Comprehensive Insights into Risk Modelling Software
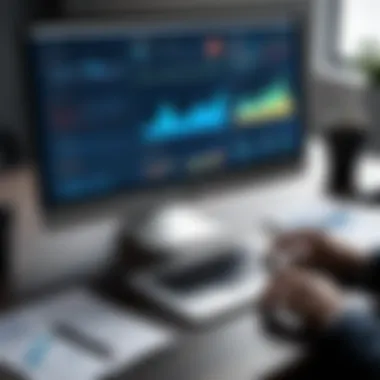
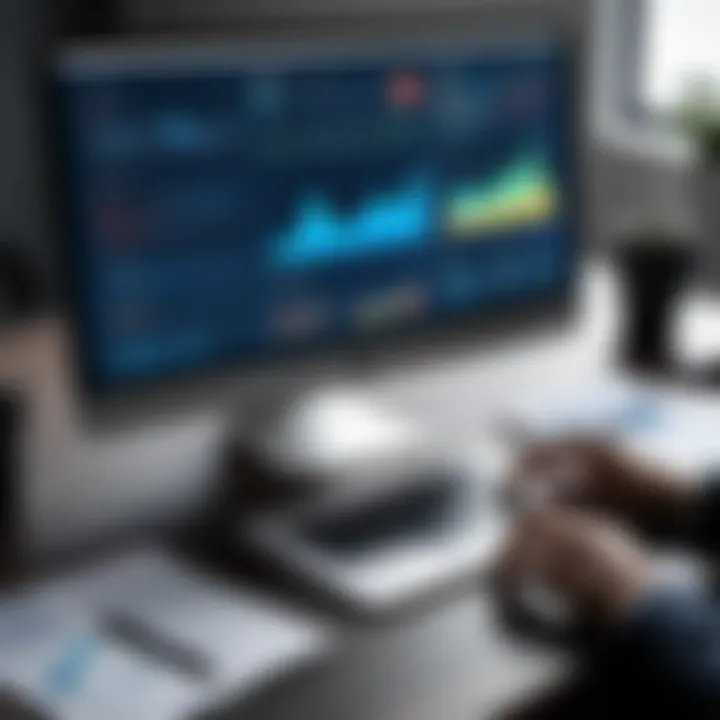
Intro
In today’s fast-paced business landscape, where uncertainty lurks behind every corner, organizations increasingly rely on sophisticated tools to mitigate potential losses. Risk modelling software stands out as an essential player in this arena, guiding businesses through the murky waters of decision-making. But what exactly is risk modelling software, and how can it transform the way businesses operate? This article delves into the intricacies of such software, providing insights into its features, applications, and the current trends shaping the industry.
Key Features
Overview of Core Features
Risk modelling software comes packed with a range of functionalities tailored to address specific needs. Some of the key features most often highlighted include:
- Data Analysis: The heart of any risk modelling tool lies in its ability to process vast amounts of data. Advanced algorithms analyze historical data to predict future outcomes, allowing businesses to make data-driven decisions.
- Scenario Analysis: Users can simulate different scenarios to see how various factors affect risk. This capability is crucial for businesses looking to understand potential challenges before they arise.
- Reporting Tools: Clear and actionable insights are necessary for decision-making. Risk modelling software often provides customizable reports, making it easy to visualize data and communicate findings.
- Integration with Existing Systems: A seamless flow of information is vital. Modern risk modelling solutions offer robust integration capabilities with ERP systems, CRMs, and other software, enhancing efficiency.
User Interface and Experience
The user interface can make or break the effectiveness of risk modelling software. A well-designed interface simplifies navigation and empowers users to leverage its full potential without extensive training. Aspects that contribute to a positive experience encompass:
- Intuitive Dashboards: Clear, user-friendly dashboards that display critical metrics at a glance facilitate quicker decision-making.
- Customizability: The ability to tailor the interface to suit specific roles and preferences allows users to focus on what matters most.
- Support and Resources: Access to tutorials, webinars, or a responsive support team can enhance the overall user experience, ensuring users are never left in the dark.
Pricing and Plans
Overview of Pricing Models
Navigating the pricing landscape of risk modelling software can be a daunting task. Most providers offer various pricing models:
- Subscription-Based: Often favored for its flexibility, this model charges users periodically (monthly or annually), allowing companies to scale their usage as needed.
- One-Time License Fee: Some organizations prefer a one-off payment, gaining lifetime access to the software. However, this may not include ongoing support or updates.
- Freemium Model: This offers basic features for free, with advanced functionalities available through paid plans. It's a great way for businesses to trial the software before committing.
Comparison of Different Plans
When selecting the right plan, it’s crucial to assess what you need. Here’s a basic comparison of fictional features from three different models:
| Plan Name | Core Features | Support | Price | | Basic | Data Analysis, Reporting | Email Support | $100/month | | Standard | Scenario Analysis, Custom Reports | Phone + Email | $250/month | | Premium | All Features | 24/7 Support | $500/month |
Properly weighing these options ensures alignment with your organization's unique needs.
"The key to managing risk is understanding it in all its forms and using that knowledge to make informed decisions."
In summary, risk modelling software is a powerful ally for organizations aiming to navigate uncertainties effectively. By diving into the features, pricing models, and user experience, decision-makers can make informed choices that fit their specific contexts, ultimately enhancing their strategic planning capabilities.
Understanding Risk Modelling Software
In today's fast-paced business environment, understanding risk modelling software is crucial for making informed decisions. This type of software provides organizations with tools to identify, analyze, and mitigate risks associated with various business activities. The importance of comprehending these systems can't be understated, especially as companies face increasingly complex challenges in their operations.
Definition and Purpose
Risk modelling software is designed to simulate and predict potential risks that businesses may encounter. Its primary purpose is to enable organizations to visualize the impact of different scenarios and make necessary adjustments before actual occurrences. Many businesses use this type of software to quantify risks, thereby allowing for proactive management strategies. In essence, risk modelling fosters a culture of foresight, promoting better business strategies and resource allocation.
Essential Features
When exploring risk modelling software, it’s essential to recognize some key features that enhance its functionality. This section delves into three critical elements: data analysis tools, risk assessment capabilities, and reporting functions.
Data Analysis Tools
Data analysis tools are at the heart of effective risk modelling software. These tools help in processing vast amounts of data, identifying trends, and drawing meaningful conclusions. A standout characteristic of data analysis tools is their ability to handle complex datasets, enabling businesses to visualize risks in real-time. For organizations looking to fine-tune their risk management processes, data analysis tools represent a foundational choice owing to their versatility and efficiency.
One unique feature of these tools is their integration with machine learning algorithms. It allows users to predict outcomes based on historical data. However, while powerful, one must approach these tools with caution; the reliance on past data can sometimes skew results if not monitored properly.
