabilities, and better decision-making processes.
Annual Growth Rate Projections
According to detailed projections provided by Gartner, the annual growth rate of the machine learning market is expected to reach XX% over the next few years. This growth rate is indicative of strong market momentum as enterprises increasingly look to leverage machine learning to enhance operational capabilities.
Several factors drive this growth trend:
- Escalating Demand for Automation: Businesses aim to automate routine tasks, resulting in increased reliance on machine learning technologies.
- Rising Data Volume: The explosion of data generation necessitates advanced analytical tools, positioning machine learning as a viable solution.
- Investment Surge: Venture capital and corporate investments in machine learning have surged, indicating confidence in the market's long-term viability.
"The pace at which machine learning is evolving provides firms with unparalleled opportunities to innovate and enhance operational efficiency."
The projected growth rate highlights not only potential revenue increases for technology providers but also reflects a shift in organizational attitudes toward embracing digital transformation. As businesses of all sizes prepare to adapt, understanding these growth dynamics will be pivotal in maintaining a competitive edge in the landscape of machine learning.
Contributing Factors to Market Dynamics
Understanding the contributing factors to market dynamics is essential for interpreting the growth trends and overall trajectory of the machine learning sector. With the insights provided by Gartner, we can identify various elements that play pivotal roles.
Technological Advancements
Technological advancements represent a cornerstone of the machine learning market's evolution. Breakthroughs in algorithms, hardware capabilities, and data processing infrastructure fuel innovation. Machine learning models have improved in accuracy and efficiency, allowing businesses to derive insights from vast datasets much more quickly than before. The shift towards cloud computing has facilitated access to powerful computational resources without the need for substantial upfront investments in infrastructure. This transition empowers small and medium-sized enterprises to leverage machine learning technologies previously reserved for larger organizations.
Increased Investment in AI Technologies
Investment trends reflect a growing confidence in the capabilities and potential of machine learning. As industries recognize the impact of AI, funding is being directed towards startups and established firms specializing in machine learning solutions. Venture capital investments have surged, with firms seeking to capitalize on the anticipated returns from AI-driven products. In addition, established companies are allocating larger portions of their budgets to R&D, aimed at integrating machine learning into their existing systems and processes.
"The influx of capital into AI technology is vital. It enables the continuous enhancement of machine learning applications across various sectors."
Corporate Adoption of Machine Learning
The corporate landscape is increasingly adopting machine learning as a strategic component of their operations. Many firms now view machine learning as essential rather than optional. This mindset shift stems from the need to stay competitive in a data-driven world. Industries like healthcare, finance, and retail are integrating machine learning to improve decision-making processes and enhance customer experiences. By employing predictive analytics and automated systems, companies can streamline operations, reduce costs, and ultimately drive revenue growth. Furthermore, the growing case studies showcasing successful machine learning implementations serve as compelling evidence for others to follow suit.
Regional Market Analysis
The analysis of regional markets is vital for understanding the nuances within the machine learning ecosystem. It allows stakeholders to identify local trends that can shape investment decisions and business strategies. Different regions exhibit distinct characteristics influenced by their technological infrastructure, regulatory environment, and economic conditions. Grasping these regional dynamics can lead to informed planning and optimized resource allocation for businesses.
North America Insights
North America remains a leader in machine learning innovation. The combination of advanced technology infrastructure and significant venture capital investment creates a conducive environment for machine learning startups and established firms alike. According to recent reports, major tech companies like Google, Amazon, and Microsoft are heavily investing in AI research and development.
The healthcare and finance sectors are rapidly adopting machine learning solutions, driving growth. Many enterprises are using machine learning for predictive analytics, risk assessment, and operational automation.
One key aspect of the North American market is the availability of talent. Numerous universities offer specialized programs in artificial intelligence, leading to a steady influx of skilled professionals into the workforce. However, there is still a reported talent shortage, making competition fierce among companies seeking top-tier talent.
