Lead Scoring and Predictive Analytics in B2B Software
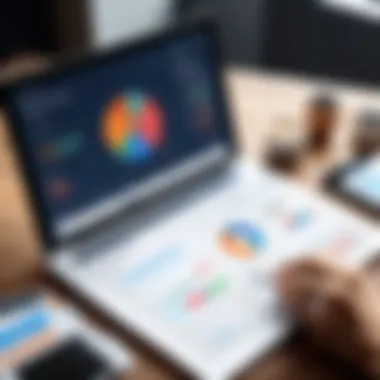
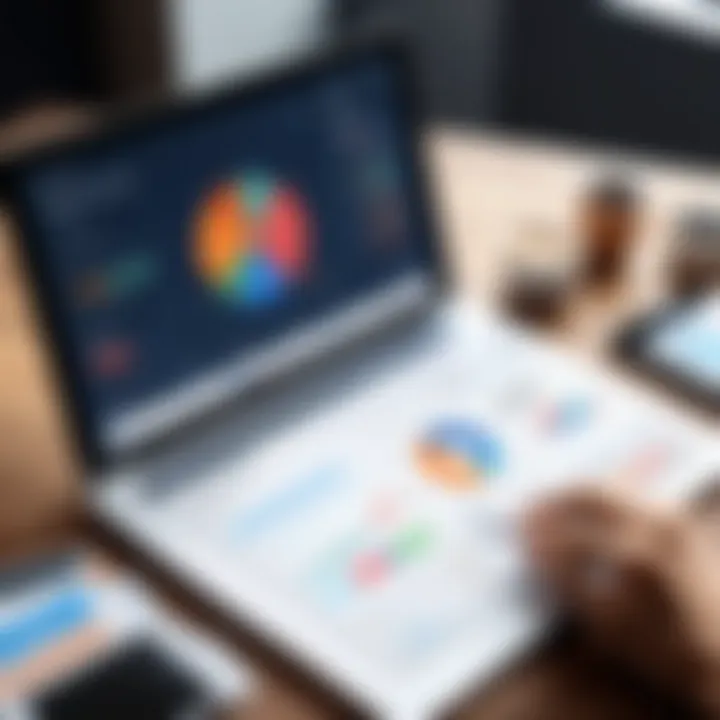
Intro
In the fast-paced world of B2B software acquisition, lead scoring and predictive analytics have emerged as key tools that organizations employ to enhance their decision-making processes. Understanding the intersection of these methodologies is crucial. As businesses strive to make smarter investments, the ability to identify high-value leads and predict their potential can make all the difference. This article will bring clarity to how lead scoring paired with predictive analytics shapes effective software purchasing strategies.
One does not need to look too far to find examples of how these concepts play out in real-world scenarios. Companies like HubSpot have leveraged data-driven insights to refine their sales processes, showing that effective scoring can illuminate paths that mere intuition might leave in the dark. The fusion of lead scoring with predictive analytics enables businesses to engage with prospects that are more likely to convert, thereby optimizing both resources and time. However, it’s not without its hurdles.
This exploration will offer actionable insights aimed at decision-makers and IT professionals who are keen on refining their software acquisition processes. Let’s dive deeper into the fundamental components of lead scoring and predictive analytics and how they work hand in hand to drive success in the competitive landscape of B2B solutions.
Understanding Lead Scoring
Lead scoring plays a pivotal role in the landscape of B2B software decisions, primarily because it enables organizations to sift through various leads and identify those that hold the most promise. By methodically evaluating potential clients based on specific criteria, companies can allocate their resources more effectively, maximizing their chances of closing deals. Whether a business is seeking to boost sales, refine its marketing approach, or enhance customer relationships, understanding lead scoring is essential. This practice streamlines efforts and allows teams to focus on leads that are more likely to convert, ultimately driving growth.
Definition and Importance
Lead scoring refers to the process of assigning values to prospects based on their characteristics and behavior. Essentially, it acts like a compass, guiding sales and marketing teams towards the leads with the highest potential for conversion. The importance cannot be overstated; when done correctly, it saves precious time and energy, transforming the way teams interact with prospects. In addition, focusing on quality leads rather than quantity enhances both efficiency and effectiveness in the sales process.