Risk Assessment Capabilities
Next, we look at risk assessment capabilities, which are fundamental to understanding risk levels within a business context. These capabilities assess potential threats and estimate their impact on operations. A primary benefit of risk assessment tools is their ability to categorize risks into various classes, which helps prioritize response actions.
A unique aspect here is the ability to conduct stress testing. This can expose vulnerabilities within a model, being quite crucial for businesses operating in volatile environments. However, potential disadvantages can arise due to the complex nature of these assessments. They demand a level of expertise that may not be readily available, sometimes leaving gaps in the analysis.
Reporting Functions
Finally, reporting functions play a vital role in risk modelling software by providing stakeholders with clear insights into potential risks. Through dashboards and summary reports, businesses can quickly disseminate critical information to decision-makers. A defining trait of effective reporting functions is their ability to present data in visually engaging formats, making it easier to grasp complex information.
One exceptional feature includes customizable report templates, allowing businesses to tailor information to specific audiences, whether they are executives or front-line managers. However, focusing too heavily on visuals may sometimes overshadow the quantitative analysis, so striking a balance is key.
"In risk management, understanding the tools at your disposal is just as vital as understanding the risks themselves."
In summary, understanding risk modelling software is pivotal for modern organizations aiming to navigate uncertainty. With effective data analysis, robust risk assessment capabilities, and insightful reporting functions, businesses can build a resilient framework for identifying and managing risks.
The Importance of Risk Modelling in Business
Risk modelling software has emerged as a critical component for businesses looking to navigate the often murky waters of decision-making. In today’s fast-paced environment, organizations cannot afford to operate on gut feelings alone. The complexities of financial markets, supply chains, and regulatory landscapes require a thorough understanding of potential risks.
By leveraging risk modelling software, companies can better assess various scenarios, ensuring they can make informed choices that align with their strategic goals. This not only fosters a culture of proactive risk management but also enhances overall organizational resilience.
Decision Making
In many cases, the difference between a successful outcome and a colossal misstep comes down to the decisions made at crucial junctions. Risk modelling software plays a vital role in ensuring these decisions are grounded in solid data rather than mere assumptions. For example, consider a company about to invest in a new product line. Without proper risk assessment, they might overlook potential market volatility that could affect their return on investment. A well-configured risk model would take historical data, market trends, and competitor actions into account, presenting a clearer picture for decision-makers.
Moreover, intuitive dashboards and visualizations can simplify complex data, allowing stakeholders to grasp key insights without needing a PhD in statistics. This accessibility fosters cross-departmental collaboration, as everyone can engage with the data without feeling overwhelmed.
Resource Allocation
When it comes to allocating resources, effective risk modelling brings clarity. Organizations often face competing demands for limited resources, which makes prioritization essential. By identifying areas of high risk and potential return, risk models enable businesses to allocate resources where they are most needed. This means a company can invest in divisions or initiatives poised for growth while minimizing exposure in riskier areas.
For example, a construction firm may utilize risk modelling software to analyze various projects’ potential risks, from site conditions to weather patterns. By determining which projects carry the least risk, the company can channel its resources effectively, ultimately boosting profitability and minimizing waste.
Regulatory Compliance
In a landscape where regulations can change faster than one can say "compliance," companies must stay one step ahead. Risk modelling software equips organizations with the tools necessary to navigate this unpredictability. It not only helps in identifying potential compliance risks but also ensures that the frameworks in place are robust and adaptable.
For instance, financial institutions often contend with strict compliance requirements that evolve based on economic conditions and regulatory updates. With risk modelling software, these institutions can assess their exposure to non-compliance and adjust their strategies accordingly.
"Adopting risk modelling solutions can significantly reduce the likelihood of regulatory fines and enhance overall operational efficiency."
By integrating risk management practices into their compliance regimes, organizations can foster a culture that prioritizes accountability while empowering teams to act quickly when facing unforeseen challenges.
Types of Risk Modelling Software
Understanding the different types of risk modelling software is crucial as it equips businesses with tools to navigate uncertainties effectively. Not all risks are quantifiable in the same way. Therefore, organizations often must cultivate an arsenal of varied models, each designed for specific risks and scenarios. This section dissects the three primary categories: quantitative models, qualitative models, and scenario analysis tools. Each of these types brings distinct benefits and considerations, which we'll explore in detail.
Quantitative Models
Quantitative risk modelling is rooted in numerical data and mathematical frameworks. These models aim to provide a clear picture through statistics and calculations. They can forecast potential losses based on historical data and statistical analysis. For instance, a financial institution might use quantitative risk models to analyze market volatility and determine potential impacts on investments.
The benefits are significant:
- Precision: The ability to calculate risk exposure in specific monetary terms.
- Predictability: Offers forecasts that can be tested for accuracy over time.
- Scalability: Effective for large datasets, allowing organizations to grasp extensive risk profiles.
However, challenges do arise. The complexity of the calculations can lead to over-reliance on models that may not translate well into real-world situations. Furthermore, if the data quality dips, the results can paint a misleading picture.
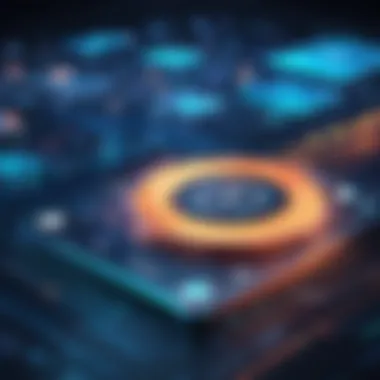
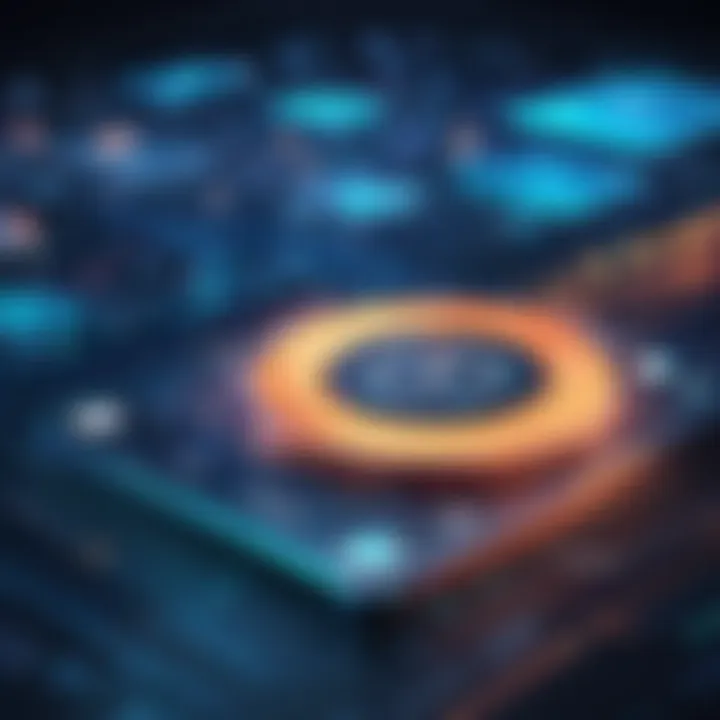
Qualitative Models
On the flip side, qualitative models focus less on numbers and more on the subjective evaluation of risks. They often involve expert judgment to assess risks based on various factors that can’t always be quantified. These include political stability, employee morale, or brand reputation. A company facing risks related to a merger might employ qualitative models to gauge employee sentiments or market perceptions.