Europe Market Trends
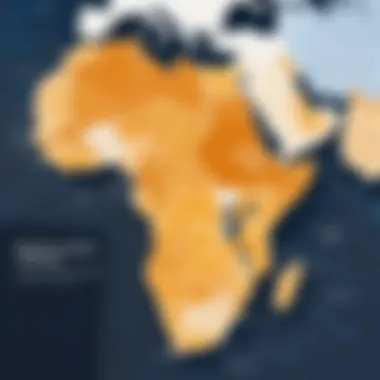
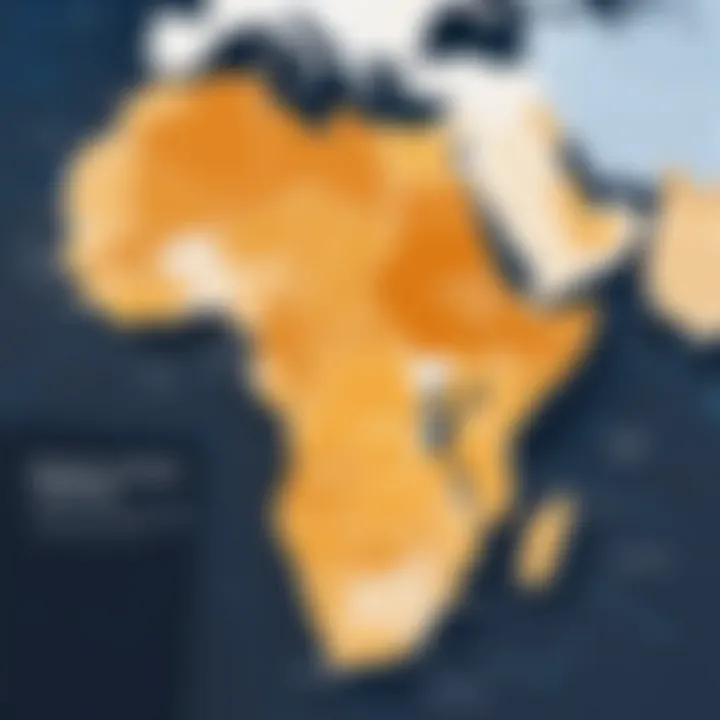
Europe presents a varied landscape for machine learning technologies. Countries like Germany and the UK are at the forefront, while emerging markets in Eastern Europe are gradually adopting these solutions. The ongoing emphasis on data protection and privacy, particularly with regulations like GDPR, significantly impacts machine learning strategies across the continent.
Businesses in Europe are exploring machine learning for areas such as customer personalization and supply chain optimization. The growing trend of digital transformation is pushing several industries to embrace machine learning. This region also sees a rise in government initiatives aimed at fostering AI development. The commitment to ethical AI practices is another critical factor defining the European market, influencing company policies and consumer trust.
Asia-Pacific Growth Opportunities
The Asia-Pacific region is poised for significant growth in the machine learning market. Countries such as China and India are rapidly expanding their digital economies, driven by a young, tech-savvy population and increasing smartphone penetration. The investment in AI technologies is gaining momentum, with both government and private sectors recognizing its transformative potential.
In retail, businesses are leveraging machine learning for inventory management, customer experience enhancement, and predictive analytics.
Manufacturing is also a key sector where companies are adopting machine learning to improve efficiency and reduce costs.
However, various challenges exist. Issues surrounding data privacy and lack of skilled workforce can hinder growth. Addressing these challenges will be crucial for capitalizing on the opportunities in this vibrant market.
"Understanding regional specifics is crucial for maximizing the impact of machine learning strategies across different markets."
The regional market analysis provides a detailed perspective on where businesses should focus their energies. The dynamic nature of machine learning technologies, coupled with the specific characteristics of each region, indicates that staying informed will be essential for future growth and competitiveness.
Industry Applications of Machine Learning
Machine learning has transformed various sectors by enabling organizations to analyze large datasets efficiently. The ability to extract insights and make predictions has made it a vital tool across industries. Understanding these applications provides clarity on the practical benefits and considerations for businesses looking to implement machine learning solutions.
Healthcare Sector
Machine learning is increasingly significant in the healthcare industry. Its applications range from predictive analytics to patient management systems. Algorithms can analyze complex medical data to predict disease outbreaks or improve patient diagnoses. For instance, tools built on machine learning assist radiologists with imaging interpretations, enhancing accuracy. This leads to quicker diagnoses and ultimately better patient outcomes.