Components of Effective Lead Scoring
Demographics
Demographics are fundamental in the lead scoring process because they provide at-a-glance insights into who the prospects are. Factors like age, job title, industry, and company size matter significantly. For instance, targeting IT managers in mid-sized companies can lead to higher conversion rates than a broader approach. The key characteristic here is that demographics offer a clear picture of the target audience, making it a popular choice for this article. However, it’s worth noting that relying solely on demographics can sometimes lead to a skewed understanding of a lead's potential, as not all valuable leads fit prescribed molds.
Behavioral data
Behavioral data adds a layer of nuance to lead scoring by showing how prospects interact with the company's online platforms or marketing materials. For example, someone who opens emails repeatedly or engages with product demos on a website signals genuine interest. This type of data is popular because it reflects real-time user engagement, painting a picture of how likely a lead is to convert. A unique feature of behavioral data is its responsive nature; as it evolves, so too can the lead's score. However, a potential disadvantage is that it requires continuous monitoring and analysis, which can be resource-intensive.
Engagement levels
Engagement levels can reveal how invested a lead is in your offerings. For instance, a repeat visitor to your website or a participant in webinars suggests a strong interest. The key attribute here is that engagement levels tap into a lead's enthusiasm, which can be a game-changer in prioritizing outreach. It's beneficial in this article as it aligns with the goal of nurturing leads. One downside, though, is that not all engaged leads may be ready to convert. Some may just be gathering information, so a delicate balance is required.
Buying intent
Buying intent is arguably the most critical scoring component, as it directly relates to the chance of a lead making a purchase. Indicators of intent include requests for quotes, downloading product brochures, or engaging in product-specific discussions. The significant characteristic that makes buying intent a popular choice for this article is its predictive value—it gives strong signals about where a lead is in the buying process. On the flip side, focusing too much on buying intent can lead to missing out on nurturing relationships with leads that may need more time before deciding.
Traditional Approaches to Lead Scoring
Manual scoring methods
Manual scoring methods involve team members assessing leads based on established criteria. While this can be personalized, it often leads to inconsistencies due to subjective judgments. This key characteristic makes it a less popular choice for modern B2B operations, as it can turn the process into a guessing game on who the best leads are. A unique feature is that manual methods can be great in smaller operations where personalized touch matters; however, they become cumbersome with larger lead volumes and may result in biases that skew the scoring.
Rule-based systems
Rule-based systems use a set of predefined criteria to automate the lead scoring process. This approach allows for a more systematic evaluation of leads based on established rules, such as granting points for specific actions. A key benefit of this approach is consistency; once rules are in place, the scoring becomes uniform across all leads. However, a notable disadvantage is that rule-based systems can be rigid. They may not adapt to changes in leads’ behavior or market dynamics as swiftly as more flexible systems, limiting their effectiveness.
"Implementing effective lead scoring models lies at the heart of optimizing B2B software decisions. By harnessing distinct yet interrelated components, businesses can not only streamline their sales efforts but also improve their pipeline management significantly."
The Role of Predictive Analytics
Predictive analytics holds a pivotal position in optimizing lead scoring, especially in the realm of B2B software decisions. By providing data-driven insights, it transforms how businesses prioritize their leads, enhancing the efficiency and effectiveness of their marketing and sales efforts. Focusing on patterns and behaviors exhibited by past leads, predictive analytics helps in forecasting future buying behaviors and customer journeys. This leads to better alignment between marketing strategies and customer needs, ensuring that businesses can act swiftly and intelligently in a competitive landscape.
Defining Predictive Analytics
Predictive analytics can be defined as the branch of advanced analytics that uses both historical and current data combined with machine learning and statistical techniques to make predictions about future events. In simple terms, it’s all about analyzing data to identify trends that might otherwise go unnoticed in traditional analytics. This proactive approach enables organizations to not just react to changes but to anticipate them, leading to more strategic decision-making.