Key aspects of qualitative models include:
- Contextual Evaluation: They capture nuances that quantitative models can miss.
- Flexibility: Since they don't rely heavily on numerical data, they can adapt quickly to changing environments.
- Rich Insights: Often provide deep insights into interpersonal dynamics within organizations.
However, their subjectivity can be a double-edged sword. Bias in judgment can distort findings, making it essential to balance these models with quantitative metrics.
Scenario Analysis Tools
Then we have scenario analysis tools, which allow organizations to imagine different future states. This type of software helps businesses prepare for various potential outcomes by simulating different risk scenarios. It uses both qualitative and quantitative data to predict how changes might affect operations or financial health. For example, a tech company might model scenarios ranging from a minor technology failure to a major data breach.
The advantages here are compelling:
- Comprehensive Planning: Encourages a broader view of risk and resilience in the face of unexpected challenges.
- Engagement: Often involves cross-department discussions, fostering collaboration.
- Decision-Making: Helps organizations make informed choices rather than reacting impulsively.
Nevertheless, the potential downsides include the time-consuming nature of creating these models and the risk of "analysis paralysis," where overthinking possibilities can prevent decisive action.
"A well-prepared organization does not only react to the present risks but also anticipates potential future challenges through effective modelling."
In summary, recognizing the right type of risk modelling software is not merely about selecting a tool; it’s about aligning the organization’s objectives, resources, and risk appetite with the most suitable modelling approach. Whether through quantitative models, qualitative assessments, or scenario analyses, the goal remains the same: to create a resilient framework capable of withstanding uncertainties in an ever-evolving business landscape.
Key Players in the Risk Modelling Software Market
Understanding the landscape of risk modelling software cannot be done without recognizing the pivotal role that leading companies and emerging technologies play. This market is characterized by a variety of players—some well-established, others shaking things up with fresh innovations. The significance of this section lies in grasping how these entities shape the software’s capabilities and drive advancements that ultimately affect end-users' outcomes.
Established Leaders
When it comes to risk modelling, a few names stand out, having earned their place as industry leaders over the decades. Companies like SAS, IBM, and Oracle have built robust reputations thanks to their comprehensive solutions, deep expertise, and continuous innovation. Their software platforms are often a safe choice, particularly for large enterprises that require proven functionality and reliability.
- SAS: Known for its advanced analytics, SAS offers a suite of tools that facilitate complex data analysis and risk assessments. Their strong emphasis on data security provides firms with peace of mind.
- IBM: IBM’s Watson has made waves in the industry by integrating machine learning into risk modelling, enhancing predictive analytics and data processing speeds. Their software can handle vast amounts of data, making it ideal for banking and finance sectors plagued with constant fluctuations.
- Oracle: With its cloud-based solutions, Oracle provides an adaptable environment for businesses of all sizes, allowing for custom solutions tailored to specific risks faced by different industries.
These organizations don’t just lead by numbers; they also usher in trends and standards that smaller software companies often need to follow. Their stability offers a familiar ground where businesses can determine how risk modelling can be tailored to their specific needs.
Emerging Technologies
In contrast, emerging technologies are breathing new life into the risk modelling field. Start-ups and smaller companies often introduce revolutionary ideas that established players may overlook. For example, platforms leveraging blockchain technology provide unparalleled transparency and traceability in risk assessments, appealing to sectors where trust and data integrity are non-negotiable.
Some noteworthy players include:
- QuantConnect: This open-source platform allows users to backtest algorithmic trading strategies in the cloud, introducing a new way for firms to assess financial risks.
- Riskified: Focusing on e-commerce, Riskified’s solutions assess the risks of online transactions in real time, providing businesses with deep insights based on data analytics and machine learning.
- Vena Solutions: By blending traditional risk modelling with cloud capabilities, Vena allows finance teams to work collaboratively, thereby ensuring that risks are assessed in real-time, enhancing overall organizational agility.
The beauty of these emerging technologies lies in their sheer adaptability. They are often created from the ground up with the latest tech, making them inherently flexible to deal with today's fast-paced business changes. This encourages established firms to innovate continuously, or risk being left behind.
"Innovation doesn’t come from just doing things better, it comes from doing different things."
In summary, recognizing both established leaders and emerging technologies in the risk modelling software market is crucial for businesses to navigate their options effectively. Firms must weigh the benefits of robust, proven solutions against the agility and innovation offered by newer players as they seek to fortify their risk management strategies. The interplay between these two groups creates a dynamic environment that ultimately benefits all stakeholders in the industry.
Integration and Interoperability
Integration and interoperability are pivotal concepts in the sphere of risk modelling software. They refer not only to how well a risk modelling tool can connect with existing systems and processes but also to its capability to share and utilize data effectively across platforms. In an era where information flows omnipresently between departments and systems, ensuring these aspects are taken into account during the selection process is vital for maximizing efficiency and enhancing decision-making.
Understanding integration is the first step here. A robust risk modelling software solution doesn’t only serve as a standalone application; it needs to work harmoniously with various existing hardware and software infrastructures. This consideration saves organizations the hassle of overhauling current systems and helps in maintaining continuity across operations. The more seamlessly the software integrates, the less friction there is in data transfer, which, in turn, leads to timely and informed decision-making.
Interoperability digs a bit deeper—it assesses how well different systems can communicate and understand each other’s data formats and terminologies. This is especially true for organizations relying on a mix of old and new systems, which can sometimes be as mismatched as oil and water. In such cases, implementing solutions that enable effective interoperability can be the difference between a smooth operation and a convoluted mess.
The benefits of focusing on integration and interoperability include:
- Lower Operational Costs: Reducing the need for extra resources to manage data in disparate systems reduces overall expenditures.
- Improved Accessibility: Users can access necessary data without navigating through multiple systems, saving precious time.