Moreover, machine learning supports personalized medicine. By analyzing genetic information, treatments can be tailored to individual patients. This precision allows for more effective therapies with fewer side effects. The integration of machine learning in healthcare not only optimizes operational efficiency but also enhances the quality of care provided.
Finance and Banking
In finance, machine learning models are crucial for fraud detection and risk management. By analyzing transaction patterns, financial institutions can identify anomalies and prevent fraudulent activities promptly. The dynamic nature of financial markets makes it necessary for banks to adopt machine learning to stay competitive.
Additionally, these models are used for credit scoring, enabling banks to offer loans with better insights on applicant risk. Machine learning streams vast amounts of financial data to deliver real-time predictions and insights, thereby aiding strategic decision-making. This efficiency can lead to lower operational costs and improved customer satisfaction, as clients receive faster responses and personalized service.
Retail and E-commerce
The retail sector benefits immensely from machine learning through enhanced customer experiences and optimized inventory management. E-commerce companies like Amazon use algorithms to analyze customer behavior. This analysis informs product recommendations, engaging visitors and often resulting in increased sales. Personalized marketing strategies driven by machine learning further enhance consumer connection.
Additionally, retail businesses utilize predictive analytics for inventory management. By forecasting demand, they can maintain optimal stock levels, reducing costs associated with overstock or stockouts. Machine learning streamlines operations in the supply chain, ensuring that products are replenished efficiently based on consumer trends.
Challenges in the Machine Learning Market
Machine learning presents vast opportunities, but it also faces significant hurdles. Grasping these challenges is essential for stakeholders involved in the machine learning market. By scrutinizing these limitations, organizations can devise strategic approaches to mitigate risks and enhance their operational efficiency. This section explores two critical challenges: data privacy and security issues, alongside the talent shortage in the industry.
Data Privacy and Security Issues
As businesses turn to machine learning for insights, the handling of sensitive data emerges as a pressing concern. Data privacy regulations, such as the General Data Protection Regulation (GDPR) in Europe, impose strict guidelines on data collection and processing. Non-compliance can lead to severe legal repercussions and loss of consumer trust, making it imperative for organizations to understand these regulations.
Secure data management practices are essential. Compromised data can not only harm a company's reputation but also compromise the integrity of machine learning models. Breaches can lead to unauthorized access to sensitive information, necessitating robust security measures. Companies must invest in cybersecurity frameworks to protect their datasets. A few strategies include:
- Implementing encryption technologies to safeguard data.
- Using anonymization techniques to minimize risks when handling personal information.
- Regularly conducting security audits to identify vulnerabilities.
- Ensuring a clear understanding of third-party data agreements.
Data security and privacy are not just compliance issues; they are fundamental to maintaining competitive advantage in the market. Stakeholders must be proactive in addressing these concerns to ensure sustainable growth in their machine learning initiatives.
Talent Shortage in the Industry
The success of machine learning initiatives depends significantly on the availability of skilled personnel. A prominent challenge is the shortage of experts in data science and machine learning. This is troubling for organizations seeking to harness the power of machine learning technologies. Companies are often forced to compete fiercely for talent in a limited pool of qualified candidates. The complexities of machine learning require specialization and expertise, which many existing educational programs have yet to fully address.
To combat the talent shortage, businesses can take several measures:
- Investing in training programs to upskill current employees in machine learning techniques.
- Collaborating with universities and vocational schools to create tailored educational programs focused on practical machine learning applications.
- Offering attractive incentives and flexible work arrangements to attract top talent.
- Building a culture of continuous learning and innovation to engage existing staff.
The challenge of talent acquisition is crucial, as high-caliber teams are necessary to drive successful machine learning projects. Addressing this gap will require commitment and strategic action from businesses.