Key Techniques in Predictive Analytics
Statistical modeling
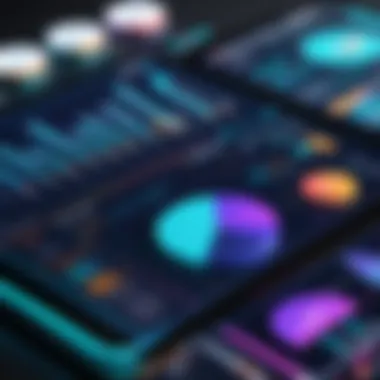
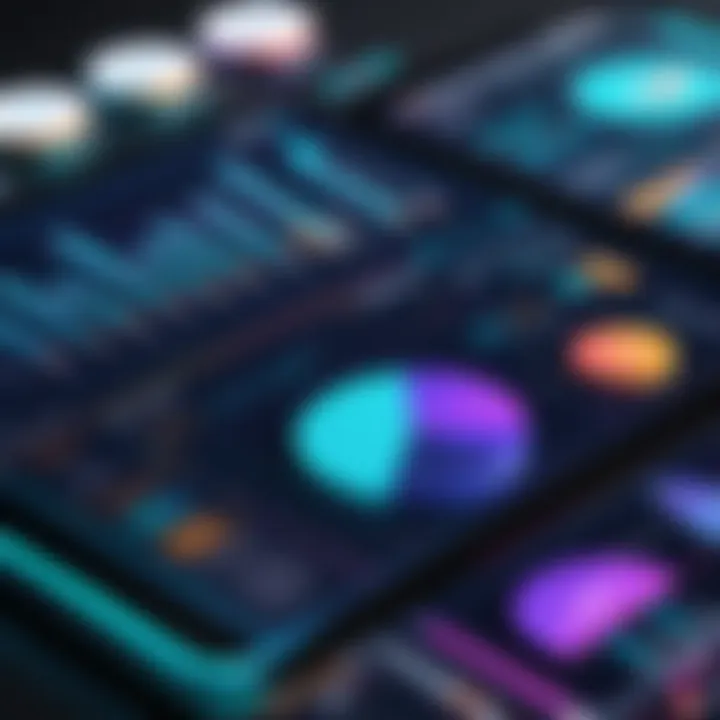
Statistical modeling is a fundamental technique within predictive analytics. It involves applying mathematical models to assess the relationships between various variables. This method is particularly beneficial for identifying correlations between lead behaviors and their likelihood of conversion. The key characteristic of statistical modeling is its ability to simplify complex datasets into understandable frameworks, which allows decision-makers to make informed choices backed by empirical evidence.
One unique feature of statistical modeling is its capability to produce actionable insights from data. By highlighting significant predictors, it guides businesses in prioritizing leads with the highest potential based on specific characteristics. However, one must be cautious of its limitations, as reliance on historical data might not always predict future scenarios accurately, particularly in rapidly changing markets.
Machine learning
Machine learning is another essential aspect of predictive analytics that has gained popularity in recent years. This approach utilizes algorithms that learn from data patterns to improve over time without explicit programming. What sets machine learning apart is its adaptability; as more data becomes available, the models refine their predictions, ensuring they stay relevant.
Its unique feature lies in its capability to process large volumes of data much faster than statistical modeling. This allows companies to analyze vast datasets in real-time, identifying emerging trends and shifts in customer behaviors. However, a downside could be the complexity of these algorithms, which sometimes makes it hard for decision-makers to understand how conclusions are drawn—one might even say it's a case of 'black box' analytics where clarity can be compromised.
Predictive Analytics vs Traditional Analytics
While traditional analytics typically focuses on what has happened, predictive analytics takes a step further to estimate what is likely to happen in the future. Traditional analytics relies heavily on historical data to produce reports, whereas predictive analytics combines predictive modeling with the ability to analyze data trends, automate data collection, and optimize lead scoring.
The benefits of predictive analytics become clear when you consider the agility it provides to businesses. Instead of merely reacting to trends, companies can start to cultivate their paths through informed strategy modifications that anticipate market changes.
Predictive analytics changes the game by allowing businesses to move from a reactive stance to a proactive approach; it’s not just about surviving anymore, but thriving.
With predictive analytics, B2B companies can not only enhance their lead scoring but also create a more robust framework for understanding their customer base, ensuring they are always one step ahead in the competitive landscape.