- Enhanced Collaboration: With data flowing freely between systems, teams can work together more fluidly, leading to better outcomes.
- Greater Flexibility: As businesses evolve, having systems that can integrate smoothly with new technologies provides a competitive edge.
Considering these factors when choosing risk modelling software cannot be overstated. A thorough understanding of how well a proposed solution will mesh with pre-existing tools ensures a smoother transition and boosts productivity.
Compatibility with Existing Systems
When evaluating risk modelling software, compatibility with existing systems should be a top priority. This aspect determines whether the software can integrate seamlessly with your current technological ecosystem, ultimately influencing the return on your investment.
For instance, imagine a company using a legacy system for financial analysis. If the newly implemented risk modelling software can’t pull in data from this legacy system or, worse, isn’t designed to output in compatible formats, this gap can lead to severe delays and errors. This doesn’t just cause frustration; it can also have financial ramifications. Therefore, ensuring that the chosen software has a track record of successful integration with similar systems can save time, money, and headaches down the line.
Moreover, organizations should prioritize solutions that are adaptable and can accommodate future technological advancements. Being able to upgrade your systems without switching out entire software platforms is not only cost-effective but also fosters an environment of continuous improvement.
APIs and Data Export Options
Application Programming Interfaces (APIs) play a crucial role in the integration narrative. They are like bridges that allow different software systems to communicate and exchange data smoothly. An effective risk modelling software should provide robust API support, allowing for the exchange of data with other applications effortlessly.
Consider this: your risk modelling software produces crucial reports, but nobody can access that data unless it’s manually entered into another application. This bottleneck is exactly the kind of issue that well-designed APIs can circumvent. When choosing risk modelling solutions, inquire about the API capabilities:
- Data Retrieval: Can the software retrieve data from existing systems easily?
- Data Sharing: How efficiently can it share findings and reports with external stakeholders or teams?
- Ease of Use: Are the APIs user-friendly, or do they require extensive technical know-how to implement?
Additionally, data export options are equally important. Teams often need to manipulate or analyze data in other systems, so being able to extract data in various formats—like CSV, JSON, or XML—gives organizations the flexibility they need.
Customization and Scalability
In the fast-paced world of risk modelling, customization and scalability are paramount. Businesses face unique challenges that require tailored approaches. Oftentimes, a one-size-fits-all solution simply doesn't cut the mustard. Risk modelling software needs to be adaptable, catering to the specific needs of different industries while also being able to grow alongside an organization. This section will discuss the nuances and importance of these two elements in effective decision-making.
Tailored Solutions for Different Industries
The importance of having customized risk modelling solutions cannot be overstated. Different industries come with their own set of risks, regulatory requirements, and operational peculiarities. For instance, the banking sector operates under strict compliance regulations and requires precise calculation of financial risks, while the healthcare industry often grapples with patient safety and compliance risks related to government regulations.
Some software solutions offer customizable dashboards, enabling users to create metrics that are relevant to their specific field. This is a game changer. The flexibility can significantly enhance the accuracy and value of insights derived from risk modelling. Moreover, it aids organizations in being proactive rather than reactive when facing challenges. Tailored solutions ensure that the metrics and models reflect the actual risk landscape of the industry in question.
A few examples of tailored solutions include:
- Financial Services: Tools that automatically incorporate market fluctuations into risk assessments.
- Healthcare: Systems that track patient outcomes and treatment risks relevant to hospitals.
- Manufacturing: Custom models assessing supply chain vulnerabilities that can impact production.
Scalability for Growth
Scalability is another critical aspect of risk modelling software. As businesses expand, their needs evolve. A system that works for a small enterprise might falter as that operation grows into a larger entity. It’s essential that risk modelling software is designed with scalability in mind to accommodate future growth.
Imagine a startup that initially used a basic risk assessment tool, one that only analyzes a handful of data points. As the business grows, the volume of data it must contend with increases dramatically. Without a scalable solution, some companies find themselves scrambling to upgrade their software or, worse, losing valuable insights due to the limitations of their systems.
Factors to consider for scalability include:
- Data Volume: Ability to handle greater amounts of data without a dip in performance.
- User Access: Capacity to support multiple users as organizations expand their teams.
- Feature Expansion: Option to integrate new features as market demands shift.
"Scalability ensures that risk modelling systems can grow with an organization, enabling continued support for decision-making as businesses expand."
In summary, customization allows for precise tailoring to specific needs while scalability ensures that risk modelling software can evolve as the organization grows. Both are indispensable in crafting an adaptive approach to risk management, ultimately boosting efficiency and understanding in a dynamic environment.
Data Security and Compliance
When it comes to risk modelling software, data security and compliance is not merely a box to check off; it plays a pivotal role in the overarching framework. Organizations are handling heaps of sensitive information—from financial records to personal data. This makes it crucial to employ software that not only safeguards this data but also adheres to various regulations. The stakes are high, and any misstep can lead to financial penalties, reputational damage, and legal consequences.
In essence, effective data security measures contribute to trust and integrity, both essential qualities in today’s competitive landscape. Businesses need to ensure that their risk modelling software complies with standards relevant to their industry while remaining adaptable to evolving compliance requirements. In this subsection, we’ll delve into the specifics of protecting sensitive information and explore the regulatory frameworks that dictate compliance.
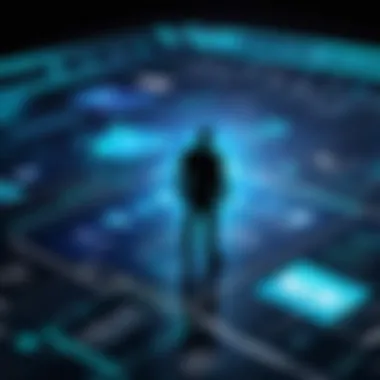
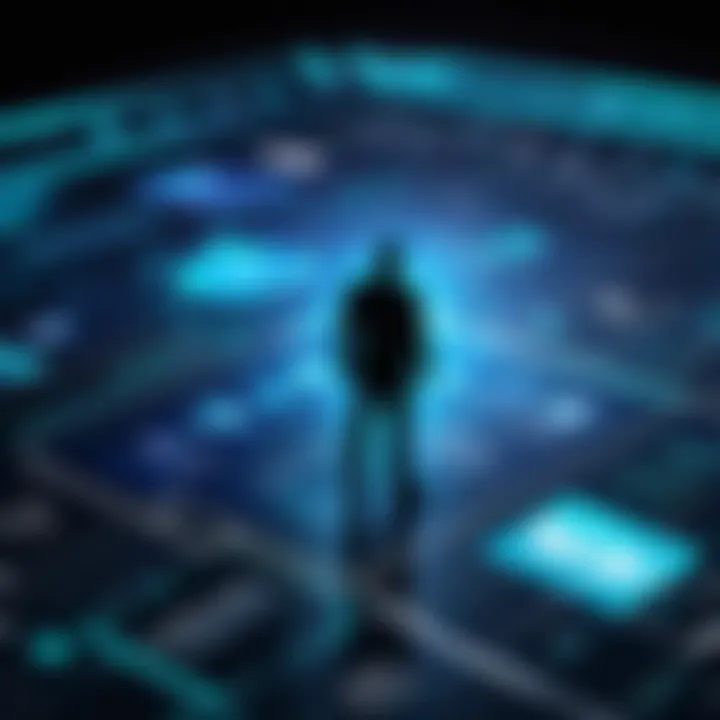
Protecting Sensitive Information
Protecting sensitive information is key to maintaining not just compliance but also operational continuity. Risk modelling software acts as a fortress, needing to shield valuable data from breaches or unauthorized access. This involves employing sophisticated encryption technologies that can safeguard data even when it’s in transit or stored in databases.