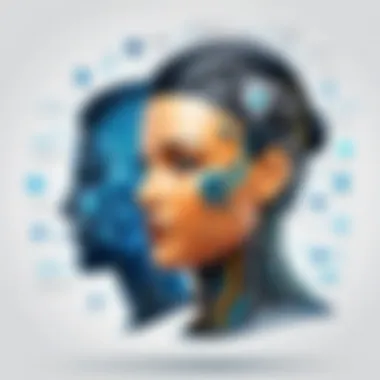
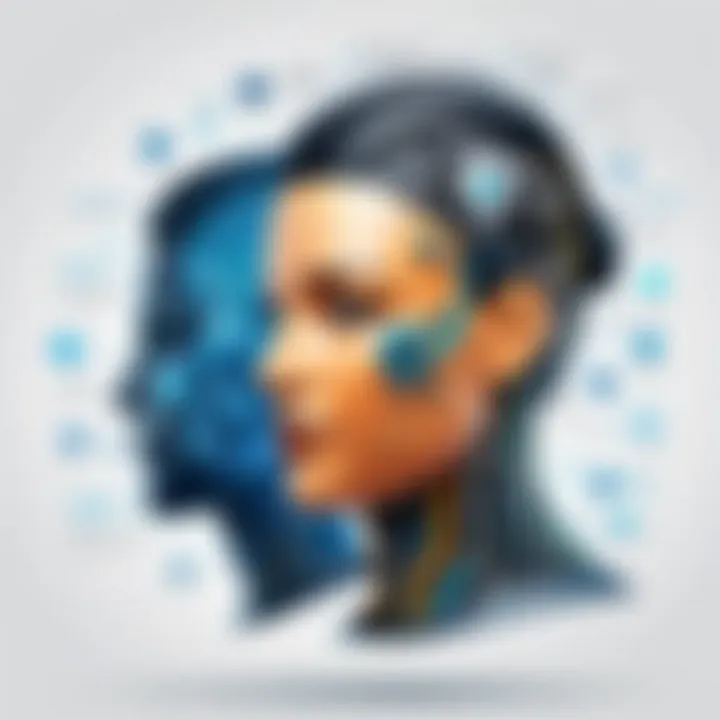
"Machine learning is only as powerful as the people behind it. Without the right talent, the technology cannot reach its full potential."
In summary, challenges like data privacy concerns and talent shortages present significant barriers in the machine learning market. Organizations must prioritize addressing these issues to thrive in an increasingly competitive space.
Future Outlook for Machine Learning
The future outlook for machine learning is pivotal in understanding how organizations can harness technology to remain competitive. As markets evolve, the insights garnered from machine learning analyses become essential for strategic planning. Businesses that lead in this technology can enhance their efficiency while gaining a substantial advantage over their competitors. Here are some critical elements that define this future outlook:
- Innovation Growth: Machine learning is expected to continue its rapid evolution, with innovations frequently changing the landscape of all industries. New algorithms and improved computing power will lead to better performance and more capabilities.
- Integration Across Sectors: The adoption of machine learning will not be confined to tech companies. Industries like healthcare, finance, and retail are increasingly incorporating AI solutions into their operations. This broadening scope signifies a collective transformation in how companies operate.
- Regulatory Landscape: As machine learning grows, so does the scrutiny placed upon it. Policymakers are developing guidelines to ensure ethical use. Organizations must prepare for these shifts which may influence their strategies regarding the deployment of machine learning solutions.
Understanding these elements helps businesses to align their objectives accordingly.
Emerging Trends
Several emerging trends in machine learning indicate the directions in which the market is headed:
- Explainable AI: As machine learning models identify complex patterns, the need for transparency becomes vital. Explainable AI will enable users to understand model decisions, helping to build trust amongst stakeholders.
- Edge Computing: This trend seeks to reduce latency by processing data closer to where it is generated. Companies are expected to leverage this to enhance performance for real-time applications.
- AutoML: Automated Machine Learning seeks to simplify model development, enabling non-experts to create machine learning models with more ease.
Adapting to these trends will be crucial for companies aiming to maintain relevancy.
Predicted Market Evolution
The evolving market for machine learning is characterized by numerous anticipated changes:
- Projected Growth Rates: Market analysts expect substantial growth rates in the coming years, with estimates pointing to a market size reaching hundreds of billions of dollars.
- Investment Surge: Venture capital firms are increasingly eyeing machine learning startups, hinting at a trend where more funding will flow into this sector. The rise of investment will boost growth and innovation.
- Diverse Applications: The versatility of machine learning poses significant potential for application across diverse sectors. This diversity foreshadows vibrant market dynamics that will benefit businesses leveraging these technologies.
Machine learning is poised for remarkable growth, affecting various sectors similarly, which necessitates a keen understanding of its trajectory to capitalize on the opportunities ahead.