Integrating Predictive Analytics with Lead Scoring
Integrating predictive analytics with lead scoring represents a pivotal step for businesses looking to sharpen their strategies in the B2B software market. This fusion not only enhances the accuracy of scoring models but also provides a more nuanced understanding of potential customers. When organizations leverage predictive analytics in combination with lead scoring methods, they can streamline their decision-making process, allocate resources more efficiently, and ultimately drive sales effectiveness.
Building a Data-Driven Scoring Model
Data collection methods
Data collection methods are foundational to forming a robust lead scoring model. By gathering data systematically from various touchpoints, organizations can better track and understand customer interactions. Potential sources include CRM systems, web forms, and even social media interactions. The key aspect of these collection methods is that they help paint a comprehensive picture of the leads.
A popular choice among businesses is implementing automated systems that can continuously pull data from these sources. This ensures that the information is always up-to-date and accurate. One unique feature of using automated data collection methods is their capacity for real-time feedback, which enables sales teams to react swiftly to changes in lead behavior. However, there are disadvantages; it may lead to an overwhelming amount of data, necessitating effective filtering and analysis to ensure valuable insights.
Feature selection
Feature selection plays a crucial role in refining lead scoring models. This process involves identifying which variables are important from the vast pool of collected data. Organizations typically prioritize characteristics that correlate with purchasing decisions and customer engagement. A key characteristic of effective feature selection is its focus on reducing noise—eliminating the less relevant data points to hone in on what truly matters for scoring.
The assessment of features can be done through various techniques, including statistical tests, correlation analysis, and machine learning methods that help in ranking features based on their impact. One unique feature here is the ability to combine quantitative and qualitative data, allowing models to capture nuanced perspectives. Yet, the disadvantage of an inappropriate selection process can lead to models that are either too simplistic or overly complex, diminishing their predictive power.
Enhancing Accuracy of Leads
Accuracy in lead scoring is not just about acquiring data and selecting features. It's about continuous enhancement of models based on feedback and ongoing analysis. Predictive analytics can significantly boost the likelihood of identifying genuinely worthwhile leads. By regularly analyzing past scoring effectiveness and updating models to reflect new insights, businesses will find that the potential for conversion increases substantially.
Moreover, a focus on refining algorithms can help identify patterns that might go unnoticed with a more traditional approach. This results in a more intelligent scoring model that can adapt and evolve as market conditions change. Ultimately, the integration of predictive analytics with lead scoring fosters an environment where businesses can prioritize their efforts and resources more strategically, aligning themselves closer to their target audience.
"Predictive analytics is the key to transforming vast data into actionable insights, making every lead count."
Integrating these two methodologies not only helps in optimizing B2B software decisions, but it also sets the stage for success in an increasingly competitive landscape.
Practical Applications in B2B Software Decisions
The intersection of lead scoring and predictive analytics carries immense weight in the realm of B2B software acquisition. As technology evolves, so do buyer preferences and expectations, which can be as fickle as the wind. A solid understanding of practical applications in this area becomes vital for organizations looking to not just keep up but excel in their market. Insightful use of these methodologies aids companies in making informed decisions, thereby optimizing their software purchases and achieving better overall alignment with their strategic goals.
One of the most appealing benefits of integrating lead scoring with predictive analytics is the ability to prioritize potential leads effectively. Companies can allocate their resources more judiciously by identifying which leads warrant immediate attention and which can be nurtured at a later date. This prioritization means sales teams can engage with prospects who are not just interested but also likely to convert. Consequently, it leads to higher conversion rates and, ultimately, a more robust bottom line.
Factors to consider when piecing together these practical applications include:
- Data Quality: The accuracy and reliability of data directly affect the effectiveness of lead scoring. Clean, high-quality data ensures that predictive models yield meaningful insights.
- Customization: Tailoring scoring models to fit individual business needs ensures relevant metrics are prioritized. Different industries may require nuanced approaches, and a cookie-cutter solution can fall short.
- Feedback Loops: Incorporating user feedback and sales data continually refines the scoring models. This iterative process helps in adapting to uncharted waters as buyer behavior changes.
"Effective lead scoring is not a ‘set it and forget it’ tactic. Instead, it is a living strategy that should evolve alongside your business and its prospects."