Another layer of protection often comes from role-based access controls, ensuring that only the eyes that need to see the data get to see it. Security practices should also encompass regular audits and penetration testing to uncover vulnerabilities.
Moreover, organizations should educate employees about data protection protocols. A chain is only as strong as its weakest link; therefore, an internally aware workforce enhances the entire security posture.
Some strategies for protecting sensitive information include:
- Data Encryption: Encrypting data to render it unreadable without the proper decryption keys.
- Access Controls: Implementing stringent access controls to limit who can view or manipulate sensitive data.
- Regular Audits: Conducting ongoing evaluations of data security measures to identify and mitigate risks.
"Data security isn't just a tech issue; it's a business imperative that affects every stakeholder."
Regulatory Frameworks
Regulatory frameworks often serve as the bedrock for compliance in risk modelling software. These frameworks—varying by geography and industry—determine how data must be handled, stored, and processed. For instance, the General Data Protection Regulation (GDPR) in the European Union establishes strict guidelines on data usage and provides individuals with greater control over their personal information. Similarly, regulations like the Health Insurance Portability and Accountability Act (HIPAA) govern how healthcare organizations should handle sensitive patient information in the United States.
Failing to adhere to these frameworks isn’t simply about losing customer trust; it could lead to hefty fines that can cripple even the most promising startups. As such, building software that is both compliant and secure from day one is essential. Organizations should regularly consult legal experts to stay up-to-date with changing regulations.
Furthermore, risk modelling software providers often take great care to ensure their products meet various requirements, which can streamline compliance for end-users.
Important regulatory considerations include:
- GDPR: Ensuring compliance with European data laws, protecting individuals’ privacy.
- HIPAA: Addressing data security in healthcare settings.
- PCI DSS: Following payment card industry standards for processing credit card information.
In summary, data security and compliance is not just about ticking off requirements; it's about establishing a stronghold that protects sensitive information while navigating the complex landscape of regulatory expectations. It’s this dual focus that empowers organizations to harness risk modelling software effectively while minimizing risks.
Case Studies: Successful Implementation
Successful implementation of risk modelling software reveals pivotal insights into how the theoretical benefits translate into real-world applications. The case studies help in illustrating the specific challenges faced by various industries and how these tools aid in navigating uncertainties. By examining these examples, organizations can draw valuable lessons that inform their own risk management strategies.
Industry-Specific Examples
Different sectors exhibit distinct risk profiles and management methods. Here are a few notable instances of how risk modelling software was successfully applied:
- Financial Services: A banking institution implemented a risk modelling tool to enhance its credit risk assessment framework. By integrating predictive analytics, they identified potential defaults earlier, which led to a 20% reduction in non-performing loans over three years.
- Healthcare Sector: A large hospital system adopted risk modelling software to manage patient outcomes. The tool analyzed historical data to predict potential complications in surgical patients. This not only improved patient safety but also cut readmission rates by 15%.
- Manufacturing: In the automotive industry, a manufacturer leveraged risk modelling to predict supply chain disruptions. By simulating various scenarios, they were able to mitigate risks coming from suppliers in volatile regions, ensuring a steady production flow even during crises.
These real-world cases highlight how tailored applications of risk modelling software can create substantial differences in operational efficiency and decision-making processes across industries.
Lessons Learned
Implementing risk modelling software is not without its hurdles. Here are some key lessons distilled from various case studies:
- Data Quality is Paramount: Good data leads to better decision-making. Companies that invested time in cleansing and organizing data found more accurate predictive insights, thus enhancing their modelling reliability.
- User Training is Essential: Employees must feel confident in using these tools. Organizations that provided thorough training and continuous support experienced higher adoption rates and greater satisfaction.
- Iterative Processes Bring Greater Improvements: Risk modelling should not be a one-time endeavor. Companies that regularly revised and updated their models based on emerging data and trends noticed an increase in accuracy and relevance.
- Collaboration Across Departments: Engaging different departments during implementation can enrich the data pool. Risk assessments benefit from diverse perspectives, thus leading to more comprehensive risk profiles.
By taking these lessons into account, organizations can set themselves up for a more successful experience with risk modelling software adoption, ultimately leading to robust risk management capabilities.
"Understanding not just the software but the context in which you operate is the key to unlocking its full potential."
Successful case studies demonstrate that a thoughtful approach in implementing risk modelling software can significantly sharpen an organization's ability to respond to risks and uncertainties.
Challenges in Risk Modelling
Navigating the landscape of risk modelling software isn't always a walk in the park. Many organizations find themselves grappling with various challenges that can affect the effectiveness of their risk management strategies. As industries become increasingly digital, these challenges can present serious obstacles. Understanding these difficulties is crucial for anyone looking to implement risk modelling effectively. This section examines two primary challenges: the quality and availability of data, and the complexity of models.
Data Quality and Availability
One of the most pressing issues in risk modelling is the quality of data. Data serves as the bedrock upon which models are constructed. Poor quality data can lead to inaccurate results, which in turn can steer decisions down the wrong path. For instance, if a financial institution relies on outdated or incomplete information, it might underestimate the potential risks involved in lending operations. This could result in financial losses or worse, systemic issues within the economy.
Availability of high-quality data is also essential. Organizations often work in silos, leading to gaps in data sharing and input. This fragmented approach hampers the ability to gain a holistic view of risk. In some cases, necessary data might simply not exist, stymying any comprehensive analysis.
To mitigate these issues, companies can invest in data governance frameworks that define standards for data quality and a centralized system for data access. Additionally, regular audits may help identify inconsistencies and areas needing improvement.
"Good quality data is the oil that runs the engine of modern risk modelling."