Implications for Businesses
The implications of machine learning for businesses are profound and multi-faceted. As explored in this article, organizations must recognize that the adoption of machine learning technologies is not merely a trend but a strategic necessity. This sector is growing rapidly, and failure to adopt these advanced technologies could result in lost competitive advantage.
Adopting machine learning facilitates enhanced decision-making processes. Companies can analyze vast amounts of data to derive actionable insights. Utilizing algorithms helps identify patterns and predict outcomes more accurately than traditional methods. Such capabilities can improve operational efficiency and optimize resources.
Moreover, organizations benefit from personalized customer experiences. Machine learning can tailor services and products to individual needs, increasing customer satisfaction and loyalty.
Strategic Adoption of Machine Learning
For effective implementation, a strategic approach to machine learning must be taken. This involves several key steps:
- Assessment of Needs: Companies should begin with a detailed evaluation of their business objectives. Understanding specific areas where machine learning can add value is critical.
- Data Readiness: Before adopting these technologies, organizations must ensure they possess high-quality data. Clean, relevant data is essential for training machine learning algorithms.
- Infrastructure Setup: A robust infrastructure is necessary to support machine learning applications. This may include investing in cloud services or on-premises solutions, depending on the company's requirements.
- Skill Development: There is a pressing need for skilled professionals to manage and maintain machine learning systems. Organizations may consider training existing employees or hiring specialists.
- Project Management: Implementing machine learning projects require careful management. Using methodologies such as Agile can help in iteratively developing solutions and minimizing risks.
By prioritizing these elements in their strategy, organizations can effectively harness machine learning's potential, ensuring alignment with their broader business goals.
Investment Considerations
Investing in machine learning presents unique challenges and opportunities. Several factors influence the financial outlay that businesses might encounter:
- Initial Costs: The implementation of machine learning involves significant upfront costs. This includes software licensing, infrastructure setup, and potential hiring of data scientists.
- ROI Assessment: Companies must construct clear metrics to assess the return on investment from machine learning projects. Understanding short-term outlays versus long-term benefits is vital.
- Scalability: It's crucial to consider the scalability of machine learning solutions. Choosing flexible and adaptable technologies can allow organizations to grow without necessitating extensive reinvestment.
- Risk Management: Businesses should also be cognizant of risks associated with these investments. Data privacy concerns and regulatory compliance may impact both cost and strategy.
- Continuous Learning: Technology evolves, and so should investments in machine learning. Organizations should foster a culture of continuous evaluation of emerging technologies to stay competitive.
In summary, the implications for adopting machine learning extend beyond immediate benefits; they shape future business landscapes. By establishing strategic frameworks and carefully considering investment dynamics, organizations can unlock significant value from machine learning initiatives.
Finale
In this article, we examined the complexities surrounding the machine learning market size with valuable insights from Gartner's research. The significance of understanding this sector extends beyond mere statistics; it encompasses the strategic directions businesses must adopt to stay competitive in an evolving landscape. This final segment synthesizes the key findings and contextualizes their implications for future decisions.
Summary of Key Insights
The research highlighted various factors that influence the machine learning market:
- Market Growth: The substantial expansion in the machine learning sector, backed by technological advancements and increasing corporate investments.
- Investment Trends: Significant financial commitment from both private and public sectors toward artificial intelligence technologies.
- Regional Disparities: Distinct market dynamics across different global regions, emphasizing the need for localized strategies.
- Industry Application: Diverse applications in sectors such as healthcare, finance, and retail demonstrate the technology's versatility and relevance.
These insights reveal a market that is not only robust but also layered with opportunities and challenges. Organizations must not only recognize these trends but also act upon them meticulously to harness their full potential.
Final Thoughts
The future of machine learning is promising yet complex. As companies navigate through this dynamic environment, understanding the market size and trends is crucial. Decision-makers need to remain aware of:
- Evolving Technology: Staying updated with the latest developments in machine learning tools is essential for sustaining competitive advantage.
- Strategic Partnerships: Forming alliances with tech innovators can deepen an organization's capabilities in deploying AI solutions effectively.
- Regulatory Considerations: Adherence to data privacy regulations will shape the successful implementation of machine learning initiatives.