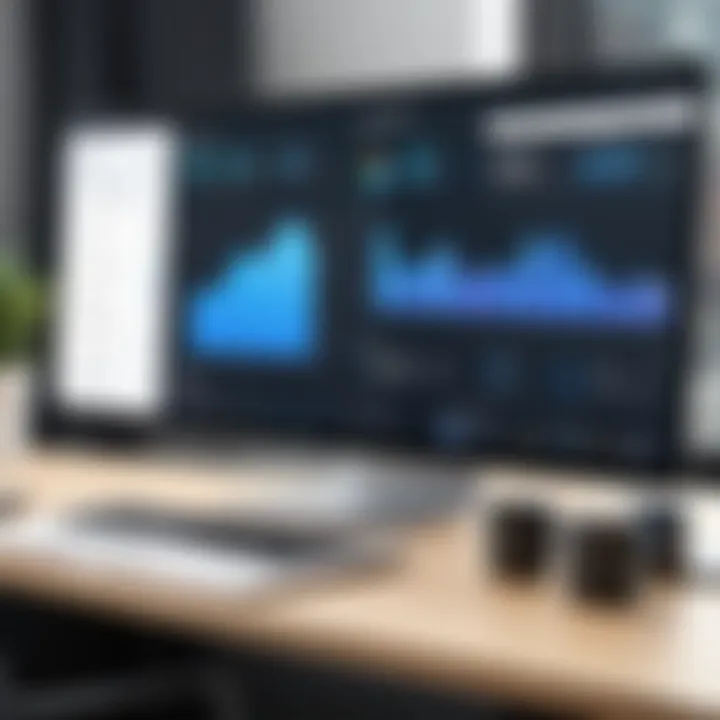
Lead Prioritization Strategies
In the competition to secure sales, focusing on the right leads can make all the difference. Lead prioritization strategies form the cornerstone of a successful approach. Simply put, knowing which leads are hot and which are lukewarm can direct efforts where they matter most.
Some key strategies for prioritization might include:
- Scoring Matrix: A visual tool that juxtaposes different metrics like engagement, demographic fit, and purchase intent helps in making data-driven decisions rapidly.
- Behavioral Tracking: Monitoring how potential leads interact with emails, webinars, or content guides adjustments to their scores based on real-time data.
- Automation Tools: Utilizing platforms such as HubSpot or Salesforce can further streamline the lead prioritization process, ensuring that the most promising leads rise quickly to the top of the funnel.
The essence of these strategies lies in their ability to transform data into actionable insights that resonate with the sales team. This clarity fosters a more strategic approach, enabling teams to work smarter, not harder.
Nurturing High-Scoring Leads
Nurturing high-scoring leads is akin to tending a garden; it requires attention and care. Once leads are identified and prioritized, the next step is to engage them effectively. High-scoring leads have shown interest, but it doesn't stop there. They need further engagement to convert them into customers.
To nurture these leads successfully, many organizations have adopted the following techniques:
- Personalized Communication: Tailored messages that resonate with specific pain points show leads that the organization understands their struggles and has solutions in hand. This personal touch can significantly enhance the nurturing process.
- Content Marketing: Providing valuable content such as webinars, whitepapers, or case studies relevant to the lead’s industry ensures ongoing engagement. It helps establish trust and position the organization as a thought leader.
- Automated Follow-ups: Setting up automated follow-ups keeps the conversation going, ensuring leads don't go cold. Well-timed reminders or targeted offers based on lead behaviors can keep your brand top-of-mind.
Every interaction counts. Nurturing isn't merely about selling; it's about building a relationship that fosters loyalty and trust. When leads feel valued and understood, they are more likely to make the leap from interest to purchase.
Best Practices for Implementing Lead Scoring Models
When diving into the intricate waters of lead scoring, understanding best practices becomes paramount. Implementing lead scoring models isn’t just a checkbox on your to-do list; it’s a strategic endeavor that can make or break your B2B software acqisition process. With the right practices in place, organizations can fine-tune their approach, maximize resources, and ultimately foster business growth.