Complexity of Models
The increasing sophistication of risk models has its own set of hurdles. While complex models can offer deeper insights, they often come with higher maintenance costs and an increased chance of misinterpretation. For example, the use of intricate algorithms woven into predictive models can obscure decision-making pathways. When team members need to navigate these complex structures, misunderstandings can easily arise, leading to erroneous outcomes.
Moreover, regulatory agencies often mandate specific risk metrics that need to be calculated. Meeting these regulatory requirements can add layers of complexity to already challenging models. New frameworks for compliance can further clutter the analytical process, turning what could be a straightforward analysis into an intricate puzzle.
To counteract the intricacies that such models entail, organizations should foster a culture of understanding among their stakeholders. Providing training sessions on how to interpret these models effectively can bridge the gap between complex mechanics and business decision-making.
Future Trends in Risk Modelling Software
As we move forward, the realm of risk modelling software is witnessing transformative changes driven by technological advancements. Understanding these trends is essential for organizations aiming to stay ahead in an increasingly complex business environment. This section elaborates on the integration of artificial intelligence and machine learning, as well as the advancements in predictive analytics, both of which play pivotal roles in reshaping how risk is assessed and managed.
Integration of AI and Machine Learning
The infusion of AI and machine learning into risk modelling software is more than just a passing fad; it represents a seismic shift in how data is processed and analyzed. These technologies can enhance the accuracy of risk predictions by identifying patterns that would be impossible for human analysts to discern alone. For instance, machine learning algorithms can analyze vast datasets, refining risk models over time through continual learning from new data enters.
Moreover, machine learning can tailor risk assessments to individual organizations, adapting recommendations based on unique operational contexts. Some software solutions now include features that allow real-time adjustment of risk models as new variables are recognized. Such integration not only improves efficiency but also boosts the confidence of decision-makers faced with complex risk landscapes.
"With AI, organizations are not just reacting to risks; they are anticipating them, significantly reducing potential impacts on their operations."
The consideration of ethical implications is also necessary when implementing AI. Ensuring transparency in algorithmic decisions is crucial for maintaining trust among stakeholders.
Advancements in Predictive Analytics
Predictive analytics is steadily carving out its niche in risk modelling, providing organizations with tools to forecast potential risks more accurately. By analyzing historical data and current trends, predictive analytics software can generate insights that inform strategic planning and risk mitigation efforts.
The benefits of such advancements are manifold:
- Improved Risk Awareness: Organizations can spot emerging risks that may otherwise go unnoticed.
- Enhanced Decision-Making: Data-driven insights allow for more informed choices regarding resource allocation and strategic initiatives.
- Operational Efficiency: Automation of data analysis frees up valuable resources, enabling focus on higher-level strategic tasks.
For example, a financial institution might use predictive analytics to evaluate market volatility, thereby refining their investment strategies and improving capital management. This depth of analysis paves the way for more resilient operational frameworks.
In closing, the integration of AI and machine learning alongside the advancements in predictive analytics signifies a significant evolution in risk modelling software. These technologies not only enhance the risk assessment processes but also facilitate a proactive approach toward risk management. As businesses continue to adapt to these advancements, they will find themselves better equipped to navigate the uncertainties inherent in today’s market landscape.
Assessment and Comparison of Software Solutions
Assessing and comparing risk modelling software solutions is a cornerstone consideration for organizations aiming to enhance their risk management strategies. In a landscape flooded with various offerings, the need for a structured evaluation is paramount to ensure that the chosen software aligns with both business objectives and regulatory requirements. This process is not merely about picking a tool, it’s about selecting a partner that can aid in navigating the complexities of risk management.
The advantages of thorough assessment are manifold. Primarily, it allows decision-makers to identify features that will effectively address their specific risk exposure. Furthermore, understanding pricing structures enables businesses to select not just effective, but also economically viable solutions. Considerations about user-friendliness and the support provided by software vendors are crucial as well, affecting both initial satisfaction and long-term utilization.
A well-executed comparison not only highlights the strengths of potential software solutions but also uncovers weaknesses that may not be apparent at first glance. This exploration can prevent costly missteps that might arise from poor software choices. Identifying compatibility with existing systems can also ease the transition, reducing downtime and resource allocation associated with implementation.
"A decision from an informed position can save an organization not only money but also enhance its overall risk posture."
Evaluation Criteria
When delving into the evaluation criteria for risk modelling software, a few key aspects come to the forefront. These criteria provide a framework for dissecting the myriad options available, ensuring a focused approach to assessment:
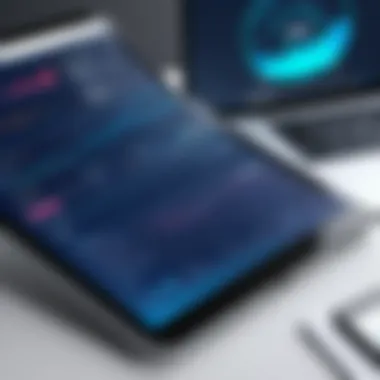
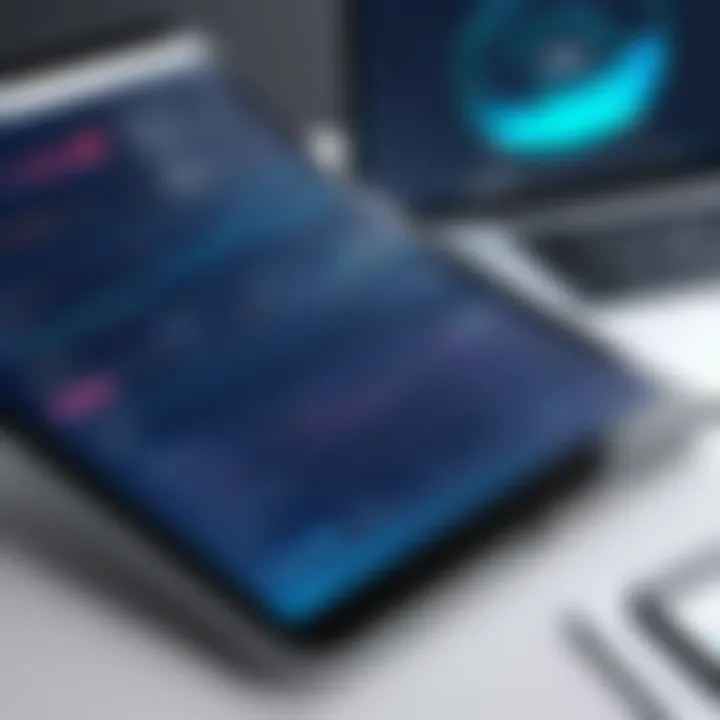
- Functionality: The core capabilities of the software must align with the organization's risk modelling needs. This includes assessing whether it can handle quantitative analyses, qualitative assessments, or both.