Defining Clear Objectives
A crucial first step in any strategy is clarity of vision. Defining clear objectives for your lead scoring model isn’t merely a formality; it’s the foundation upon which everything else rests. What are you hoping to achieve? Whether it's improving lead conversion rates, reducing customer acquisition costs, or enhancing marketing and sales alignment, objectives must be specific and measurable.
Without the "why" clearly laid out, teams may find themselves floundering, unsure of their direction. Here are some key considerations when defining your objectives:
- Aligning with Business Goals: Any lead scoring initiative should mirror the broader objectives of the organization. If the main goal is revenue growth, calibrate your scoring to prioritize leads that have the highest potential to convert.
- Stakeholder Involvement: Engage different departments to get a holistic view. Sales and marketing might have unique insights into what constitutes a high-quality lead.
- Measurable KPIs: Instead of vague goals like "generate more leads," opt for something like "increase lead conversion rate by 15% over the next quarter."
By establishing clear, pragmatic objectives, teams can ensure that their lead scoring efforts remain focused and effective, keeping everyone on the same page.
Ongoing Model Evaluation
Setting up a lead scoring model isn't a "set it and forget it" venture. Like any machine, it requires regular maintenance and evaluation to ensure it’s running smoothly and yielding favorable outcomes. Ongoing model evaluation helps organizations adapt and refine their approach as market dynamics and business needs change. Here are some aspects to consider:
- Regular Review Cycles: Schedule periodic evaluations—quarterly or bi-annually—to assess effectiveness. This will allow you to adjust your lead scoring criteria based on new data or unexpected changes in consumer behavior.
- Feedback Loops: Create mechanisms for gathering feedback from sales teams. Their frontline insights can be invaluable in informing future iterations of the scoring model.
- Data Monitoring: Continuously track data quality. Outdated or irrelevant information can skew scoring models significantly. Keeping your database fresh ensures that the scoring remains relevant.
Regular evaluation of your model’s performance can provide insights to refine processes and better allocate resources.
- Assessing Model Accuracy: Use A/B testing to determine if adjustments to your scoring model truly improve lead quality. Implementing changes without measuring their impact can lead to missteps.
By making ongoing evaluation part of the lead scoring strategy, organizations can pivot when necessary and remain competitive. The landscape is ever-changing, and responsiveness is key.
Challenges in Lead Scoring and Predictive Analytics
In the ever-evolving landscape of B2B software acquisition, accurately scoring leads and making predictive analyses can feel like trying to hit a moving target. While organizations strive to harness the power of data for smarter decision-making, they often encounter significant roadblocks. Addressing these challenges is not merely a technical issue; it’s a strategic necessity that can dramatically influence the effectiveness of marketing efforts and sales strategies. If businesses are to optimize their lead scoring and predictive analytics, they must unravel common pitfalls that can critically compromise their initiatives.
Data Quality Issues
The adage, "garbage in, garbage out," rings profoundly true in the realm of data analytics. When it comes to lead scoring, the integrity of data is paramount. Poor-quality data can stem from various sources: outdated contact lists, erroneous entries, or even inconsistent formatting. Each of these problems can skew the overall score that a lead receives, leading to missed opportunities or, conversely, misplaced focus.
- Identification of quality data: One critical aspect of ensuring high-quality data involves identifying and filtering out duplicates in databases. Regular audits can help clean existing leads.
- Data enrichment: This may involve updating lead profiles through third-party services or direct outreach, ensuring that the information is accurate and current.
Moreover, data gathered from disparate systems often lack cohesion, complicating efforts to create a unified view of potential customers. Having robust processes in place for data validation is one of the key measures organizations can adopt to mitigate quality issues.