- Ease of Use: With complex models, the solution should be designed for accessibility, minimizing the training curve for users.
- Integration: Compatibility with current systems can save substantial time and resources. It's crucial for the software to integrate seamlessly with data streams and existing platforms.
- Vendor Support: Robust customer support is essential. A good vendor should offer extensive resources for troubleshooting and user guidance.
- Scalability: As organizations grow, their risk management needs evolve. Thus, the chosen software must scale as required.
Taking the time to compare each of these criteria across selected platforms will significantly aid in making a judicious choice.
Features vs. Pricing
The relationship between features and pricing of risk modelling software is often a delicate balancing act. While one might assume that higher cost equates to better features, this isn't always the case. Understanding this relationship requires a critical look into what is truly necessary versus what is merely a shiny add-on.
- Basic vs. Advanced Features: Determine the essential features necessary for your operations. Sometimes, basic analysis tools might suffice, while other situations may demand advanced predictive analytics.
- Cost Structures: Various pricing models can include subscription-based fees, one-time purchases, or even tiered models that charge based on usage. Each has its own implications on budgeting and financial forecasting.
- Hidden Costs: Be conscious of potential hidden fees related to onboarding, ongoing training, or even data management. These can lead to vastly inflated total costs over time.
- Value Proposition: Assess how well the features of the software justify its cost. This involves looking at ROI, potential for streamlining operations, and long-term value, rather than just upfront costs.
It’s imperative to align software capabilities with budgetary constraints while ensuring that the selected tool reliably meets the organization’s risk modelling needs. By focusing on both features and pricing, businesses can adopt a more strategic approach in selecting risk modelling software.
User Training and Support
Effective user training and robust support are cornerstones of successfully implementing risk modelling software within any organization. When employees understand how to leverage these tools, they can make informed decisions that enhance operational efficiency and minimize uncertainties. Training is not just about familiarizing users with software features; it is about embedding a risk-aware culture within the organization. When teams feel confident in their capabilities, the potential for optimizing risk management practices dramatically increases. Organizations must consider tailored training programs that cater to different levels of expertise among users to ensure that all participants glean the maximum benefit.
Onboarding Processes
The onboarding process sets the stage for successful software adoption. It's essential to structure this part to address the unique needs of the company and its employees. This includes a dedicated onboarding timeline, outlining when and how each team member should engage with the system.
- Initial Setup: Start by configuring software settings that align with the company's specific risk parameters. This helps in creating a personalized experience from the get-go.
- Training Modules: Develop interactive training sessions that use real-life scenarios relevant to the organization's environment. This approach demystifies complex concepts and encourages active engagement.
- Hands-On Practice: Allow users to familiarize themselves with the software through practical exercises. This hands-on experience can lead to a much better understanding than just talking through features.
"Training isn't just an event - it's a continual journey of skill enhancement and engagement."
Organizations also should collect feedback post-training to refine future sessions. Understanding user experiences helps improve onboarding processes for new employees and may surface previously overlooked challenges.
Ongoing Support Options
Ongoing support is crucial to maintaining user engagement with the software as needs evolve. Providing a safety net encourages users to experiment without fear of failure. Here are several effective strategies:
- Helpdesk Availability: Setting up a helpdesk can provide immediate assistance for any technical issues that users may encounter, ensuring minimal disruptions.
- User Community: Creating forums or groups enables users to share insights, tricks, and solutions. This becomes a living document of best practices that all can benefit from.
- Regular Updates: Regularly updating training materials to reflect new features or changes in the system ensures users are always informed and can utilize the software to its fullest potential.
- Advanced Training Sessions: Periodic advanced training can help seasoned users deepen their understanding and tackle more complex risk scenarios.
Encouraging open lines of communication for users to express their concerns or suggestions about the software can reinforce the importance of user experience in refining support structures. Effective ongoing support not only stabilizes usage but can also improve the organization's overall risk management strategies.
Industry Regulations Affecting Risk Modelling
Understanding the role of industry regulations in risk modelling software is essential for any organization serious about managing potential hazards. The landscape of risk is shaped not only by internal factors but also by external expectations and legal requirements. When companies operate within regulated industries, they often find themselves navigating a maze of compliance mandates. Not adhering to these guidelines can lead to significant financial penalties and reputational damage, not to mention the consequences of failing to protect stakeholders appropriately.
Key considerations regarding industry regulations include:
- Ensuring compliance with local, national, and international laws.
- Understanding how regulations evolve with the advancements in technology and data usage.
- Knowing the penalties for non-compliance, which can be both monetary and legal.
The integration of regulations into risk modelling practices also serves as a guiding star for decision-makers. These regulations provide a framework for creating models that do not just forecast risk but do so within acceptable limits set by governing authorities. By implementing software that takes these regulations into account, businesses can not only limit their liability but also enhance their credibility in the marketplace.
Key Regulations to Consider
When discussing regulations, there are several pivotal ones that resonate across various sectors:
- GDPR (General Data Protection Regulation): Governing how organizations collect and process personal data, especially relevant for businesses operating in the European Union. The importance of GDPR in risk modelling cannot be overstated as it mandates strict data privacy measures.
- SOX (Sarbanes-Oxley Act): This is vital for publicly traded companies in the United States. SOX aims to protect shareholders from fraudulent financial reporting, making compliance imperative for accurate risk assessments.
- Basel III: Targeting banks and financial institutions, Basel III establishes risk management standards, addressing capital adequacy, stress testing, and market liquidity risks. Adopting models that adhere to these standards is crucial for financial entities.
- HIPAA (Health Insurance Portability and Accountability Act): For entities managing health information, HIPAA introduces privacy standards for the safeguarding of Protected Health Information (PHI).
- ISO 31000: While not regulation per se, ISO 31000 provides guidelines for risk management that organizations can reference to bolster their internal compliance measures.
"In a world where risks are omnipresent, adhering to regulations is not just about legality; it’s about ethical responsibility."
Choosing risk modelling software that aligns with these regulations means opting for solutions that support risk management processes with compliance at their core. By doing this, businesses can transform regulatory obligations from a burden into a valuable tool for strategic growth. The complexity of regulations can at times seem like trying to read a map in the dark, but with the right tools and understanding, organizations can navigate this terrain effectively.
Best Practices for Implementation
Implementing risk modelling software isn't just about selecting a package and flipping the switch. It’s a meticulous process that must be executed with precision to unlock its full potential. Best practices for implementation serve as a roadmap, guiding organizations toward success in navigating the often murky waters of risk management. Getting it right can greatly enhance the effectiveness of decision-making processes, resource allocation, and overall risk mitigation strategies.