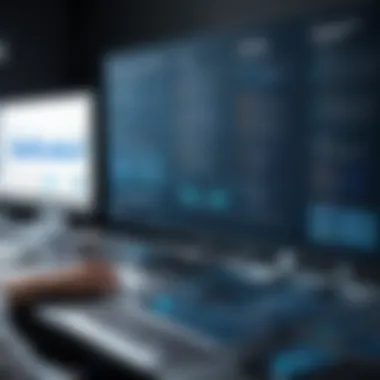
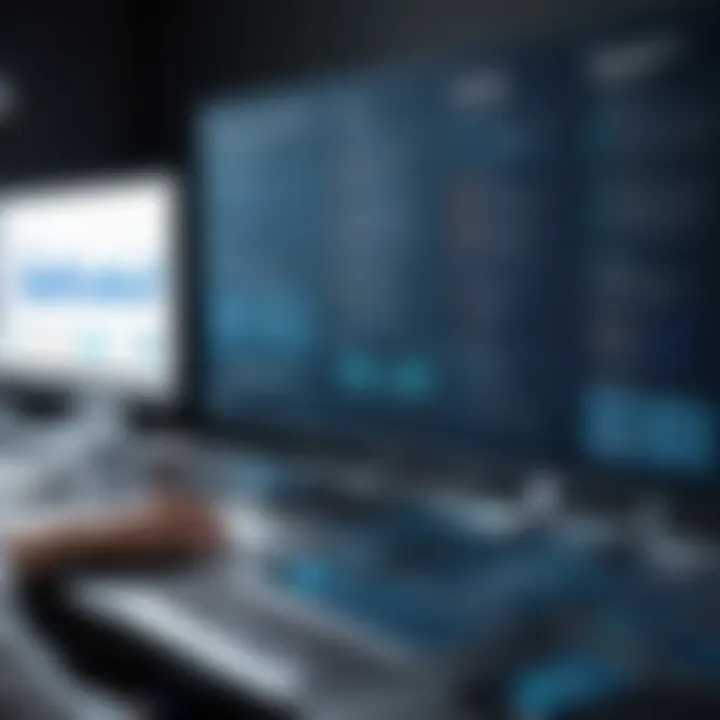
Overfitting in Predictive Models
Another significant challenge that arises in predictive analytics is the phenomenon of overfitting. When predictive models are too complex, they can start to reflect the noise in the data instead of just the underlying patterns. This leads to models that perform exceedingly well on training data but poorly in real-world scenarios. The balance between complexity and simplicity in model design is akin to walking a tightrope.
- Choosing the right model: Businesses must be wary of opting for overly sophisticated algorithms without a clear understanding of their data. Sometimes a simple model can produce equally valuable insights with greater generalizability.
- Cross-validation: Employing techniques such as cross-validation can help in testing how a model performs with different subsets of data, ensuring that it doesn't just memorize the training data.
Overfitting can masquerade as accuracy but may ultimately lead decision-makers astray, creating illusions of success based on unreliable insights.
Future Trends in Lead Scoring and Predictive Analytics
In the ever-evolving landscape of B2B software acquisition, understanding future trends in lead scoring and predictive analytics is crucial. Organizations that adapt persistently to new technologies and methodologies will fare better in identifying high-value leads. Leveraging insights and trends can provide a competitive edge, allowing companies to hone their strategies, better predict customer intent, and optimize resources, which ultimately drives revenue growth.
Emerging Technologies
Artificial Intelligence
Artifical Intelligence (AI) has emerged as a cornerstone in modern lead scoring and predictive analytics frameworks. Its ability to analyze vast amounts of data at lightning speed allows businesses to identify patterns that human analysts might overlook. The key characteristic of AI is its capability to learn and adapt over time, which enables continuous improvement in the accuracy of lead predictions. For instance, AI can sift through user behavior data more efficiently, uncovering insights about customer preferences and buying habits without the need for constant human intervention.
One unique feature of AI is its ability to utilize predictive modeling techniques to forecast future behaviors based on historical data. This advantage can help organizations prioritize leads that are more likely to convert, thus optimizing marketing efforts and increasing sales efficiency. AI does come with its downsides, though; it requires substantial commitment in terms of data quality and processing power. However, when implemented effectively, it stands out as an optimal choice for organizations keen on long-term growth.
Natural Language Processing
Natural Language Processing (NLP) also plays a significant role in refining lead scoring and predictive analytics. By analyzing textual data, NLP offers businesses the instrument to understand customer sentiment more comprehensively. The key characteristic is its focus on language comprehension, allowing organizations to interpret feedback, reviews, and engagement patterns effectively. NLP can be instrumental in gauging leads’ emotions and attitudes towards products or services, enhancing overall understanding of client needs.