Strategic Planning
Before diving headfirst into software adoption, thoughtful strategic planning is paramount. This phase involves assessing the organization's current situation, identifying specific needs, and setting clear objectives.
- Define Goals: What do you want to achieve with the risk modelling software? Are you looking to improve decision-making or comply with regulatory standards? Articulating your goals can streamline the implementation process.
- Assess Requirements: Each organization is unique, with distinct operational demands. It’s essential to evaluate the existing frameworks, workflows, and IT infrastructure. This analysis helps in choosing software that aligns seamlessly with your environment.
- Set a Timeline: Establishing a realistic implementation timeline is vital. A rushed rollout often results in oversight, issues, and insufficient training. Creating milestones to track progress ensures accountability throughout the execution process.
- Allocate Resources: Strategic planning also includes budgeting for the implementation. This budget may encompass software costs, training sessions for staff, and ongoing maintenance needs. Failing to allocate adequate resources can lead to unexpected delays and complications.
Ultimately, strategic planning mitigates uncertainty and lays a solid foundation for a successful risk modelling software implementation.
User Involvement
Involving users throughout the implementation process is another critical best practice. Their firsthand experience and insights can drive better outcomes, ensuring the software is tailored to meet actual needs. Here are key points to consider:
- Early Engagement: Get user feedback from the onset. They know the ins and outs of the daily operations, and understanding their challenges can help tailor the software’s setup.
- Training Sessions: Invest in comprehensive training programs for all users. Fully understanding how to leverage the new tools can make or break your implementation’s effectiveness.
- Continuous Feedback Loop: Establish a mechanism for continuous feedback during and after implementation. Regular check-ins can identify issues early and create opportunities for iterative improvements.
- Change Management: Encourage users to embrace the new system. Addressing psychological resistance and framing changes positively can ease the transition, fostering an environment where users feel comfortable adapting to new workflows.
Implementing best practices in strategic planning and user involvement not only smoothens the process but also aligns the software capabilities with real-world application. The end result is a more resilient, informed, and prepared organization, equipped to tackle the multifaceted challenges of risk management.
“Involve users in the process; their insights can drive success in ways you might not see from the top down.”
By adhering to these best practices, organizations can ensure they are on the right path when implementing risk modelling software, ultimately paving the way for more effective risk management strategies.
Measuring the ROI of Risk Modelling Software
Understanding the return on investment (ROI) when it comes to risk modelling software is crucial for organizations looking to safeguard their future while also optimizing their resources. ROI measures the value gained from an investment relative to its cost. This aspect is particularly pertinent in today's fast-paced business environment, where companies must justify expenses in a sea of options and potential outcomes.
A thoughtful ROI analysis helps businesses draw clear connections between software utilization and tangible benefits such as risk reduction, enhanced compliance, and better decision-making capabilities. Investing in robust risk modelling software can seem like dipping into the deep end of the pool at first—daunting and uncertain. However, clarity emerges through systematic evaluation of costs versus benefits, which ultimately aligns with organizational priorities and goals.
"Every business decision carries risk, but how can you gauge whether investing in risk modelling software is worth it? The answer lies in ROI measurement."
Cost-Benefit Analysis
Conducting a cost-benefit analysis is the first step to determining ROI in risk modelling software. This process entails identifying and quantifying all feasible costs associated with the software, along with the anticipated benefits it delivers. These costs could include initial purchase price, implementation fees, ongoing maintenance expenses, and training costs.
On the flip side, the benefits can span various dimensions:
- Reduced Loss: By pinpointing potential risks ahead of time, companies can avert financial losses.
- Time Efficiency: Automation features can free up employee time, making resources available for more strategic tasks.
- Better Compliance Rates: Software can help in aligning processes with industry regulations, preventing penalties.
In making the calculations, it’s vital to avoid an apples-to-apples comparison. For example, the cost of skipping risk modelling tools often might exceed the investment in these systems, due to unforeseen consequences. Diverse industries will see varied impacts, requiring custom calculations hinged on specific business needs.
Performance Metrics
Once the costs and benefits have been meticulously mapped out, assessing the actual performance of the risk modelling software becomes next up on the to-do list. Here, performance metrics serve as essential yardsticks for gauging effectiveness.
These metrics can give insights into how well the software meets expectations. Some key performance indicators to consider include:
- Accuracy of Risk Predictions: Is the software consistently identifying risks and their potential impacts accurately?
- User Adoption Rates: The extent to which employees use it regularly could highlight its usability and integration into everyday tasks.
- Reduction in Incident Costs: An evident decrease in costs associated with risk incidents can signify successful implementation.
Establishing clear performance targets and continually monitoring these metrics gives firms a solid foundation to assess the ongoing ROI of their risk modelling software. By adopting a data-driven approach, organizations can make informed decisions about the necessity of further investments or optimizations.
Culmination
In wrapping up our exploration of risk modelling software, it’s evident that this area represents a cornerstone for modern business strategies. The tools available today empower organizations to effectively navigate uncertainties inherent in various domains. Understanding these software solutions is not just about features and functions; it’s about harnessing potential benefits that can drive success and sustainability in unpredictable markets.
Recap of Key Insights
Throughout the article, we have uncovered the multifaceted nature of risk modelling software. To distill these insights:
- Decision-Making Enhancement: Risk modelling software enables data-driven choices that are crucial in high-stakes environments. Decision-makers are armed with tools that process vast amounts of information efficiently, leading to more informed conclusions.
- Integration and Compatibility: In an era where digital ecosystems are becoming increasingly complex, risk modelling solutions that offer robust integration with existing systems are essential. APIs and diverse data export options can make or break a software's effectiveness in a business.
- Customizable Solutions: Businesses today are not one-size-fits-all. The ability to tailor solutions to specific industry needs enhances relevance and operational efficiency. This leads to increased user adoption and maximizes return on investment.
- Future Trends: The incorporation of advanced technologies like AI and machine learning is essential. These innovations promise enhanced predictive accuracy and better risk assessment capabilities. Keeping an eye on these trends ensures organizations do not lag in their risk management strategies.
Future Directions
As we look ahead, it’s clear that risk modelling software will continue to evolve. Businesses will need to adapt promptly to these changes to stay competitive. Future directions to watch for include:
- Artificial Intelligence: Look for AI-driven analytics that not only predict potential risks but also recommend mitigative actions. It’s about transforming data into insights at lightning speed.
- Cloud-based Solutions: Migration to cloud technology will encourage collaboration and improve accessibility. Remote work trends necessitate software that can operate seamlessly across digital platforms.
- Sustainability Initiatives: Companies are increasingly being measured on their sustainability efforts. Future risk modelling will likely integrate environmental risk assessments, ensuring that businesses account for their ecological footprint.