A unique feature of NLP is its ability to extract key themes and sentiments from unstructured data, such as emails or social media interactions, helping businesses to nurture relationships with potential clients. While NLP tools can sometimes struggle with nuances in language, their evolving capabilities continue to greatly benefit organizations aiming for personalized engagement strategies. This can lead to improved responses and a higher likelihood of conversion.
Adapting to Changing Market Dynamics
As market conditions shift rapidly due to technological advancements and changing consumer preferences, businesses must become agile in their methodologies. Adapting to these changes means regularly evaluating lead scoring models to ensure they remain relevant. Organizations need to foster a culture of pragmatic decision-making that is grounded in real-time data.
By listening to customer needs and tracking market trends, companies can dynamically adjust their strategies for lead scoring and predictive analytics. Such agility aids in recognizing new opportunities and potential threats, allowing businesses to maintain their competitive edge. Ultimately, being attuned to shifts in the market landscape effectively enriches the lead scoring processes and predicts future business opportunities.
The End and Recommendations
The conclusion of this article serves as a vital summation of how lead scoring and predictive analytics can significantly enhance the decision-making process in B2B software acquisition. It wraps up the discussion by providing clarity on the core benefits these methodologies bring to the table, as well as the considerations necessary for a successful implementation.
In the fast-paced realm of B2B software decisions, where the stakes can be quite high, businesses can’t afford to make hasty judgments. Lead scoring, when augmented with predictive analytics, allows organizations to sift through potential leads in a more strategic manner. The combination creates a dynamic model that better reflects not just who is interested, but who is most likely to convert. This is where the real power lies: optimizing resources effectively.
Benefits of integrating lead scoring and predictive analytics include:
- Enhanced targeting: By understanding which leads hold the most promise, businesses can focus their marketing efforts more precisely.
- Increased efficiency: Marketing and sales teams can allocate their time and resources to leads that matter, thus improving conversions.
- Better alignment: Sales and marketing can work more closely together when they have shared insights from data-driven models.
However, some considerations demand attention:
- Data integrity: Ensuring the data feeding into these models is accurate and up-to-date is crucial.
- Ongoing adjustments: Markets and consumer behaviors shift, necessitating regular updates to scoring models to remain relevant.
Although challenges exist, making use of these practices allows businesses to not just stay afloat but thrive in a competitive landscape.
"The key to success lies in tailoring not just your strategies, but also your data analytics to the ever-changing tides of the market."
Summary of Key Insights
As explored, the integration of lead scoring and predictive analytics offers formidable advantages for B2B software acquisition. Key insights gathered throughout the article include:
- Lead scoring helps prioritize leads based on various factors such as demographics, past behavior, and intent to buy, ensuring that sales efforts focus on high-potential candidates.
- Predictive analytics leverages data to forecast outcomes, enabling businesses to make proactive decisions rather than reactive ones.
- The collaboration of these two methodologies results in a more holistic understanding of the customer's journey, breaking down silos between departments.
- It's imperative to remember that while technology can provide insights, human judgment still plays a crucial role in interpreting and acting on these insights effectively.
Next Steps for Implementation
To maximize the benefits of lead scoring and predictive analytics, organizations should consider the following steps for effective implementation:
- Gather and Clean Data: Begin by ensuring you have access to high-quality data. This ensures accuracy in your scoring model.
- Define Objectives Clearly: Establish what specific outcomes or KPIs your scoring model should drive towards.
- Choose the Right Tools: Research and select analytics and lead scoring tools that match your business needs. Tools like Salesforce or HubSpot could be beneficial partners in this journey.
- Pilot and Refine: Start with a pilot program to test your approach. Monitor performance and make iterative changes based on findings.
- Train Teams: Equip your teams with the knowledge they need to understand the power of these tools and how to apply them in daily tasks.
- Review and Update Regularly: Schedule regular reviews of your lead scoring model and predictive analytics performance to adapt to changing market conditions or business goals.
Taking these steps will not only streamline the process of defining and optimizing lead scoring systems but also foster a culture of data-driven decision-making.
By focusing on these recommendations, organizations will set the stage for a more refined and successful B2B software acquisition process.